Computational Lower Bounds for Graphon Estimation via Low-degree Polynomials
CoRR(2023)
摘要
Graphon estimation has been one of the most fundamental problems in network analysis and has received considerable attention in the past decade. From the statistical perspective, the minimax error rate of graphon estimation has been established by Gao et al (2015) for both stochastic block model (SBM) and nonparametric graphon estimation. The statistical optimal estimators are based on constrained least squares and have computational complexity exponential in the dimension. From the computational perspective, the best-known polynomial-time estimator is based on universal singular value thresholding (USVT), but it can only achieve a much slower estimation error rate than the minimax one. It is natural to wonder if such a gap is essential. The computational optimality of the USVT or the existence of a computational barrier in graphon estimation has been a long-standing open problem. In this work, we take the first step towards it and provide rigorous evidence for the computational barrier in graphon estimation via low-degree polynomials. Specifically, in both SBM and nonparametric graphon estimation, we show that for low-degree polynomial estimators, their estimation error rates cannot be significantly better than that of the USVT under a wide range of parameter regimes. Our results are proved based on the recent development of low-degree polynomials by Schramm and Wein (2022), while we overcome a few key challenges in applying it to the general graphon estimation problem. By leveraging our main results, we also provide a computational lower bound on the clustering error for community detection in SBM with a growing number of communities and this yields a new piece of evidence for the conjectured Kesten-Stigum threshold for efficient community recovery.
更多查看译文
关键词
graphon estimation,computational,polynomials,low-degree
AI 理解论文
溯源树
样例
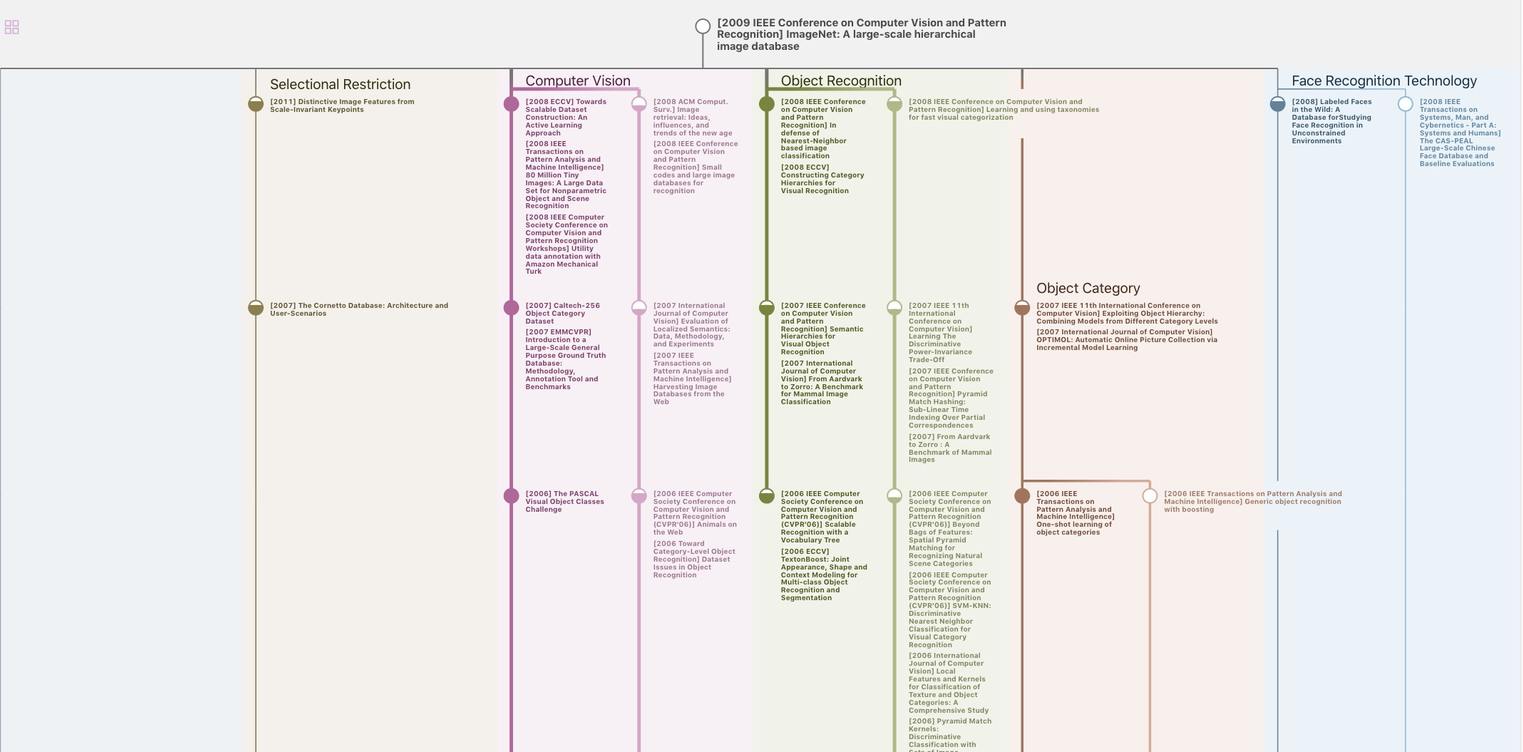
生成溯源树,研究论文发展脉络
Chat Paper
正在生成论文摘要