Continual Learning for Generative Retrieval over Dynamic Corpora
PROCEEDINGS OF THE 32ND ACM INTERNATIONAL CONFERENCE ON INFORMATION AND KNOWLEDGE MANAGEMENT, CIKM 2023(2023)
摘要
Generative retrieval (GR) directly predicts the identifiers of relevant documents (i.e., docids) based on a parametric model. It has achieved solid performance on many ad-hoc retrieval tasks. So far, these tasks have assumed a static document collection. In many practical scenarios, however, document collections are dynamic, where new documents are continuously added to the corpus. The ability to incrementally index new documents while preserving the ability to answer queries with both previously and newly indexed relevant documents is vital to applying GR models. In this paper, we address this practical continual learning problem for GR. We put forward a novel Continual-LEarner for generatiVE Retrieval (CLEVER) model and make two major contributions to continual learning for GR: (i) To encode new documents into docids with low computational cost, we present Incremental Product Quantization, which updates a partial quantization codebook according to two adaptive thresholds; and (ii) To memorize new documents for querying without forgetting previous knowledge, we propose a memory-augmented learning mechanism, to form meaningful connections between old and new documents. Empirical results demonstrate the effectiveness and efficiency of the proposed model.
更多查看译文
关键词
Document increment,Generative retrieval,Product quantization
AI 理解论文
溯源树
样例
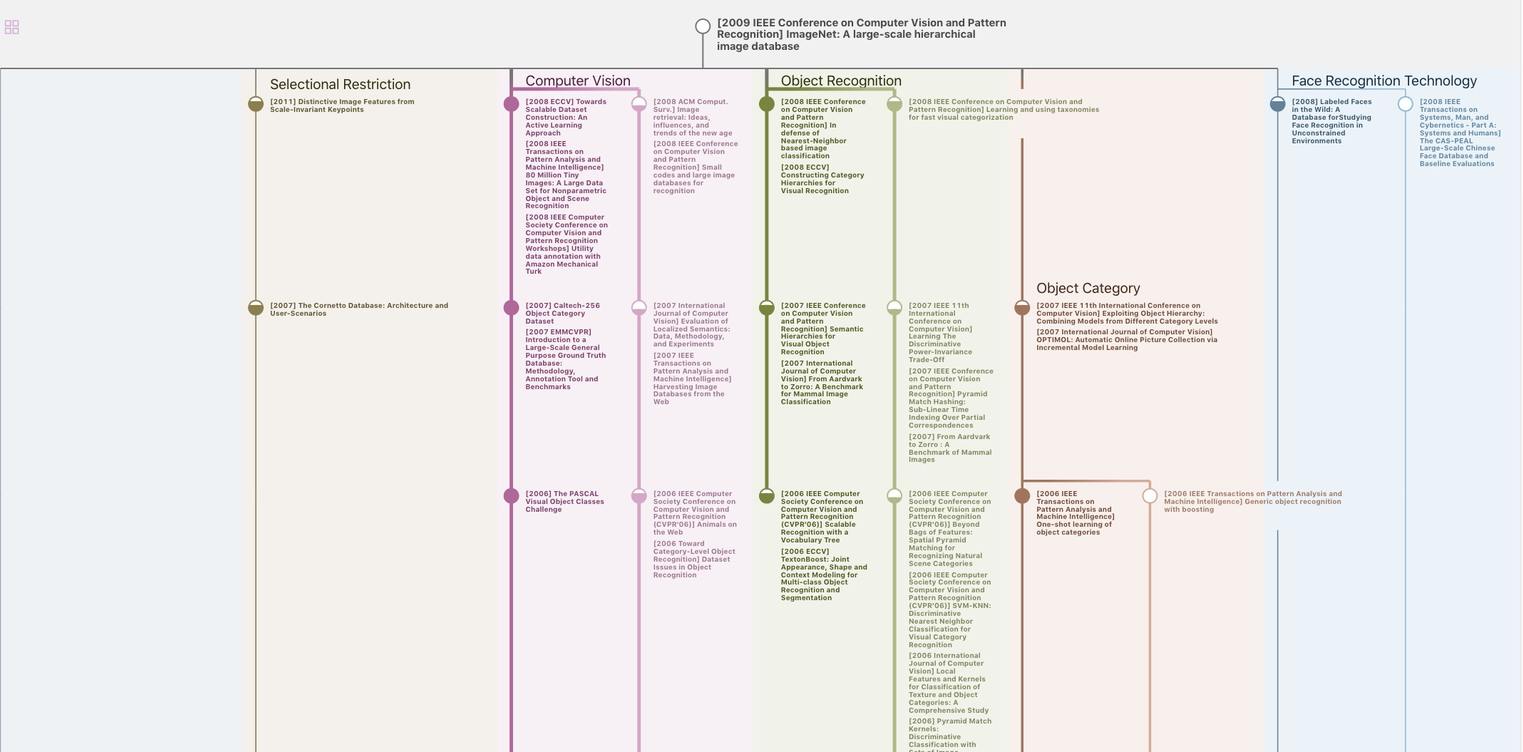
生成溯源树,研究论文发展脉络
Chat Paper
正在生成论文摘要