SMKD: Selective Mutual Knowledge Distillation.
IJCNN(2023)
摘要
Mutual knowledge distillation (MKD) is a technique used to transfer knowledge between multiple models in a collaborative manner. However, it is important to note that not all knowledge is accurate or reliable, particularly under challenging conditions such as label noise, which can lead to models that memorize undesired information. This problem can be addressed by improving the reliability of the knowledge source, as well as selectively selecting reliable knowledge for distillation. While making a model more reliable is a widely studied topic, selective MKD has received less attention. To address this, we propose a new framework called selective mutual knowledge distillation (SMKD). The key component of SMKD is a generic knowledge selection formulation, which allows for either static or progressive selection thresholds. Additionally, SMKD covers two special cases: using no knowledge and using all knowledge, resulting in a unified MKD framework. We present extensive experimental results to demonstrate the effectiveness of SMKD and justify its design.
更多查看译文
关键词
generic knowledge selection formulation,knowledge source reliability,progressive selection thresholds,selective mutual knowledge distillation,SMKD,static selection thresholds,unified MKD framework
AI 理解论文
溯源树
样例
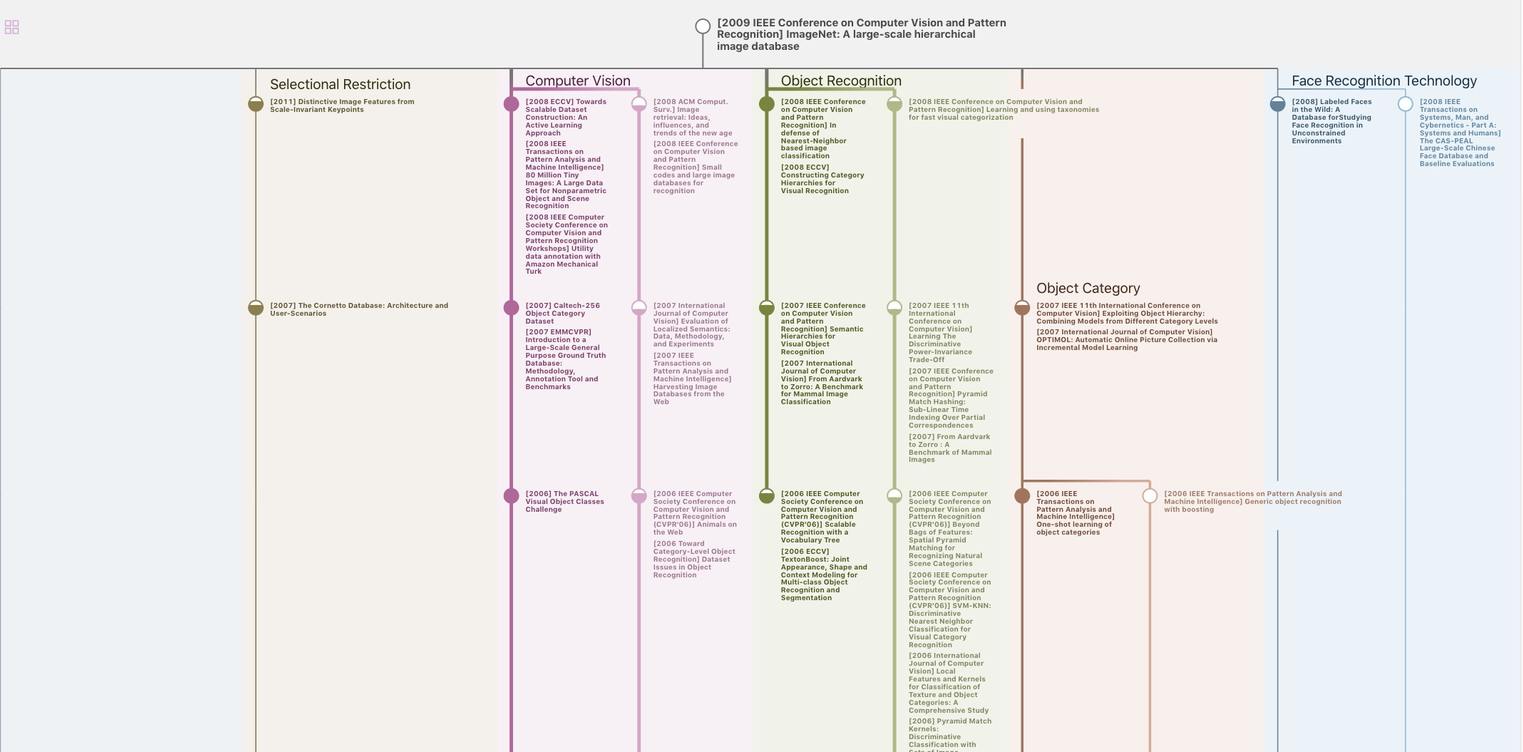
生成溯源树,研究论文发展脉络
Chat Paper
正在生成论文摘要