Participants recruitment for coverage maximization by mobility predicting in mobile crowd sensing
China Communications(2023)
Abstract
Mobile Crowd Sensing (MCS) is an emerging paradigm that leverages sensor-equipped smart devices to collect data. The introduction of MCS also poses some challenges such as providing high-quality data for upper layer MCS applications, which requires adequate participants. However, recruiting enough participants to provide the sensing data for free is hard for the MCS platform under a limited budget, which may lead to a low coverage ratio of sensing area. This paper proposes a novel method to choose participants uniformly distributed in a specific sensing area based on the mobility patterns of mobile users. The method consists of two steps: (1) A second-order Markov chain is used to predict the next positions of users, and select users whose next places are in the target sensing area to form a candidate pool. (2) The Average Entropy (DAE) is proposed to measure the distribution of participants. The participant maximizing the DAE value of a specific sensing area with different granular sub-areas is chosen to maximize the coverage ratio of the sensing area. Experimental results show that the proposed method can maximize the coverage ratio of a sensing area under different partition granularities.
MoreTranslated text
Key words
data average entropy,human mobility prediction,markov chain,mobile crowd sensing
AI Read Science
Must-Reading Tree
Example
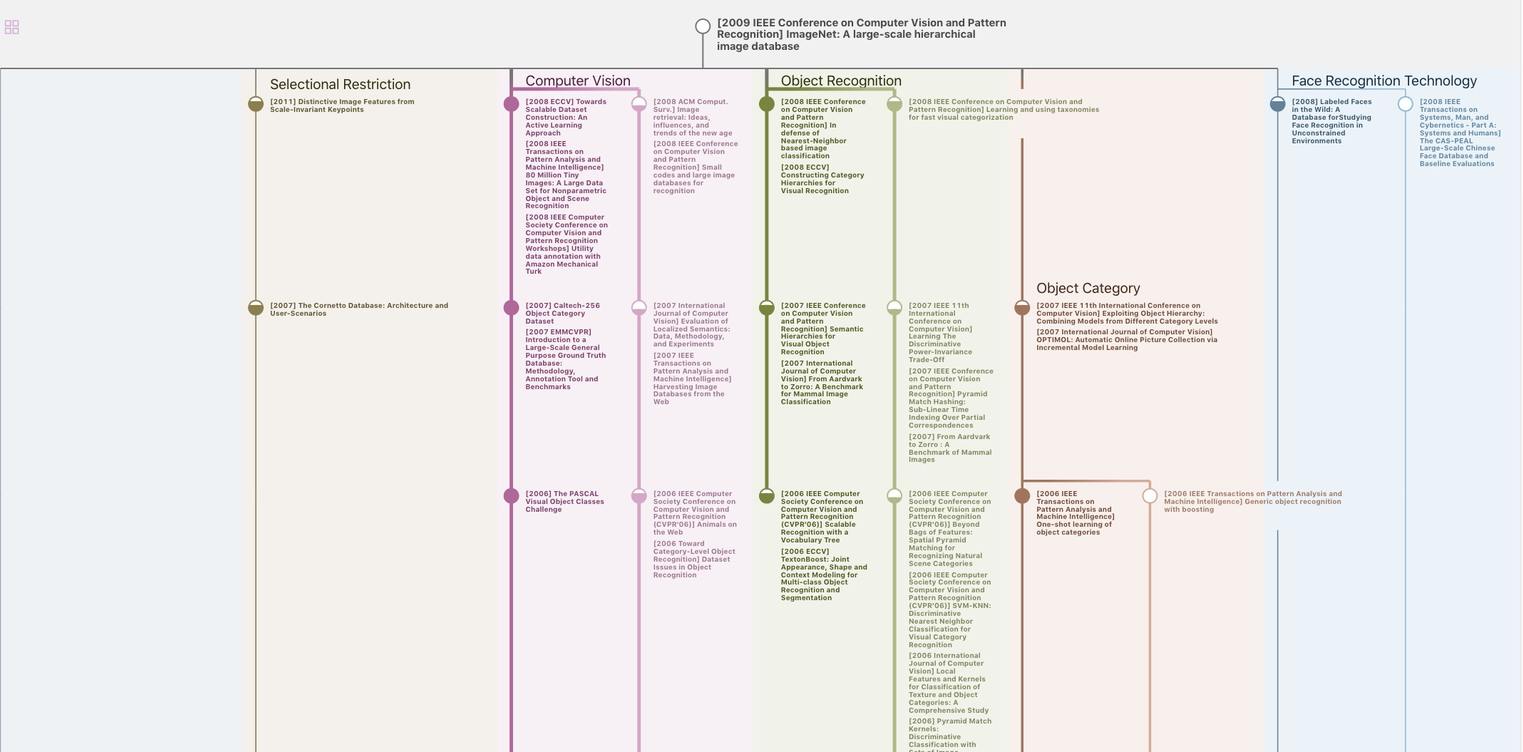
Generate MRT to find the research sequence of this paper
Chat Paper
Summary is being generated by the instructions you defined