Characterizing neural activity in cognitively inspired RL agents during an evidence accumulation task.
IJCNN(2023)
摘要
Evidence accumulation is thought to be fundamental for decision-making in humans and other mammals. It has been extensively studied in neuroscience and cognitive science with the goal of explaining how sensory information is sequentially sampled until sufficient evidence has accumulated to favor one decision over others. Neuroscience studies suggest that the hippocampus encodes a low-dimensional ordered representation of evidence through sequential neural activity. Cognitive modelers have proposed a mechanism by which such sequential activity could emerge through the modulation of recurrent weights with a change in the amount of evidence. This gives rise to neurons tuned to a specific magnitude of evidence which resemble neurons recorded in the hippocampus. Here we integrated a cognitive science model inside a Reinforcement Learning (RL) agent and trained the agent to perform a simple evidence accumulation task inspired by the behavioral experiments on animals. We compared the agent's performance with the performance of agents equipped with GRUs and RNNs. We found that the agent based on a cognitive model was able to learn faster and generalize better while having significantly fewer parameters. We also compared the emergent neural activity across agents and found that in some cases, GRU-based agents developed similar neural representations to agents based on a cognitive model. This study illustrates how integrating cognitive models and artificial neural networks can lead to brain-like neural representations that can improve learning.
更多查看译文
关键词
Evidence accumulation,Reinforcement learning,Accumulating towers task,Counting
AI 理解论文
溯源树
样例
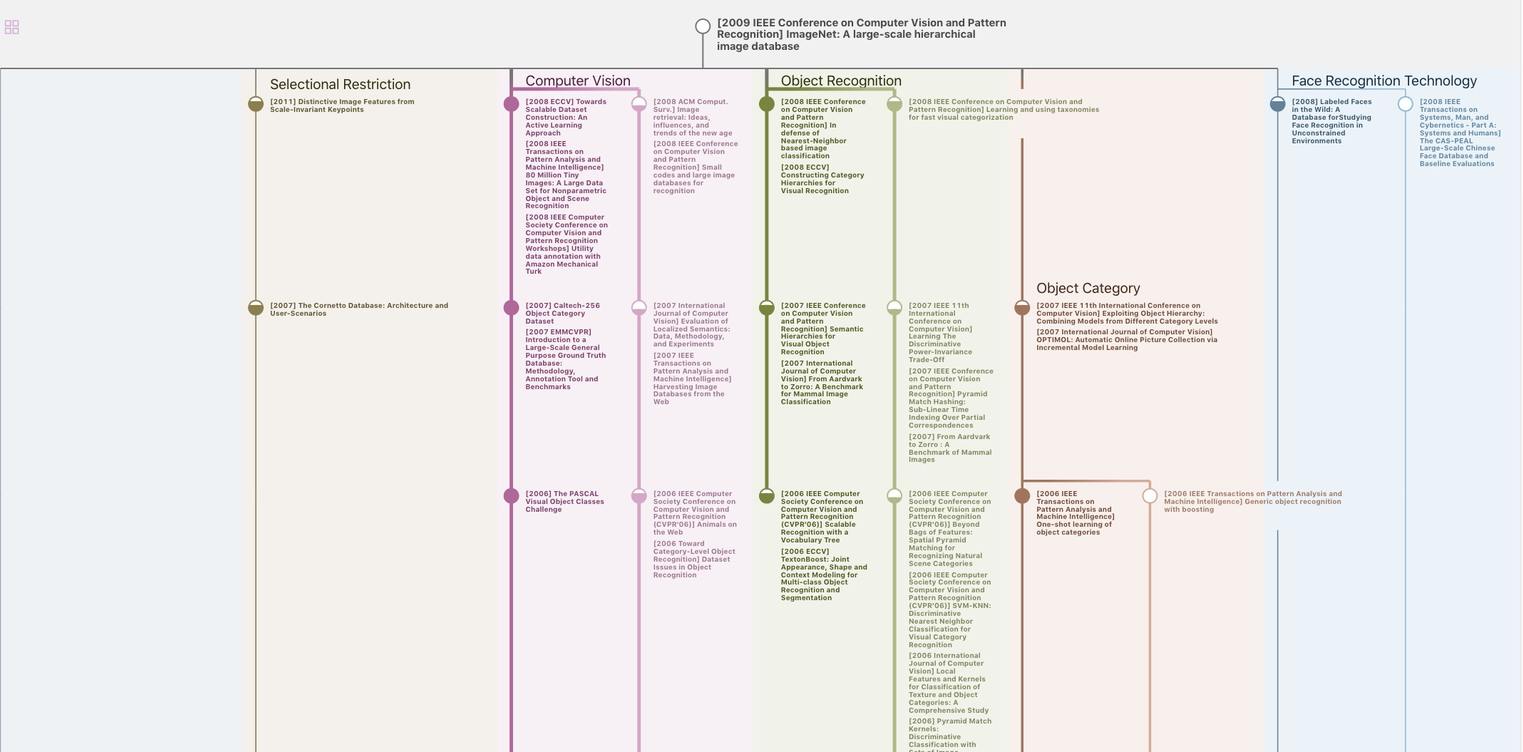
生成溯源树,研究论文发展脉络
Chat Paper
正在生成论文摘要