Detect, Augment, Compose, and Adapt: Four Steps for Unsupervised Domain Adaptation in Object Detection
CoRR(2023)
摘要
Unsupervised domain adaptation (UDA) plays a crucial role in object detection when adapting a source-trained detector to a target domain without annotated data. In this paper, we propose a novel and effective four-step UDA approach that leverages self-supervision and trains source and target data concurrently. We harness self-supervised learning to mitigate the lack of ground truth in the target domain. Our method consists of the following steps: (1) identify the region with the highest-confidence set of detections in each target image, which serve as our pseudo-labels; (2) crop the identified region and generate a collection of its augmented versions; (3) combine these latter into a composite image; (4) adapt the network to the target domain using the composed image. Through extensive experiments under cross-camera, cross-weather, and synthetic-to-real scenarios, our approach achieves state-of-the-art performance, improving upon the nearest competitor by more than 2% in terms of mean Average Precision (mAP). The code is available at https://github.com/MohamedTEV/DACA.
更多查看译文
关键词
adaptation,object detection,unsupervised domain,augment
AI 理解论文
溯源树
样例
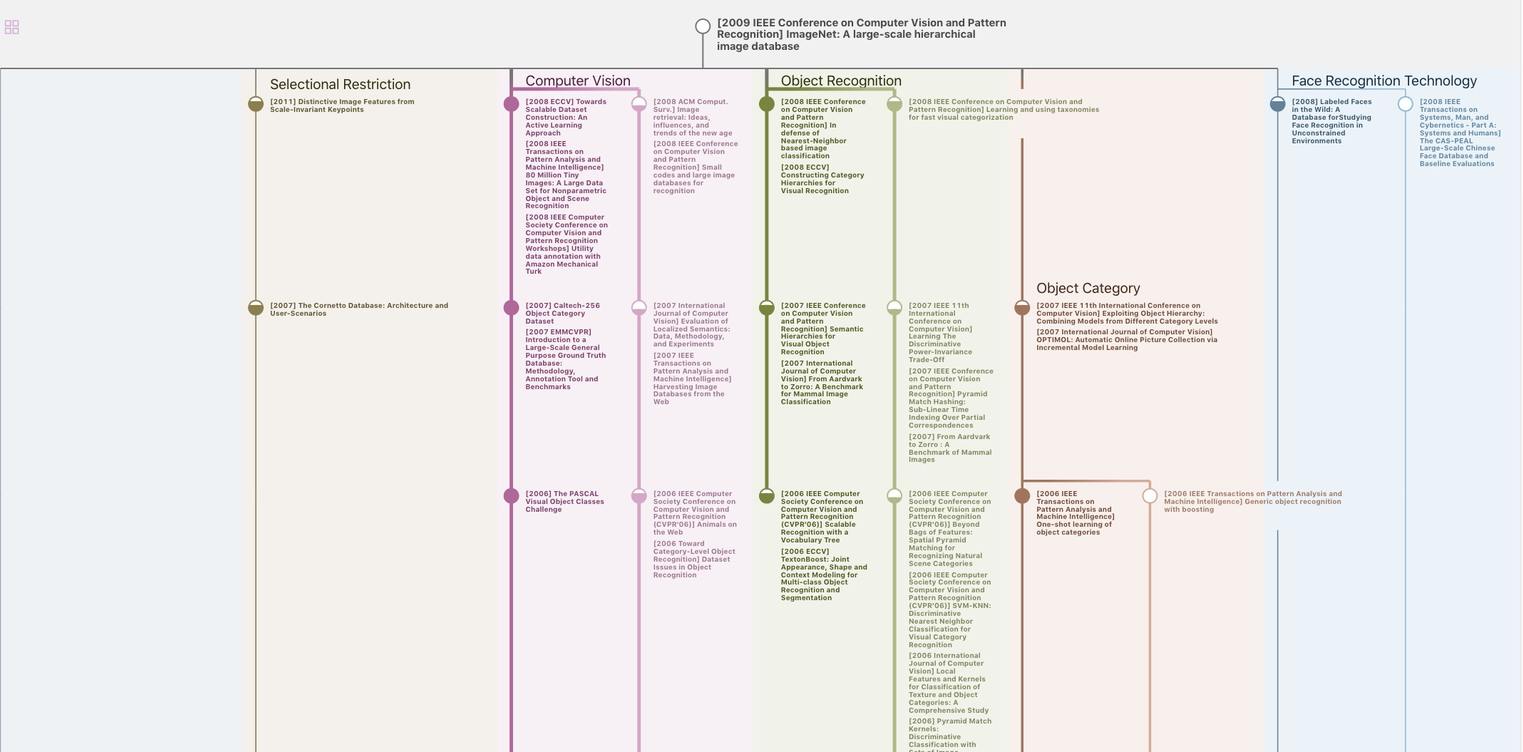
生成溯源树,研究论文发展脉络
Chat Paper
正在生成论文摘要