A Modified Aquila-Based Optimized XGBoost Framework for Detecting Probable Seizure Status in Neonates
Sensors (Basel, Switzerland)(2023)
摘要
Electroencephalography (EEG) is increasingly being used in pediatric neurology and pro-vides opportunities to diagnose various brain illnesses more accurately and precisely. It is thought to be one of the most effective tools for identifying newborn seizures, especially in Neonatal Inten-sive Care Units (NICUs). However, EEG interpretation is time-consuming and requires specialists with extensive training. It can be challenging and time-consuming to distinguish between seizures since they might have a wide range of clinical characteristics and etiologies. Technological advance-ments such as the Machine Learning (ML) approach for the rapid and automated diagnosis of new-born seizures have increased in recent years. This work proposes a novel optimized ML framework to eradicate the constraints of conventional seizure detection techniques. Moreover, we modified a novel meta-heuristic optimization algorithm (MHOA), named Aquila Optimization (AO), to de-velop an optimized model to make our proposed framework more efficient and robust. To conduct a comparison-based study, we also examined the performance of our optimized model with that of other classifiers, including the Decision Tree (DT), Random Forest (RF), and Gradient Boosting Classifier (GBC). This framework was validated on a public dataset of Helsinki University Hospital, where EEG signals were collected from 79 neonates. Our proposed model acquired encouraging re-sults showing a 93.38% Accuracy Score, 93.9% Area Under the Curve (AUC), 92.72% F1 score, 65.17% Kappa, 93.38% sensitivity, and 77.52% specificity. Thus, it outperforms most of the present shallow ML architectures by showing improvements in accuracy and AUC scores. We believe that these re-sults indicate a major advance in the detection of newborn seizures, which will benefit the medical community by increasing the reliability of the detection process.
更多查看译文
关键词
neonatal seizure,EEG,shallow learning,classification,optimization
AI 理解论文
溯源树
样例
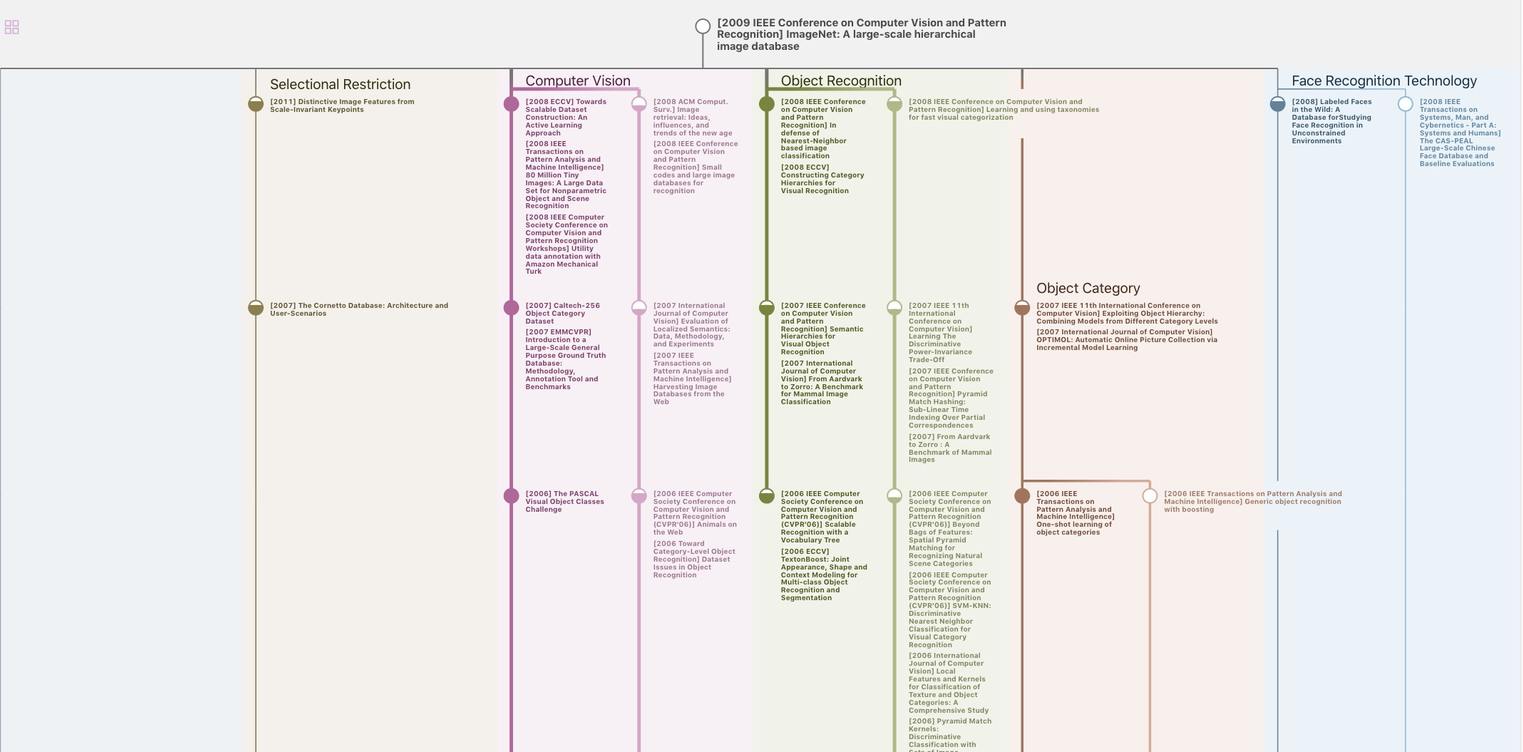
生成溯源树,研究论文发展脉络
Chat Paper
正在生成论文摘要