An Approach for EEG Denoising Based on Wasserstein Generative Adversarial Network.
IEEE transactions on neural systems and rehabilitation engineering : a publication of the IEEE Engineering in Medicine and Biology Society(2023)
摘要
Electroencephalogram (EEG) recordings often contain artifacts that would lower signal quality. Many efforts have been made to eliminate or at least minimize the artifacts, and most of them rely on visual inspection and manual operations, which is time/labor-consuming, subjective, and incompatible to filter massive EEG data in real-time. In this paper, we proposed a deep learning framework named Artifact Removal Wasserstein Generative Adversarial Network (AR-WGAN), where the well-trained model can decompose input EEG, detect and delete artifacts, and then reconstruct denoised signals within a short time. The proposed approach was systematically compared with commonly used denoising methods including Denoised AutoEncoder, Wiener Filter, and Empirical Mode Decomposition, with both public and self-collected datasets. The experimental results proved the promising performance of AR-WGAN on automatic artifact removal for massive data across subjects, with correlation coefficient up to 0.726±0.033, and temporal and spatial relative root-mean-square error as low as 0.176±0.046 and 0.761±0.046, respectively. This work may demonstrate the proposed AR-WGAN as a high-performance end-to-end method for EEG denoising, with many on-line applications in clinical EEG monitoring and brain-computer interfaces.
更多查看译文
关键词
Electroencephalogram,generative adversarial network,denoising,artifacts removal,BCI
AI 理解论文
溯源树
样例
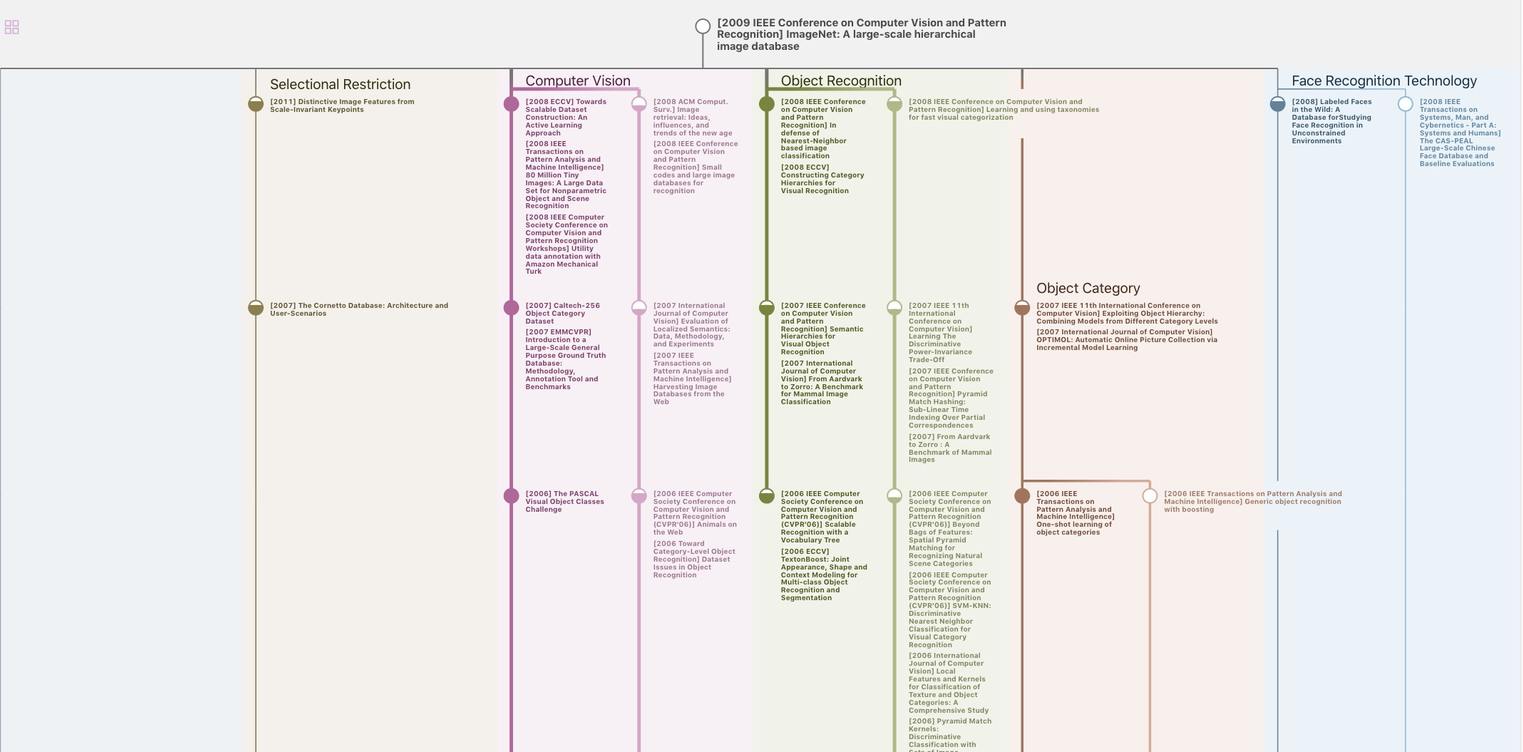
生成溯源树,研究论文发展脉络
Chat Paper
正在生成论文摘要