Denoising Attention for Query-aware User Modeling in Personalized Search
CoRR(2023)
摘要
The personalization of search results has gained increasing attention in the past few years, thanks to the development of Neural Networks-based approaches for Information Retrieval and the importance of personalization in many search scenarios. Recent works have proposed to build user models at query time by leveraging the Attention mechanism, which allows weighing the contribution of the user-related information w.r.t. the current query. This approach allows taking into account the diversity of the user's interests by giving more importance to those related to the current search performed by the user. In this paper, we first discuss some shortcomings of the standard Attention formulation when employed for personalization. In particular, we focus on issues related to its normalization mechanism and its inability to entirely filter out noisy user-related information. Then, we introduce the Denoising Attention mechanism: an Attention variant that directly tackles the above shortcomings by adopting a robust normalization scheme and introducing a filtering mechanism. The reported experimental evaluation shows the benefits of the proposed approach over other Attention-based variants.
更多查看译文
关键词
attention,search,query-aware
AI 理解论文
溯源树
样例
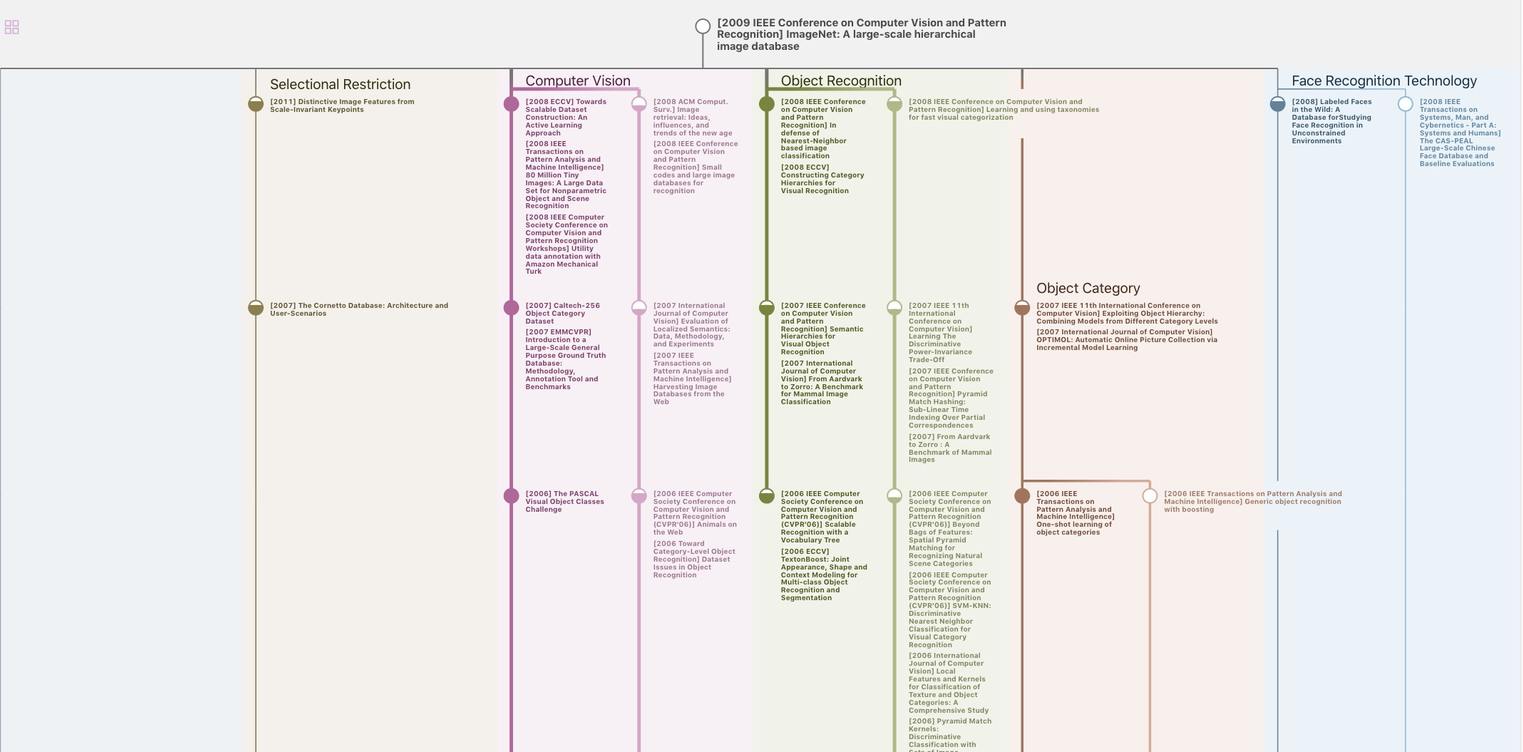
生成溯源树,研究论文发展脉络
Chat Paper
正在生成论文摘要