Stochastic Graph Bandit Learning with Side-Observations
CoRR(2023)
摘要
In this paper, we investigate the stochastic contextual bandit with general function space and graph feedback. We propose an algorithm that addresses this problem by adapting to both the underlying graph structures and reward gaps. To the best of our knowledge, our algorithm is the first to provide a gap-dependent upper bound in this stochastic setting, bridging the research gap left by the work in [35]. In comparison to [31,33,35], our method offers improved regret upper bounds and does not require knowledge of graphical quantities. We conduct numerical experiments to demonstrate the computational efficiency and effectiveness of our approach in terms of regret upper bounds. These findings highlight the significance of our algorithm in advancing the field of stochastic contextual bandits with graph feedback, opening up avenues for practical applications in various domains.
更多查看译文
关键词
stochastic graph bandit learning,side-observations
AI 理解论文
溯源树
样例
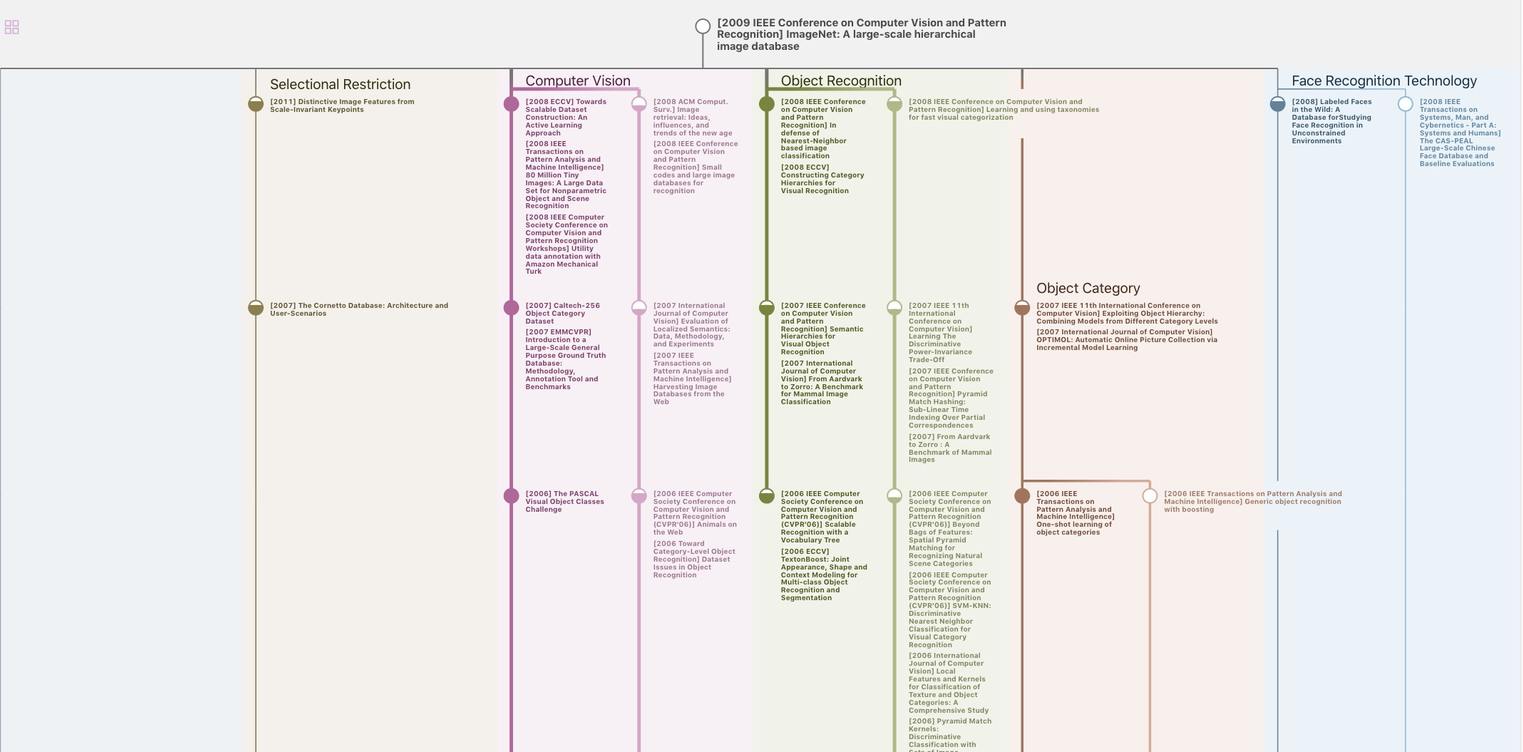
生成溯源树,研究论文发展脉络
Chat Paper
正在生成论文摘要