Comparison of Machine Learning Methods for Human Activity Recognition Using Pseudo Free-Living Data
2022 International Conference on Computational Science and Computational Intelligence (CSCI)(2022)
摘要
Recognition of human activities has become more critical in recent years. Practical approaches for classifying physical activities are becoming required in the scientific community, and such models are needed explicitly in health promotion and behavior. The data collected in real-world conditions differ from laboratory data, which has been the main focus of much research. As a result, in this work, we analyzed several machine-learning techniques and models for identifying human activity using a pseudo-free-living dataset obtained at the University of Georgia. We found that hierarchical meta-classifiers outperformed deep learning and classical models by 6% for classifying seven activities. Model personalization is promoted since it lowers the inter-subject variability of the dataset. We divided activities based on the Metabolic Equivalent of Task (MET), and we achieved 80% inter-subject accuracy and 87% accuracy by including 50% of the participant's data. Achieving high performance for machine learning models is challenging using real-world data.
更多查看译文
关键词
Supervised Classification,Human Activity Recognition,Random Forest,Deep Learning,Machine learning Introduction,METs
AI 理解论文
溯源树
样例
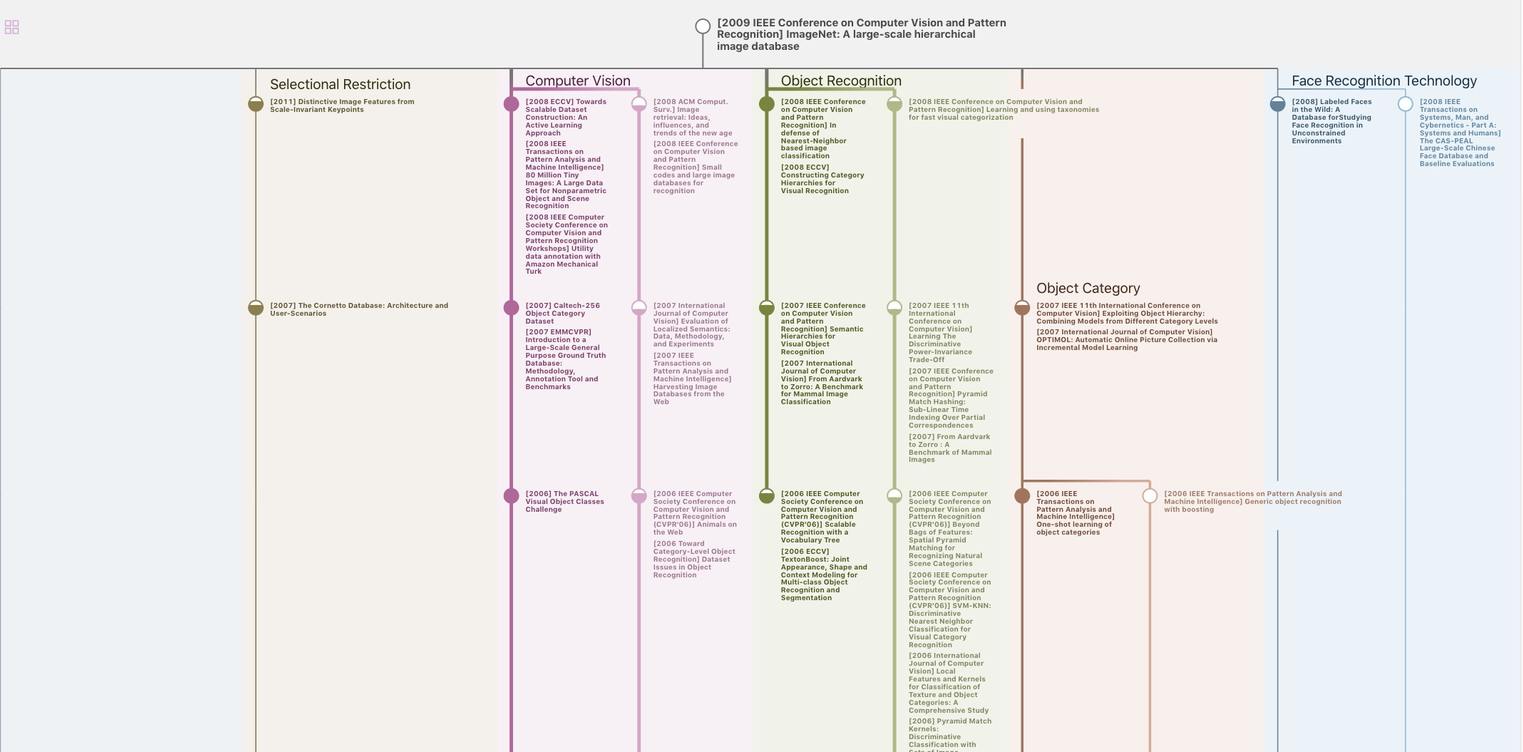
生成溯源树,研究论文发展脉络
Chat Paper
正在生成论文摘要