OvaRePred: Online tool for predicting the age of fertility milestones.
Innovation (Cambridge (Mass.))(2023)
摘要
Ovarian reserve is the quantity of primordial follicles present in the ovarian cortex that have the potential to mature into healthy oocytes. A high ovarian reserve typically results in a high chance of a successful pregnancy for couples. Fertility declines as women age because of changes in the ovarian reserve, and this decline is called ovarian aging. Diminished ovarian reserve (DOR) can impact fertility regardless of chronological age and can lead to embryonic aneuploidy, fertilization failure, and high pregnancy loss rates. Therefore, it is important to consider ovarian reserve when evaluating the potential effect of aging on fertility, rather than relying solely on chronological age. Many women are unaware of the heterogeneity in ovarian reserve and ovarian aging. Whereas irregular cycles and menopause are easily identifiable, these signs often indicate extremely low fertility, leaving limited available interventions. Well-established methods for evaluating ovarian reserve include measurement of follicle-stimulating hormone (FSH) and anti-Mullerian hormone (AMH) levels, assessment of antral follicle counts (AFC) by ultrasound, and evaluation of ovarian response to controlled ovarian stimulation. These methods are typically complemented by considering other factors such as age and overall health. However, it is important to remember that these assessments are not definitive, and their interpretation may vary among clinicians, potentially leading to different results. Previously, we developed a set of highly standardized algorithms for assessing ovarian reserve, namely the AA (AMH and Age), AFA (AMH, FSH, and age), and AAFA (AMH, AFC, FSH, and age) models.1Han Y. Xu H. Feng G. et al.An online tool for predicting ovarian reserve based on AMH level and age: A retrospective cohort study.Front. Endocrinol. 2022; 13946123Crossref Scopus (2) Google Scholar,2Xu H. Shi L. Feng G. et al.An Ovarian Reserve Assessment Model Based on Anti-Mullerian Hormone Levels, Follicle-Stimulating Hormone Levels, and Age: Retrospective Cohort Study.J. Med. Internet Res. 2020; 22e19096Crossref Scopus (15) Google Scholar,3Xu H. Feng G. Wang H. et al.A novel mathematical model of true ovarian reserve assessment based on predicted probability of poor ovarian response: a retrospective cohort study.J. Assist. Reprod. Genet. 2020; 37: 963-972Crossref PubMed Scopus (11) Google Scholar In the AAFA model, for example, poor ovarian response (POR) of <5 oocyte retrieved was used as the outcome variable, and AMH levels, evaluation of AFC by ultrasound, FSH, and age were used as the predictors. By default, the AAFA model categorizes a probability >50% as a predicted POR. We assigned an ovarian reserve score to individuals based on their predicted probability of POR, also referred to as DOR. The ovarian reserve score is calculated as 100 × (1 − POR probability), where a high POR probability corresponds to a low ovarian reserve score. The subjects were categorized into four groups based on the predicted POR probability: A (>90 points), B (>75–90 points), C (>50–75 points), and D (≤50 points). The objective was to develop a comprehensive tool for not only assessing the current ovarian reserve of individuals but also for predicting important fertility milestones such as DOR or perimenopause. For this, we added an ovarian aging curve to the previous models that were used to assess the current ovarian reserve. This comprehensive tool has significant potential as a crucial marker for women’s reproductive health, providing insights into the timing and management of significant events such as menopause. The information provided by this tool will be invaluable for guiding clinical decision-making, including optimizing the timing of fertility treatments, hormone replacement therapy, and menopause management. Moreover, the ovarian aging curve has profound implications for fertility preservation and family planning, particularly for women undergoing cancer treatments. We believe that this comprehensive tool will enable a more precise and personalized assessment of ovarian function, surpassing the traditional methods of ovarian reserve assessment that heavily rely on the expertise of clinicians. Ovarian aging is believed to follow a growth curve model.4te Velde E.R. Pearson P.L. The variability of female reproductive ageing.Hum. Reprod. Update. 2002; 8: 141-154Crossref PubMed Scopus (906) Google Scholar Furthermore, the fixed interval hypothesis proposes that the time spans between different reproductive milestones, such as subfertility, sterility, irregularity, and menopause, are fixed in the general population (as shown in Figure 1A).4te Velde E.R. Pearson P.L. The variability of female reproductive ageing.Hum. Reprod. Update. 2002; 8: 141-154Crossref PubMed Scopus (906) Google Scholar Building on this hypothesis, our objective was to establish a growth curve that illustrates ovarian aging using the cross-sectional data of 21,219 cycles from 2017 to 2018 obtained from Peking University Third Hospital.5Xu H. Feng G. Alpadi K. et al.A Model for Predicting Polycystic Ovary Syndrome Using Serum AMH, Menstrual Cycle Length, Body Mass Index and Serum Androstenedione in Chinese Reproductive Aged Population: A Retrospective Cohort Study.Front. Endocrinol. 