Biquality learning: a framework to design algorithms dealing with closed-set distribution shifts
MACHINE LEARNING(2023)
摘要
Training machine learning models from data with weak supervision and dataset shifts is still challenging. Designing algorithms when these two situations arise has not been explored much, and existing algorithms cannot always handle the most complex distributional shifts. We think the biquality data setup is a suitable framework for designing such algorithms. Biquality Learning assumes that two datasets are available at training time: a trusted dataset sampled from the distribution of interest and the untrusted dataset with dataset shifts and weaknesses of supervision (aka distribution shifts). The trusted and untrusted datasets available at training time make designing algorithms dealing with any distribution shifts possible. We propose two methods, one inspired by the label noise literature and another by the covariate shift literature for biquality learning. We experiment with two novel methods to synthetically introduce concept drift and class-conditional shifts in real-world datasets across many of them. We opened some discussions and assessed that developing biquality learning algorithms robust to distributional changes remains an interesting problem for future research.
更多查看译文
关键词
Biquality learning,Distribution shift,Weakly supervised learning,Dataset shift,Machine learning
AI 理解论文
溯源树
样例
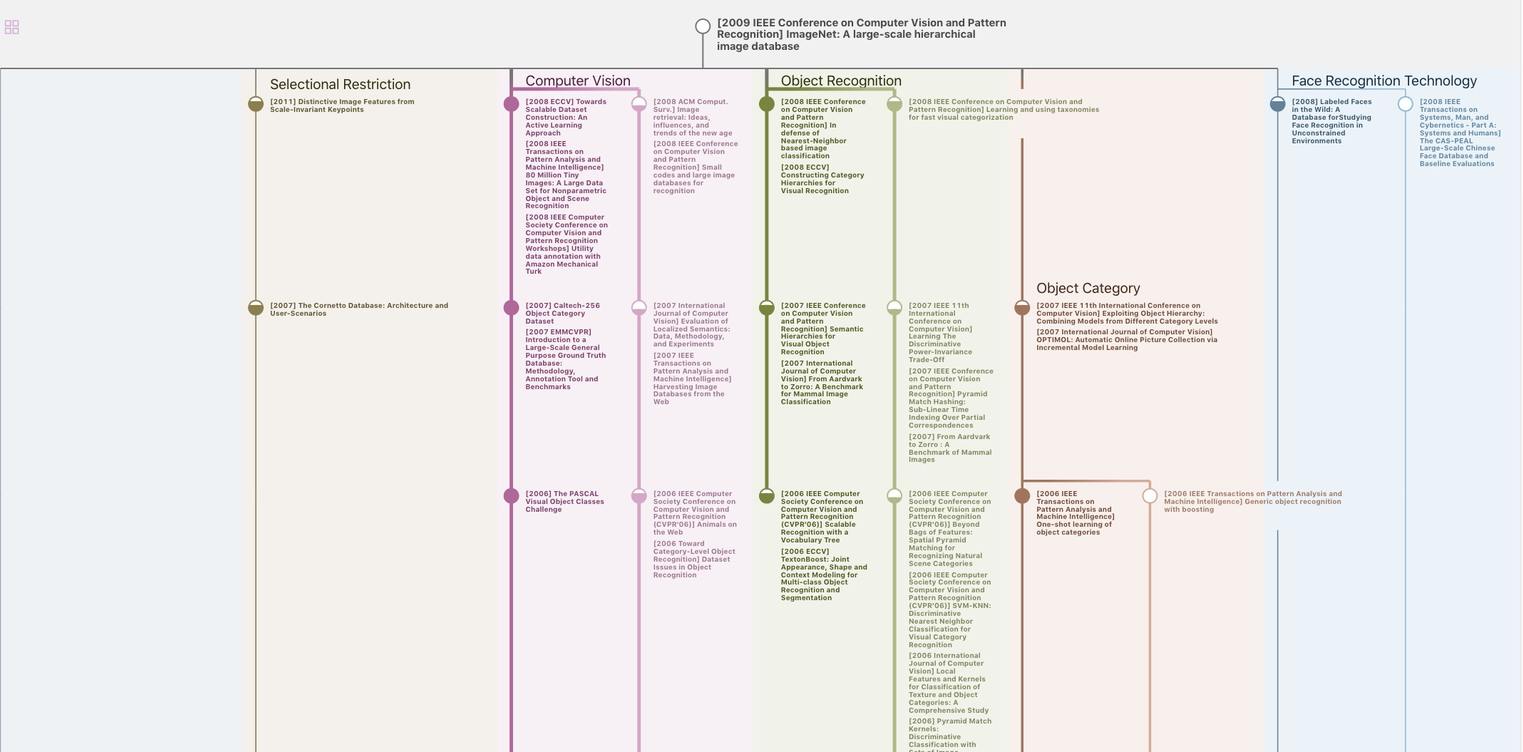
生成溯源树,研究论文发展脉络
Chat Paper
正在生成论文摘要