TEA-S: A Tiny and Efficient Architecture for PLAC-Based Softmax in Transformers
IEEE Transactions on Circuits and Systems II: Express Briefs(2023)
摘要
With the popularity of Transformer neural networks, it is inevitable for hardware accelerators to perform nonlinear computation mainly based on the softmax operation. However, a better compromise between the algorithm performance and hardware overhead is always a constant challenge. Hence, this brief advances a tiny and efficient architecture named TEA-S to implement the softmax function with the universal approximation scheme based on Piecewise Linear Approximation Computation (PLAC). With the first co-optimization of calculation and memory, TEA-S can better achieve the design goals of the tiny area and high efficiency. The implementation results show that the peak efficiency of processing 8-bit quantized data will be 487.51 Gps/(mm
$^{{2}}{\cdot }$
mW) with the tiny area of
$3052.43~{\mu }{\mathrm {m}}^{2}$
at the frequency of 0.5 GHz under 90-nm CMOS technology. Moreover, TEA-S can offer the universal solution to any lengths of input sequences, providing negligible accuracy loss in Transformers compared to the quantized baselines.
更多查看译文
关键词
softmax,transformers,plac-based
AI 理解论文
溯源树
样例
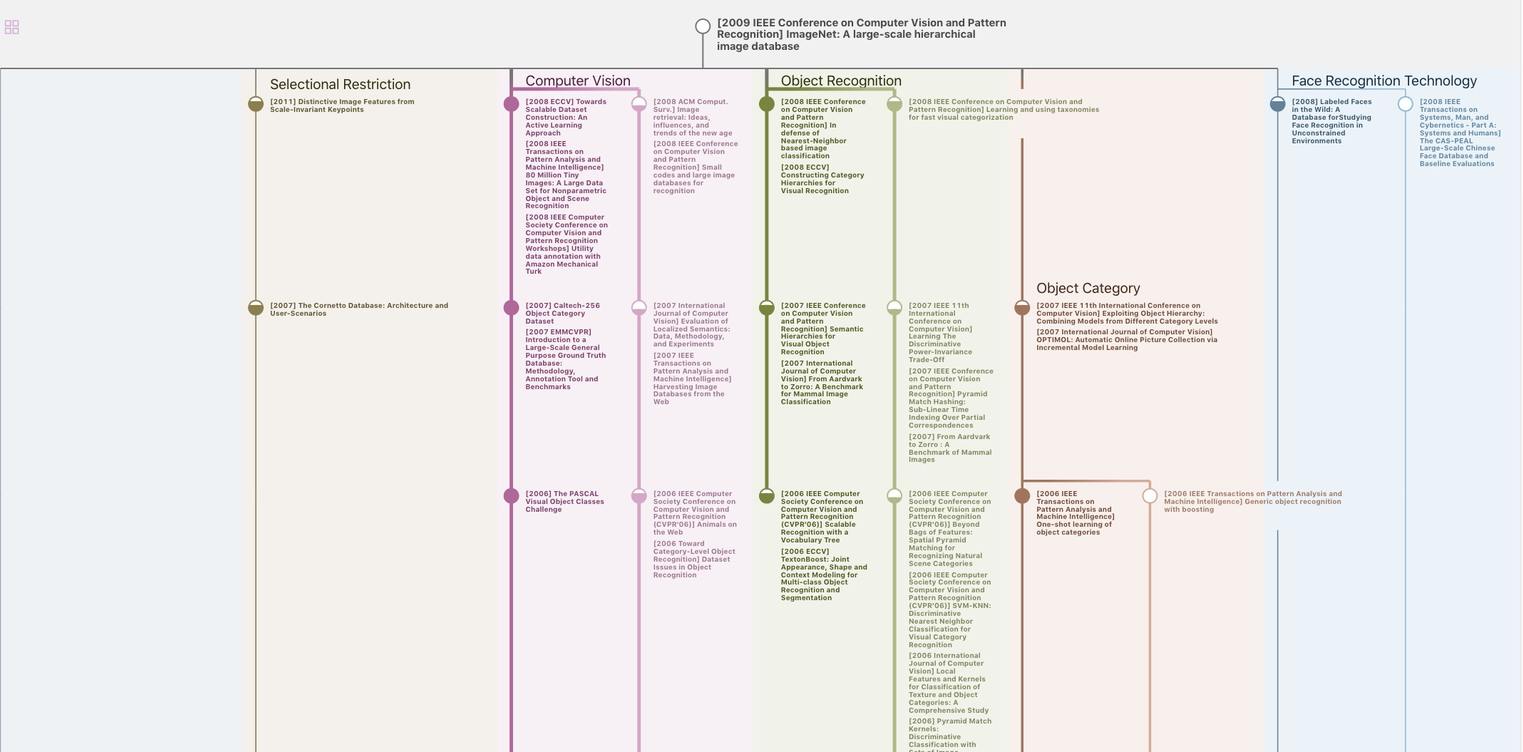
生成溯源树,研究论文发展脉络
Chat Paper
正在生成论文摘要