Data-Driven Robust Control Using Prediction Error Bounds Based on Perturbation Analysis
CoRR(2023)
摘要
For linear systems, many data-driven control methods rely on the behavioral framework, using historical data of the system to predict the future trajectories. However, measurement noise introduces errors in predictions. When the noise is bounded, we propose a method for designing historical experiments that enable the computation of an upper bound on the prediction error. This approach allows us to formulate a minimax control problem where robust constraint satisfaction is enforced. We derive an upper bound on the suboptimality gap of the resulting control input sequence compared to optimal control utilizing accurate measurements. As demonstrated in numerical experiments, the solution derived by our method can achieve constraint satisfaction and a small suboptimality gap despite the measurement noise.
更多查看译文
关键词
robust control,prediction error,data-driven
AI 理解论文
溯源树
样例
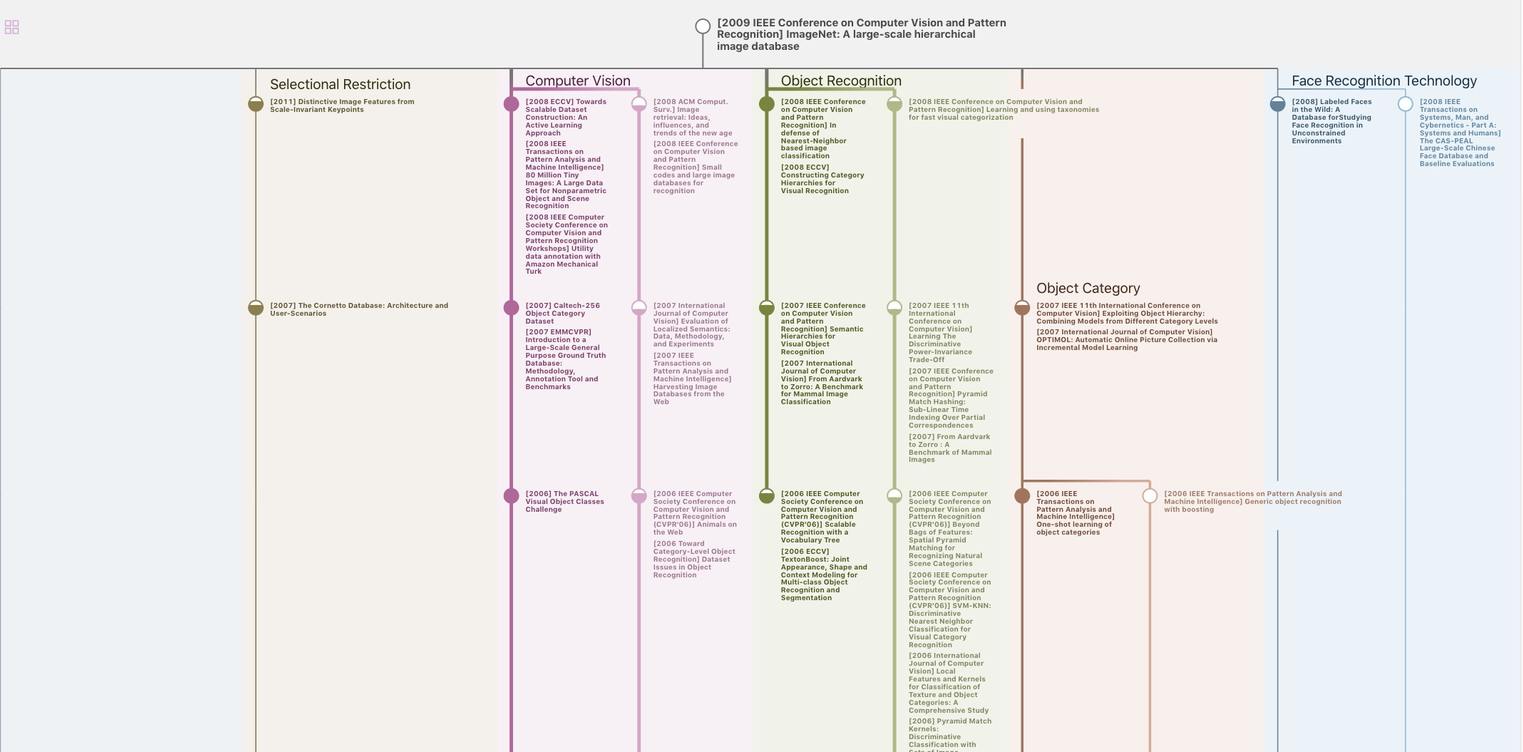
生成溯源树,研究论文发展脉络
Chat Paper
正在生成论文摘要