Prediction of COVID-19 Using a WOA-BILSTM Model
Bioengineering (Basel, Switzerland)(2023)
摘要
The COVID-19 pandemic has had a significant impact on the world, highlighting the importance of the accurate prediction of infection numbers. Given that the transmission of SARS-CoV-2 is influenced by temporal and spatial factors, numerous researchers have employed neural networks to address this issue. Accordingly, we propose a whale optimization algorithm-bidirectional long short-term memory (WOA-BILSTM) model for predicting cumulative confirmed cases. In the model, we initially input regional epidemic data, including cumulative confirmed, cured, and death cases, as well as existing cases and daily confirmed, cured, and death cases. Subsequently, we utilized the BILSTM as the base model and incorporated WOA to optimize the specific parameters. Our experiments employed epidemic data from Beijing, Guangdong, and Chongqing in China. We then compared our model with LSTM, BILSTM, GRU, CNN, CNN-LSTM, RNN-GRU, DES, ARIMA, linear, Lasso, and SVM models. The outcomes demonstrated that our model outperformed these alternatives and retained the highest accuracy in complex scenarios. In addition, we also used Bayesian and grid search algorithms to optimize the BILSTM model. The results showed that the WOA model converged fast and found the optimal solution more easily. Thus, our model can assist governments in developing more effective control measures.
更多查看译文
关键词
COVID-19,infectious disease,BILSTM,WOA (whale optimization algorithm),prediction
AI 理解论文
溯源树
样例
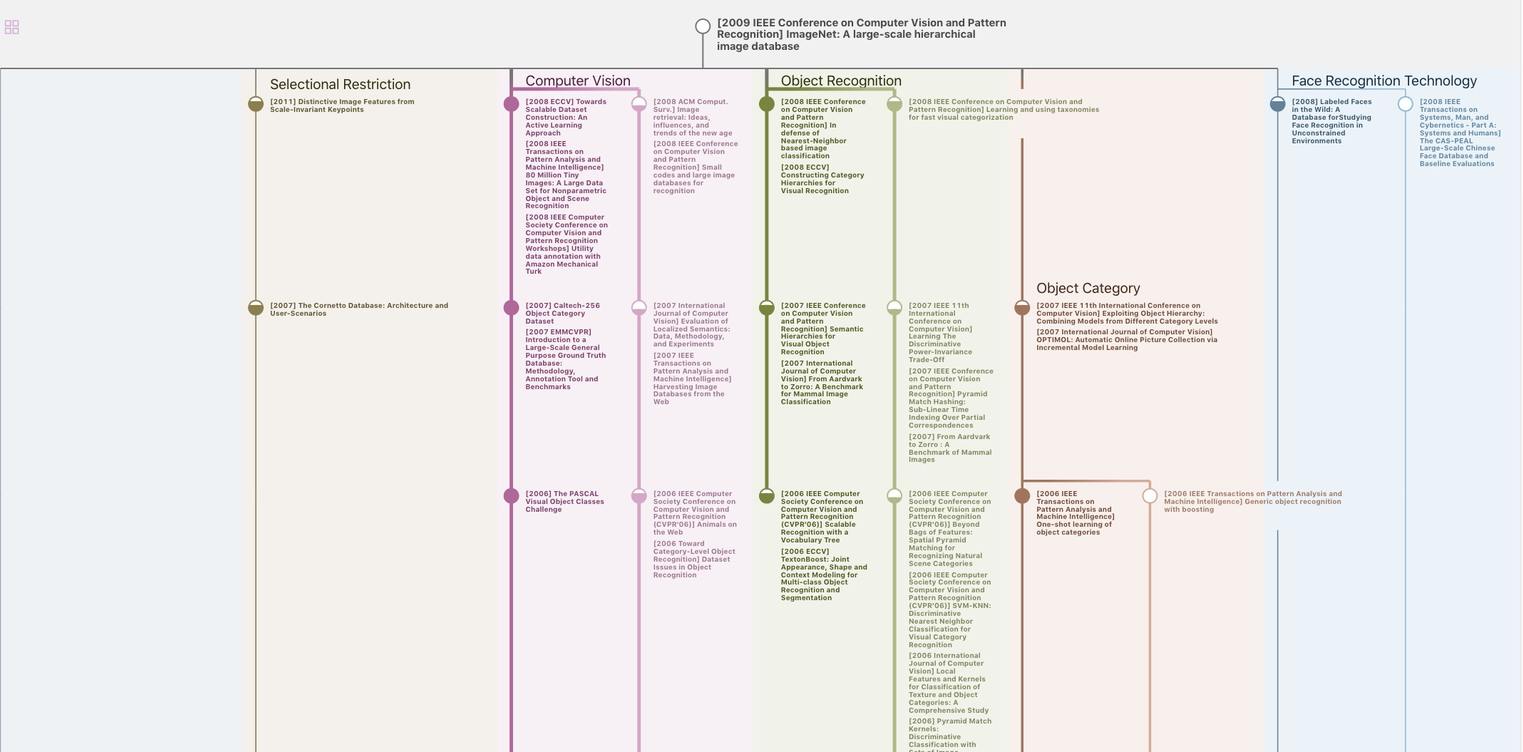
生成溯源树,研究论文发展脉络
Chat Paper
正在生成论文摘要