Rep2wav: Noise Robust text-to-speech Using self-supervised representations
CoRR(2023)
摘要
Benefiting from the development of deep learning, text-to-speech (TTS) techniques using clean speech have achieved significant performance improvements. The data collected from real scenes often contain noise and generally needs to be denoised by speech enhancement models. Noise-robust TTS models are often trained using the enhanced speech, which thus suffer from speech distortion and background noise that affect the quality of the synthesized speech. Meanwhile, it was shown that self-supervised pre-trained models exhibit excellent noise robustness on many speech tasks, implying that the learned representation has a better tolerance for noise perturbations. In this work, we therefore explore pre-trained models to improve the noise robustness of TTS models. Based on HIFI-GAN we first propose a representation-to-waveform vocoder, which aims to learn to map the representation of pre-trained models to the waveform. We then propose a text-to-representation Fastspeech2 model, which aims to learn to map text to pre-trained model representations. Experimental results on the LJSpeech and LibriTTS datasets show that our method outperforms those using speech enhancement methods in both subjective and objective metrics. Audio samples are available at: https://zqs01.github.io/rep2wav/.
更多查看译文
关键词
noise,text-to-speech,self-supervised
AI 理解论文
溯源树
样例
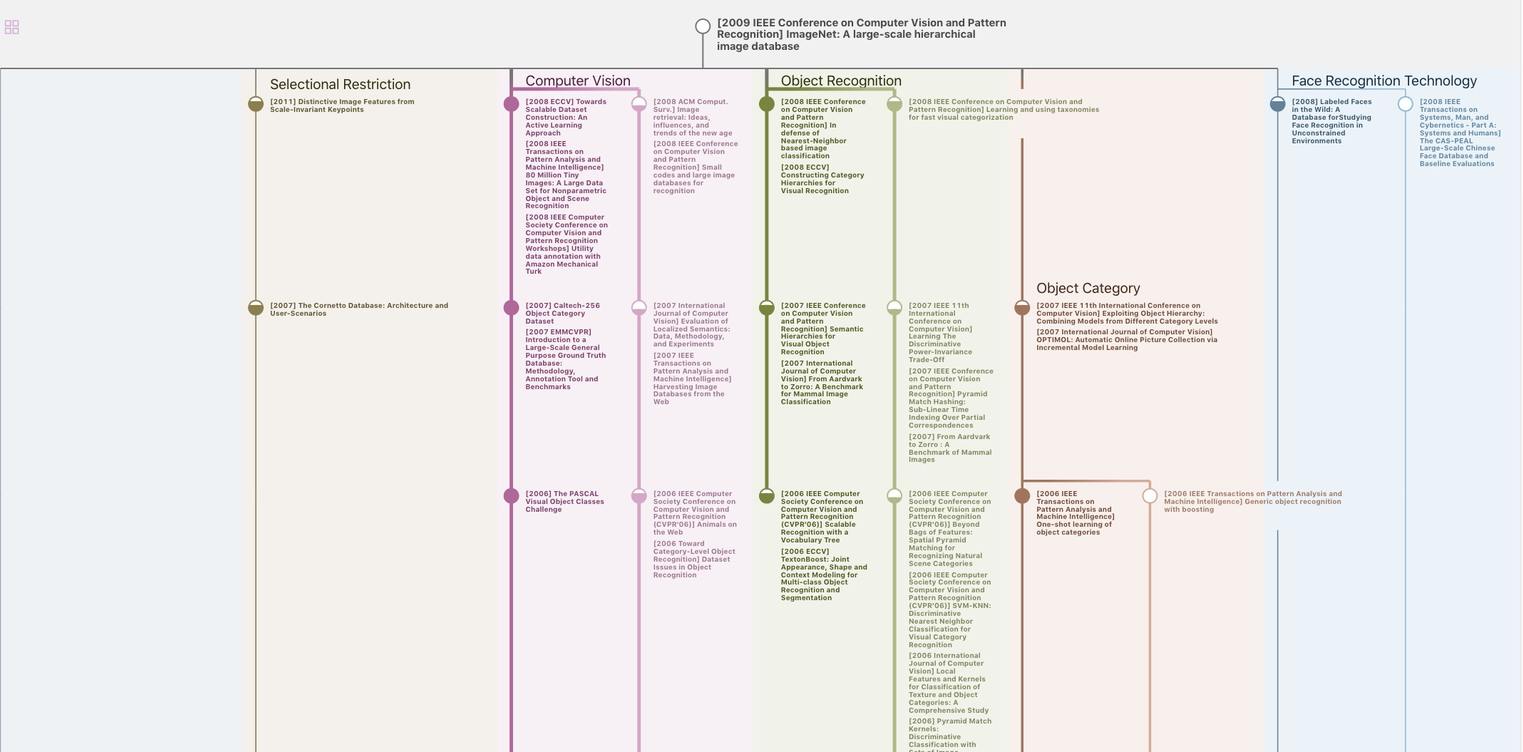
生成溯源树,研究论文发展脉络
Chat Paper
正在生成论文摘要