A Neural-Enhanced Factor Graph-Based Algorithm for Robust Positioning in Obstructed LOS Situations
IEEE OPEN JOURNAL OF SIGNAL PROCESSING(2024)
摘要
This paper presents a neural-enhanced probabilistic model and corresponding factor graph-based sum-product algorithm for robust localization and tracking in multipath-prone environments. The introduced hybrid probabilistic model consists of physics-based and data-driven measurement models capturing the information contained in both, the line-of-sight (LOS) component as well as in multipath components (NLOS components). The physics-based and data-driven models are embedded in a joint Bayesian framework allowing to derive from first principles a factor graph-based algorithm that fuses the information of these models. The proposed algorithm uses radio signal measurements from multiple base stations to robustly estimate the mobile agent's position together with all model parameters. It provides high localization accuracy by exploiting the position-related information of the LOS component via the physics-based model and robustness by exploiting the geometric imprint of multipath components independent of the propagation channel via the data-driven model. In a challenging numerical experiment involving obstructed LOS situations to all anchors, we show that the proposed sequential algorithm significantly outperforms state-of-the-art methods and attains the posterior Cramer-Rao lower bound even with training data limited to local regions.
更多查看译文
关键词
Signal processing algorithms,Data models,Feature extraction,Location awareness,Probabilistic logic,Bayes methods,Position measurement,Obstructed line-of-sight,non los,nlos,multipath,sum product algorithm,probabilistic data association,belief propagation
AI 理解论文
溯源树
样例
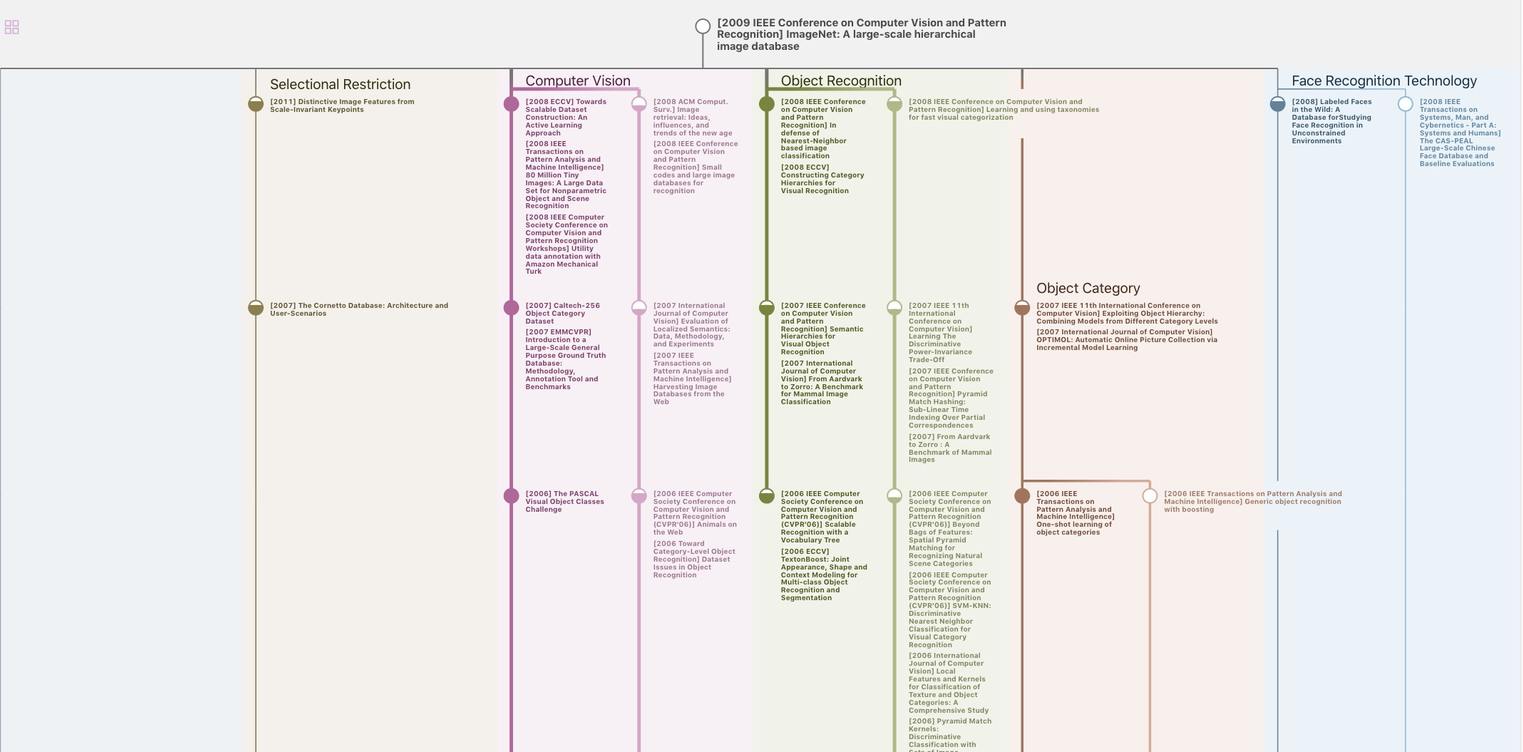
生成溯源树,研究论文发展脉络
Chat Paper
正在生成论文摘要