Multimodal Pain Recognition in Postoperative Patients: A Machine Learning Approach
medRxiv (Cold Spring Harbor Laboratory)(2023)
摘要
Objective To develop and evaluate a multimodal machine learning-based objective pain assessment algorithm on data collected from post-operative patients.
Methods The proposed method addresses the major challenges that come with using data from such patients like the imbalanced distribution of pain classes and the scarcity of ground-truth labels. Specifically, we extracted automatic features using a convolutional autoencoder (AE) along with data augmentation techniques like weak supervision and minority oversampling to improve our models’ predictive performance. This method was used in conjunction with four different machine learning classifiers: Adaptive Boosting (AdaBoost), Random Forest (RF), Support Vector Machine (SVM), and K-Nearest Neighbors (KNN) to perform binary classification on three increasing levels of pain when compared to no pain.
Results Our models are able to recognize different pain levels with an average balanced accuracy of over 80%.
Conclusion This is the first multimodal pain recognition work done on postoperative patients and our proposed method provides valuable insights for automatic acute pain recognition in such patients.
### Competing Interest Statement
The authors have declared no competing interest.
### Funding Statement
The author(s) received no specific funding for this work.
### Author Declarations
I confirm all relevant ethical guidelines have been followed, and any necessary IRB and/or ethics committee approvals have been obtained.
Yes
The details of the IRB/oversight body that provided approval or exemption for the research described are given below:
Written consent was received from the University of California, Irvine Institutional Review Board Human Research Protections HS# 2017-3747.
I confirm that all necessary patient/participant consent has been obtained and the appropriate institutional forms have been archived, and that any patient/participant/sample identifiers included were not known to anyone (e.g., hospital staff, patients or participants themselves) outside the research group so cannot be used to identify individuals.
Yes
I understand that all clinical trials and any other prospective interventional studies must be registered with an ICMJE-approved registry, such as ClinicalTrials.gov. I confirm that any such study reported in the manuscript has been registered and the trial registration ID is provided (note: if posting a prospective study registered retrospectively, please provide a statement in the trial ID field explaining why the study was not registered in advance).
Yes
I have followed all appropriate research reporting guidelines, such as any relevant EQUATOR Network research reporting checklist(s) and other pertinent material, if applicable.
Yes
The data used in this study include sensitive health information that cannot be publicly shared due to ethical restrictions outlined in the informed consent. Permission to use the data was granted by the participants solely for the specific purpose described in the consent. Data access requires individual consent or approval from the Institutional Review Board. Researchers interested in accessing the data should contact the project’s PI, Associate Professor Amir Rahmani, at a.rahmani{at}uci.edu, located at the University of California, Irvine, Institute for Future Health, Irvine, California, United States, 92617.
更多查看译文
关键词
multimodal pain recognition,postoperative patients,machine learning
AI 理解论文
溯源树
样例
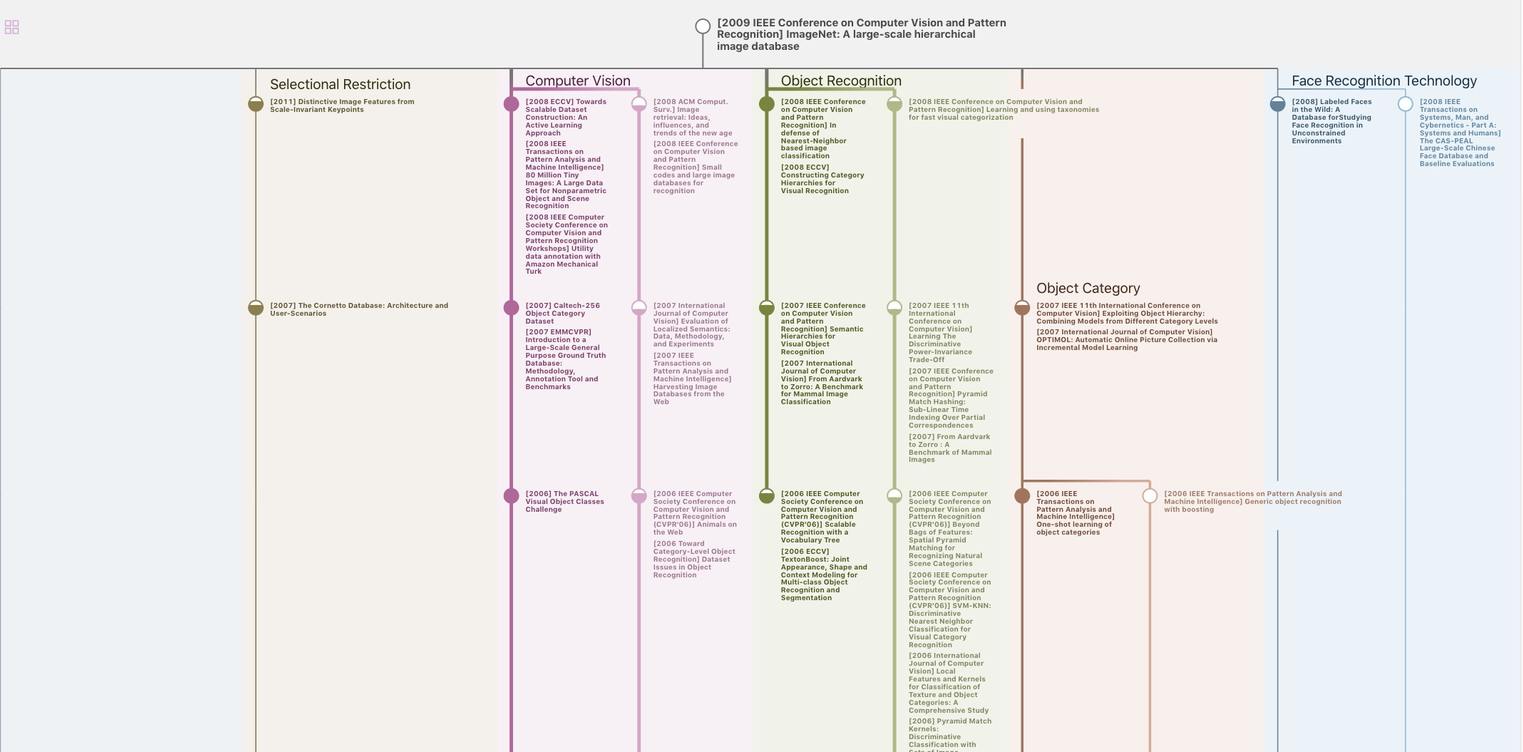
生成溯源树,研究论文发展脉络
Chat Paper
正在生成论文摘要