A VMiPG method for composite optimization with nonsmooth term having no closed-form proximal mapping
arxiv(2023)
Abstract
This paper concerns the minimization of the sum of a twice continuously
differentiable function f and a nonsmooth convex function g without
closed-form proximal mapping. For this class of nonconvex and nonsmooth
problems, we propose a line-search based variable metric inexact proximal
gradient (VMiPG) method with uniformly bounded positive definite variable
metric linear operators. This method computes in each step an inexact minimizer
of a strongly convex model such that the difference between its objective value
and the optimal value is controlled by its squared distance from the current
iterate, and then seeks an appropriate step-size along the obtained direction
with an armijo line-search criterion. We prove that the iterate sequence
converges to a stationary point when f and g are definable in the same
o-minimal structure over the real field (ℝ,+,·), and if addition
the objective function f+g is a KL function of exponent 1/2, the
convergence has a local R-linear rate. The proposed VMiPG method with the
variable metric linear operator constructed by the Hessian of the function f
is applied to the scenario that f and g have common composite structure,
and numerical comparison with a state-of-art variable metric line-search
algorithm indicates that the Hessian-based VMiPG method has a remarkable
advantage in terms of the quality of objective values and the running time for
those difficult problems such as high-dimensional fused weighted-lasso
regressions.
MoreTranslated text
AI Read Science
Must-Reading Tree
Example
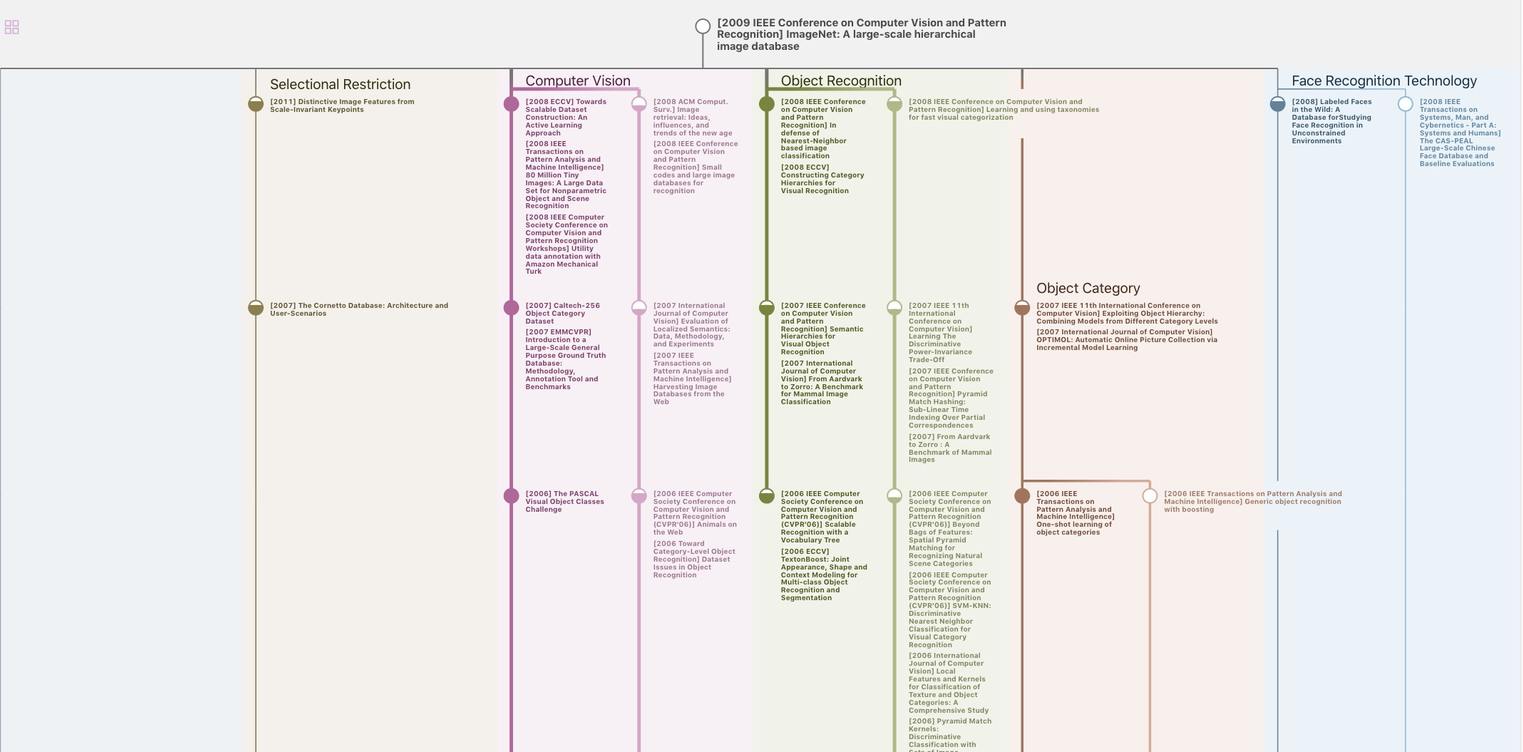
Generate MRT to find the research sequence of this paper
Chat Paper
Summary is being generated by the instructions you defined