2022; 13821368Google Scholar All cycles were included in the analysis with no exclusions. By combining information about an individual’s current ovarian reserve status with the ovarian aging curve, the time required to reach a specific ovarian reserve status can be calculated. To establish the ovarian aging curve, we used various growth curve models to investigate the relationship between the proportion of DOR and age. Ultimately, we selected a logistic growth curve model with a coefficient of determination (r2) of 0.978, indicating that it can explain 97.8% of the relationship between DOR proportion and age. In this study, DOR was defined based on the AAFA model.1Han Y. Xu H. Feng G. et al.An online tool for predicting ovarian reserve based on AMH level and age: A retrospective cohort study.Front. Endocrinol. 2022; 13946123Crossref Scopus (2) Google Scholar The growth curve depicting the relationship between DOR proportion and age is shown in Figure 1B. Notably, this curve has similar characteristics to the growth curve model of the fixed interval hypothesis constructed a century ago, which depicted changes of fertility milestones over age in a cohort that did not use birth control methods (Figure 1A). Diseases or conditions that affect ovarian function can impact the ovarian aging curve. Therefore, we generated specific ovarian aging curves for different diseases or conditions, including endometriosis, ovulatory disorders, uterine factors, fallopian tube factors, and body mass index (BMI). Infertility factors were categorized into two groups (present or absent), and BMI was classified into three groups with cutoff values of 24 kg/m2 and 28 kg/m2; however, none of the infertility factors or BMI categories had a statistically significant effect on the growth curves. The growth curves for ovulatory disorders (p value of 0.189) and uterine factor infertility (p value of 0.989) are shown in Figures 1C and 1D, respectively. The lack of statistical differences in the ovarian aging curves among different diseases or BMI categories may be attributed to the lack of data about the severity of the diseases and their comorbidities. To address this limitation, it is crucial to conduct follow-up studies using standardized data that document the severity of different diseases and their associated comorbidities. We selected the growth curve in Figure 1B, which represents the entire population, as the final ovarian aging curve for our prediction model. In our study, the population with DOR corresponds to the predicted population with POR. The DOR ratio presented in the ovarian aging curve (Figure 1B) can be considered as the probability of DOR and, consequently, the probability of POR. This ratio also corresponds to the ovarian reserve score in the AA, AFA, and AAFA models used to assess ovarian reserve.1Han Y. Xu H. Feng G. et al.An online tool for predicting ovarian reserve based on AMH level and age: A retrospective cohort study.Front. Endocrinol. 2022; 13946123Crossref Scopus (2) Google Scholar,2Xu H. Shi L. Feng G. et al.An Ovarian Reserve Assessment Model Based on Anti-Mullerian Hormone Levels, Follicle-Stimulating Hormone Levels, and Age: Retrospective Cohort Study.J. Med. Internet Res. 2020; 22e19096Crossref Scopus (15) Google Scholar,3Xu H. Feng G. Wang H. et al.A novel mathematical model of true ovarian reserve assessment based on predicted probability of poor ovarian response: a retrospective cohort study.J. Assist. Reprod. Genet. 2020; 37: 963-972Crossref PubMed Scopus (11) Google Scholar For instance, by using the current ovarian reserve scores and the ovarian aging curve, the time interval between the current ovarian reserve score and a future ovarian reserve score can be predicted. Thus, the comprehensive tool can be used to determine the predicted time interval for DOR, indicated by a DOR proportion of 50%, as well as for perimenopause, indicated by a DOR proportion of 94.0%, which represents the lowest ovarian reserve score in the data assessed by the AAFA model. With knowledge of the current age and the time interval, the age at which a future fertility milestone is likely to occur can easily be determined. The absence of a postmenopausal population in our dataset meant that the comprehensive tool could only predict perimenopause, which aligns with the population in our reproductive center that had the poorest ovarian reserve. DOR typically occurs approximately 10 years before menopause, and our ovarian aging curve reflects a similar pattern with DOR corresponding approximately to the sterility population represented in Figure 1A. We developed the comprehensive tool into an online tool called OvaRePred for evaluating the current ovarian reserve status as well as predicting the future age at which DOR and perimenopause may occur. OvaRePred can be accessed at http://121.43.113.123:9999/. Finally, individuals with diseases or conditions that could potentially affect AMH levels are advised to exercise caution when using OvaRePred or to avoid using it altogether because they can lead to inaccurate ovarian reserve scores for certain individuals. Factors that can contribute to variations in AMH levels include irregular menstrual cycles, diseases that impact ovarian function, smoking, exposure to environmental pollutants, and/or use of oral contraceptives. Specifically, when AMH levels fluctuate in the range of 3 ng/mL, the algorithms of our ovarian reserve models may generate significant fluctuations in the ovarian reserve score for an individual. OvaRePred will allow reproductive-aged women to make their own childbirth plan according to their own ovarian reserve status and may help them manage other diseases by taking their current and future ovarian reserve status into consideration.
