Towards Fast and Accurate Image-Text Retrieval With Self-Supervised Fine-Grained Alignment
IEEE TRANSACTIONS ON MULTIMEDIA(2024)
摘要
Image-text retrieval requires the system to bridge the heterogenous gap between vision and language for accurate retrieval while keeping the network lightweight-enough for efficient retrieval. Existing trade-off solutions mainly study from the view of incorporating cross-modal interactions with the independent-embedding framework or leveraging stronger pre-trained encoders, which still demand time-consuming similarity measurement or heavyweight model structure in the retrieval stage. In this work, we propose an image-text alignment module SelfAlign on top of the independent-embedding framework, which improves the retrieval accuracy while maintains the retrieval efficiency without extra supervision. SelfAlign contains two collaborative sub-modules that force image-text alignment at both the concept level and context level by self-supervised contrastive learning. It doesn't require cross-modal embedding interactions during training while maintaining independent image and text encoders during retrieval. With comparable time cost, SelfAlign consistently boosts the accuracy of state-of-the-art non-pre-training independent-embedding models respectively by 9.1%, 4.2%, and 6.6% in terms of R@sum score on Flickr30 K, MS-COCO 1 K and MS-COCO 5 K datasets. The retrieval accuracy also outperforms most existing interactive-embedding models with orders of magnitude decrease in retrieval time. The source code is available at: https://github.com/Zjamie813/SelfAlign.
更多查看译文
关键词
Visualization,Semantics,Image coding,Training,Encoding,Computational modeling,Costs,Fast image-text retrieval,concept-level cross-modal alignment,context-level cross-modal alignment,self-supervised learning
AI 理解论文
溯源树
样例
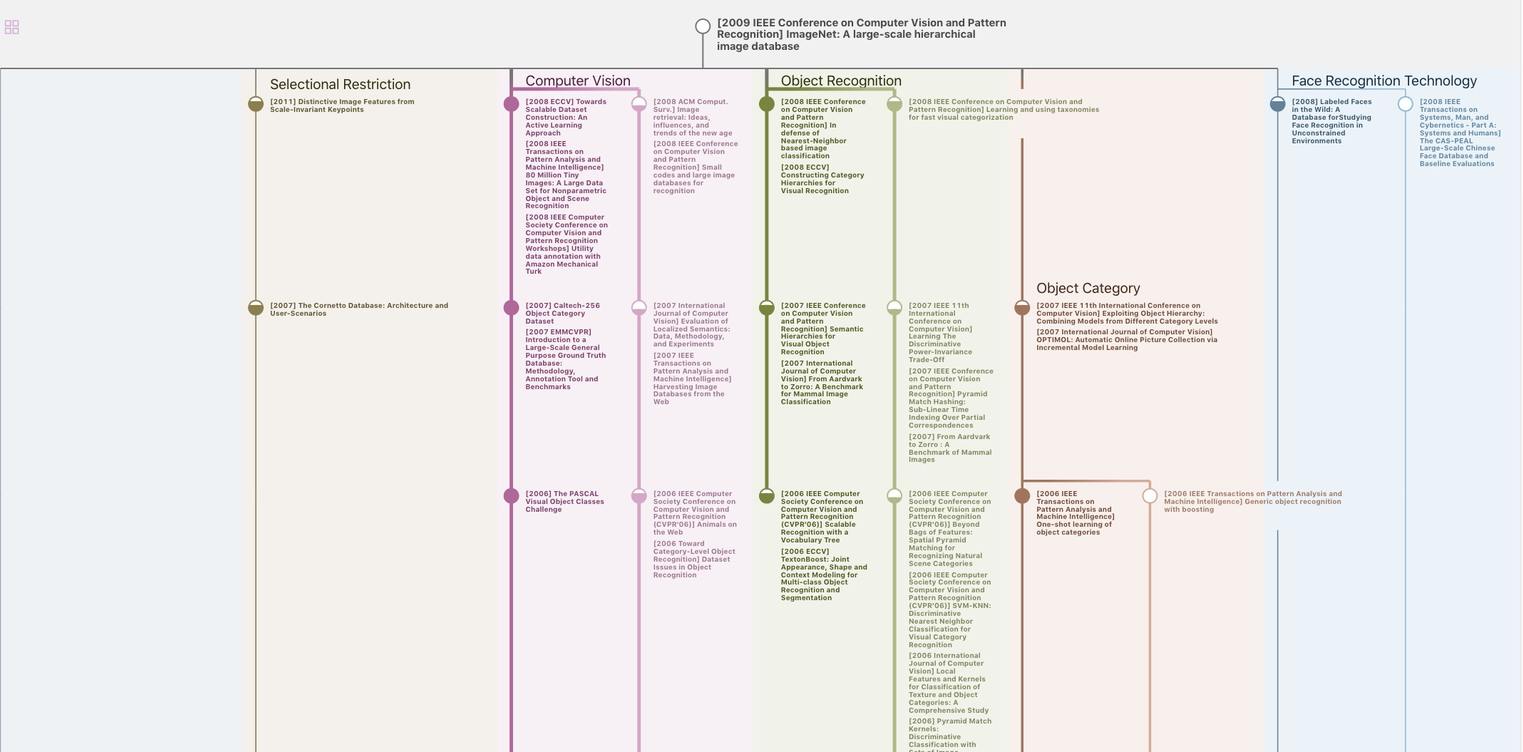
生成溯源树,研究论文发展脉络
Chat Paper
正在生成论文摘要