Multiscale Dynamic Feature Learning for Quality Prediction Based on Hierarchical Sequential Generative Network
IEEE Sensors Journal(2023)
摘要
In industrial processes, long short-term memory (LSTM) is usually used for temporal dynamic modeling of soft sensor. The process data usually have various temporal correlations under different time scales due to the continuous physical and chemical reactions. However, LSTM model can only extract the dynamic features at a specific time scale, which affects the feature learning capability and modeling accuracy. In this article, a new hierarchical sequential generative network (HSGN) is proposed for mining multiscale dynamic features using large amount of unlabeled process data for soft sensor. To extract multiscale dynamic features for quality prediction, the process data are resampled with different sampling rates and then used to pretrain the corresponding self-learning LSTM models at different time scales. Subsequently, they can used to calculate the multiscale hidden feature states for labeled samples, which are further integrated with the original input information and input into a deep belief network (DBN) to construct the prediction model for the output variable. Thus, the HSGN method can take advantage of large number of unlabeled samples to mine multiscale dynamic hidden features and overcome the irregular sampling problem in industrial processes. The application in a real industrial scene shows the effectiveness of the proposed method.
更多查看译文
关键词
Deep learning, hierarchical sequential generative network (HSGN), multiscale dynamics, quality prediction, soft sensor
AI 理解论文
溯源树
样例
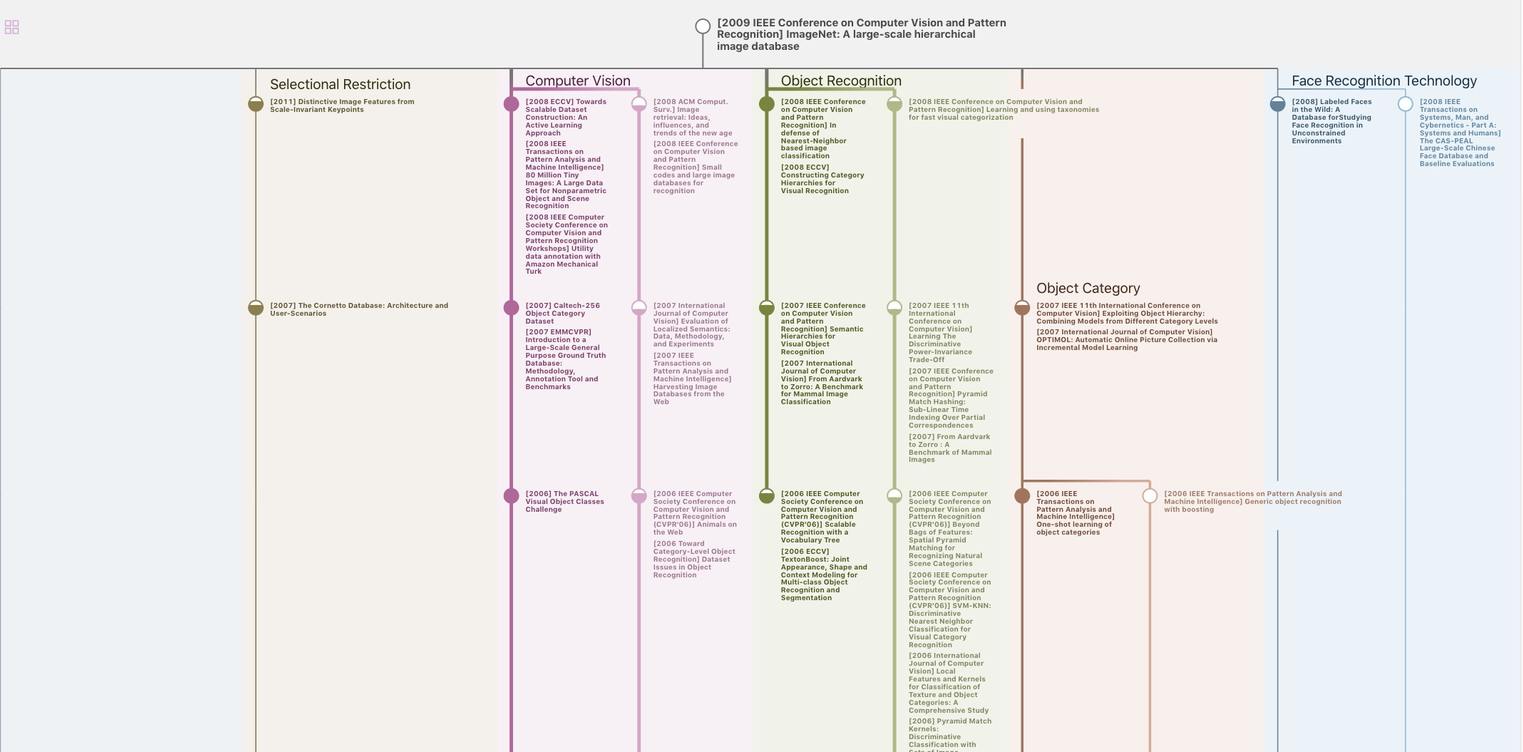
生成溯源树,研究论文发展脉络
Chat Paper
正在生成论文摘要