更多查看译文
关键词
fertility milestones,online tool,age
AI 理解论文
溯源树
样例
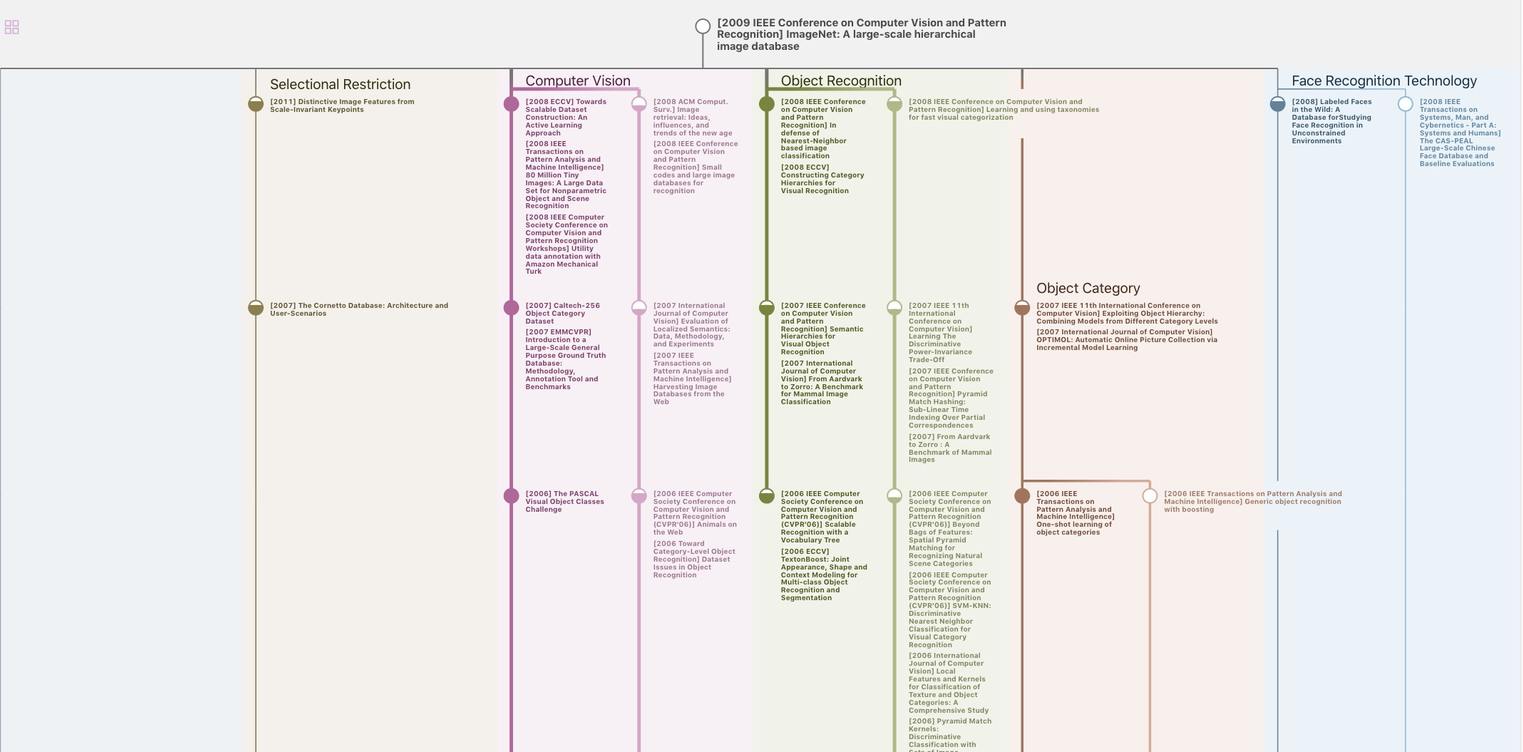
生成溯源树,研究论文发展脉络
Chat Paper
正在生成论文摘要