Communication-Efficient and Privacy-Preserving Verifiable Aggregation for Federated Learning.
Entropy (Basel, Switzerland)(2023)
摘要
Federated learning is a distributed machine learning framework, which allows users to save data locally for training without sharing data. Users send the trained local model to the server for aggregation. However, untrusted servers may infer users' private information from the provided data and mistakenly execute aggregation protocols to forge aggregation results. In order to ensure the reliability of the federated learning scheme, we must protect the privacy of users' information and ensure the integrity of the aggregation results. This paper proposes an effective secure aggregation verifiable federated learning scheme, which has both high communication efficiency and privacy protection function. The scheme encrypts the gradients with a single mask technology to securely aggregate gradients, thus ensuring that malicious servers cannot deduce users' private information from the provided data. Then the masked gradients are hashed to verify the aggregation results. The experimental results show that our protocol is more suited for bandwidth-constraint and offline-users scenarios.
更多查看译文
关键词
federated learning,homomorphic hash function,privacy protection,verifiability
AI 理解论文
溯源树
样例
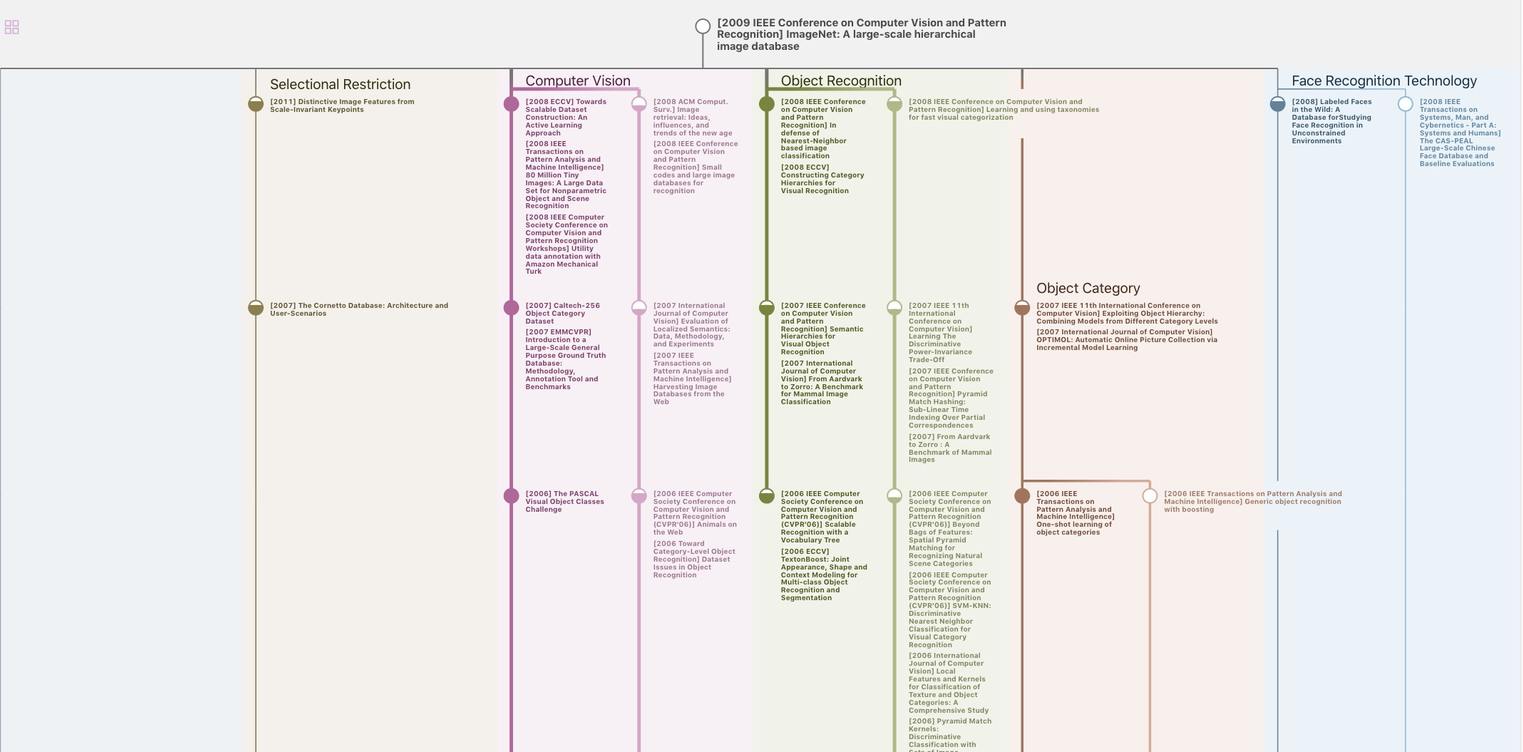
生成溯源树,研究论文发展脉络
Chat Paper
正在生成论文摘要