Robot Manipulation Task Learning by Leveraging SE(3) Group Invariance and Equivariance
CoRR(2023)
摘要
This paper presents a differential geometric control approach that leverages SE(3) group invariance and equivariance to increase transferability in learning robot manipulation tasks that involve interaction with the environment. Specifically, we employ a control law and a learning representation framework that remain invariant under arbitrary SE(3) transformations of the manipulation task definition. Furthermore, the control law and learning representation framework are shown to be SE(3) equivariant when represented relative to the spatial frame. The proposed approach is based on utilizing a recently presented geometric impedance control (GIC) combined with a learning variable impedance control framework, where the gain scheduling policy is trained in a supervised learning fashion from expert demonstrations. A geometrically consistent error vector (GCEV) is fed to a neural network to achieve a gain scheduling policy that remains invariant to arbitrary translation and rotations. A comparison of our proposed control and learning framework with a well-known Cartesian space learning impedance control, equipped with a Cartesian error vector-based gain scheduling policy, confirms the significantly superior learning transferability of our proposed approach. A hardware implementation on a peg-in-hole task is conducted to validate the learning transferability and feasibility of the proposed approach.
更多查看译文
关键词
group invariance
AI 理解论文
溯源树
样例
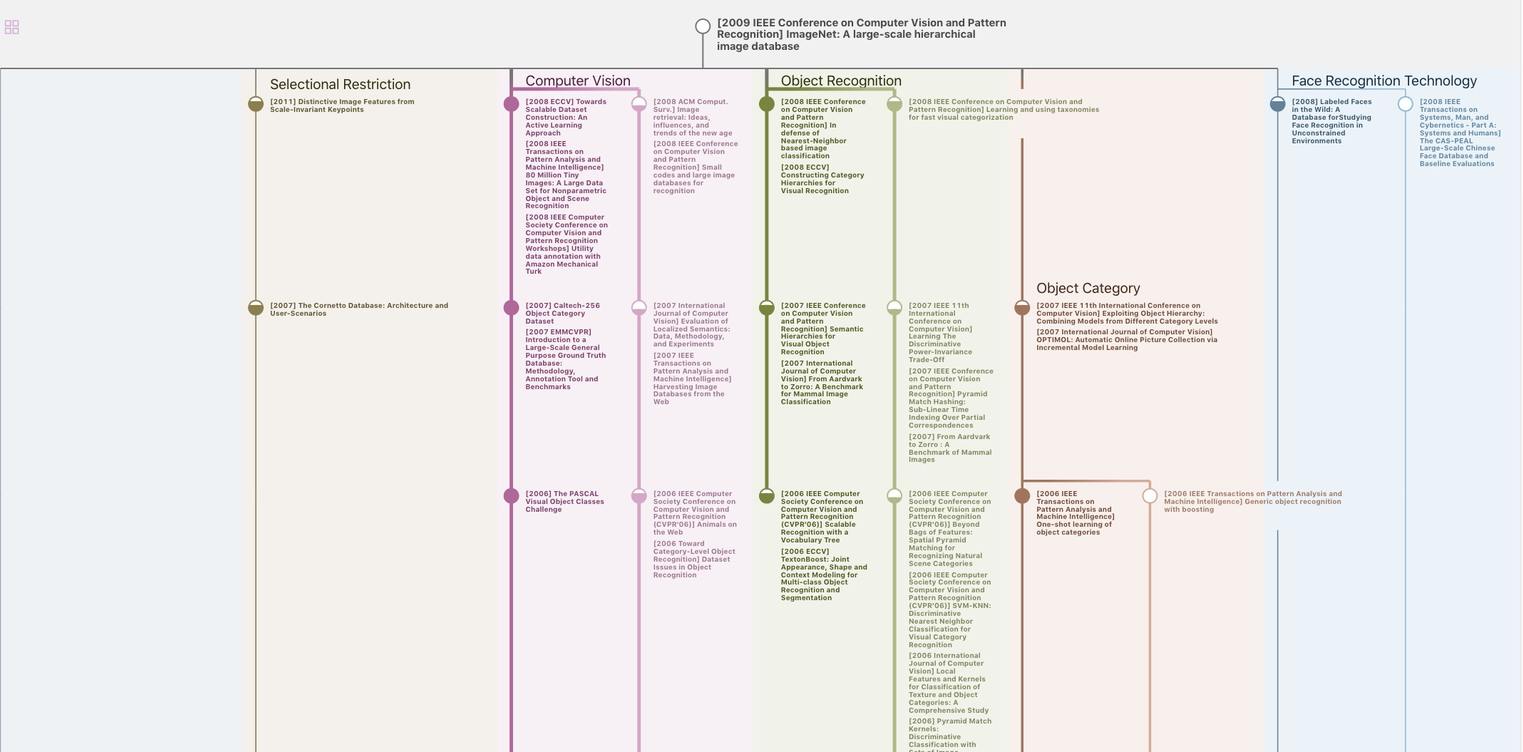
生成溯源树,研究论文发展脉络
Chat Paper
正在生成论文摘要