Multimodal Interactive Supervised Pedestrian Detection Based on yolov5
2023 3rd International Symposium on Computer Technology and Information Science (ISCTIS)(2023)
Abstract
Pedestrian detection and recognition is one of the important and fundamental tasks of environmental awareness in autonomous driving. Some existing research methods are based on visual images (RGB) for detection, but detection methods that only use visual images cannot meet harsh detection environments, such as in cloudy, rainy, foggy, and poor lighting environments, where the detection effect is poor. In recent years, fusion detection based on visual images (RGB) and thermal images (Thermal) has received increasing attention, but there are still some problems in fusion strategies. The purpose of RGB Thermal pedestrian detection is to fuse complementary visible light images and thermal infrared information to improve the performance of pedestrian detection in day and night environments [1]. In recent years, many experts and scholars have done a lot of research work on this issue [2]–[6], effectively integrating the two modal data of RGB and thermal infrared images, and have achieved some results. However, these methods directly use fused features for pedestrian detection, without considering the problem that the quality of the resulting fused features may not be high. Therefore, multimodal feature fusion pedestrian detection structures need to improve the quality of fusion features, which plays a crucial role in the research of multimodal data fusion pedestrian detection in autonomous driving. The Cross-Modal Supervision (CMS) model designed and implemented in this article has been experimentally verified on the public Kaist [7] dataset. The experimental results show that the accuracy of the cross modal supervised model on the Kaist dataset reaches 53.68°/
°
and the miss rate decreases to 46.32°/
°
.
MoreTranslated text
Key words
Pedestrian detection and identification,Deep learning,Multimodal,Feature selection,Cross modal supervision
AI Read Science
Must-Reading Tree
Example
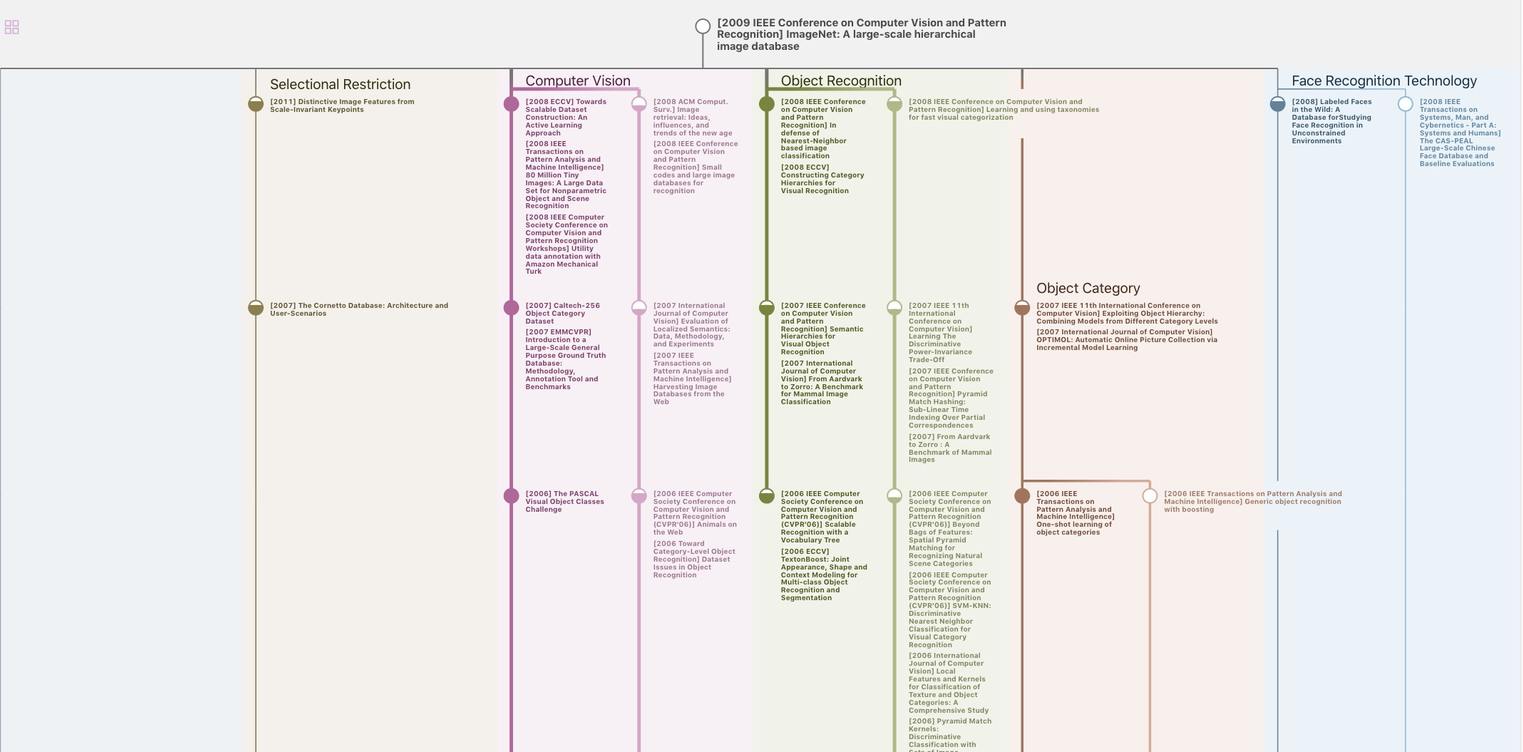
Generate MRT to find the research sequence of this paper
Chat Paper
Summary is being generated by the instructions you defined