Improving the accuracy of automated gout flare ascertainment using natural language processing of electronic health records and linked Medicare claims data
PHARMACOEPIDEMIOLOGY AND DRUG SAFETY(2024)
Abstract
Background: We aimed to determine whether integrating concepts from the notes from the electronic health record (EHR) data using natural language processing (NLP) could improve the identification of gout flares. Methods: Using Medicare claims linked with EHR, we selected gout patients who initiated the urate-lowering therapy (ULT). Patients' 12-month baseline period and on treatment follow-up were segmented into 1-month units. We retrieved EHR notes for months with gout diagnosis codes and processed notes for NLP concepts. We selected a random sample of 500 patients and reviewed each of their notes for the presence of a physician-documented gout flare. Months containing at least 1 note mentioning gout flares were considered months with events. We used 60% of patients to train predictive models with LASSO. We evaluated the models by the area under the curve (AUC) in the validation data and examined positive/negative predictive values (P/NPV). Results: We extracted and labeled 839 months of follow-up (280 with gout flares). The claims-only model selected 20 variables (AUC = 0.69). The NLP concept-only model selected 15 (AUC = 0.69). The combined model selected 32 claims variables and 13 NLP concepts (AUC = 0.73). The claims-only model had a PPV of 0.64 [0.50, 0.77] and an NPV of 0.71 [0.65, 0.76], whereas the combined model had a PPV of 0.76 [0.61, 0.88] and an NPV of 0.71 [0.65, 0.76]. Conclusion: Adding NLP concept variables to claims variables resulted in a small improvement in the identification of gout flares. Our data-driven claims-only model and our combined claims/NLP-concept model outperformed existing rule-based claims algorithms reliant on medication use, diagnosis, and procedure codes.
MoreTranslated text
Key words
gout,natural language processing
AI Read Science
Must-Reading Tree
Example
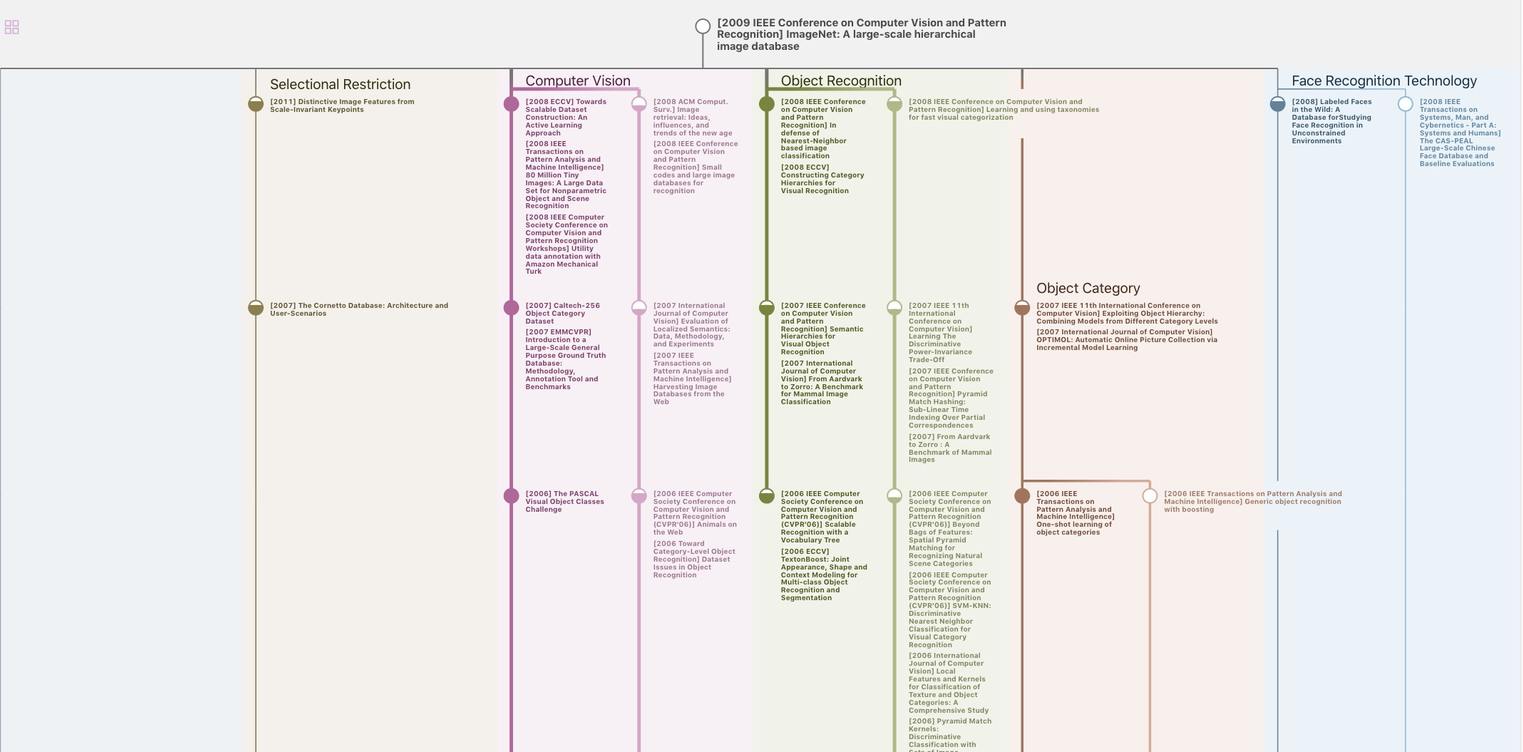
Generate MRT to find the research sequence of this paper
Chat Paper
Summary is being generated by the instructions you defined