Intrinsic instability of the dysbiotic microbiome revealed through dynamical systems inference at scale
bioRxiv (Cold Spring Harbor Laboratory)(2021)
摘要
Abstract Dynamical systems models are a powerful tool for analyzing interactions, stability, resilience, and other key properties in biomedically important microbial ecosystems, such as the gut microbiome. Challenges to modeling and inference in this setting include the large number of species present, and data sparsity/noise characteristics. Here, we introduce a Bayesian statistical method, the Microbial Dynamical Systems Inference Engine 2 (MDSINE2), which infers compact and interpretable ecosystems-scale dynamical systems models from microbiome time-series data. We model microbial dynamics as stochastic processes driven by inferred interaction modules, or groups of microbes with similar interaction structure and responses to perturbations. Additionally, we model the noise characteristics of sequencing and qPCR measurements to provide uncertainty quantification for all outputs. To evaluate MDSINE2, and provide a benchmarking resource for the community, we generated the most densely sampled microbiome time-series to date, which consists of a cohort of mice that received fecal transplants from a human donor and were then subjected to dietary and antibiotic perturbations. Benchmarking on simulated and real data demonstrate that MDSINE2 significantly outperforms state-of-the-art methods, and moreover identifies interaction modules that shed new light on ecosystems-scale interactions in the gut microbiome. We provide MDSINE2 as an open-source Python package at: https://github.com/gerberlab/MDSINE2 .
更多查看译文
关键词
dysbiotic microbiome,dynamical systems inference,intrinsic instability
AI 理解论文
溯源树
样例
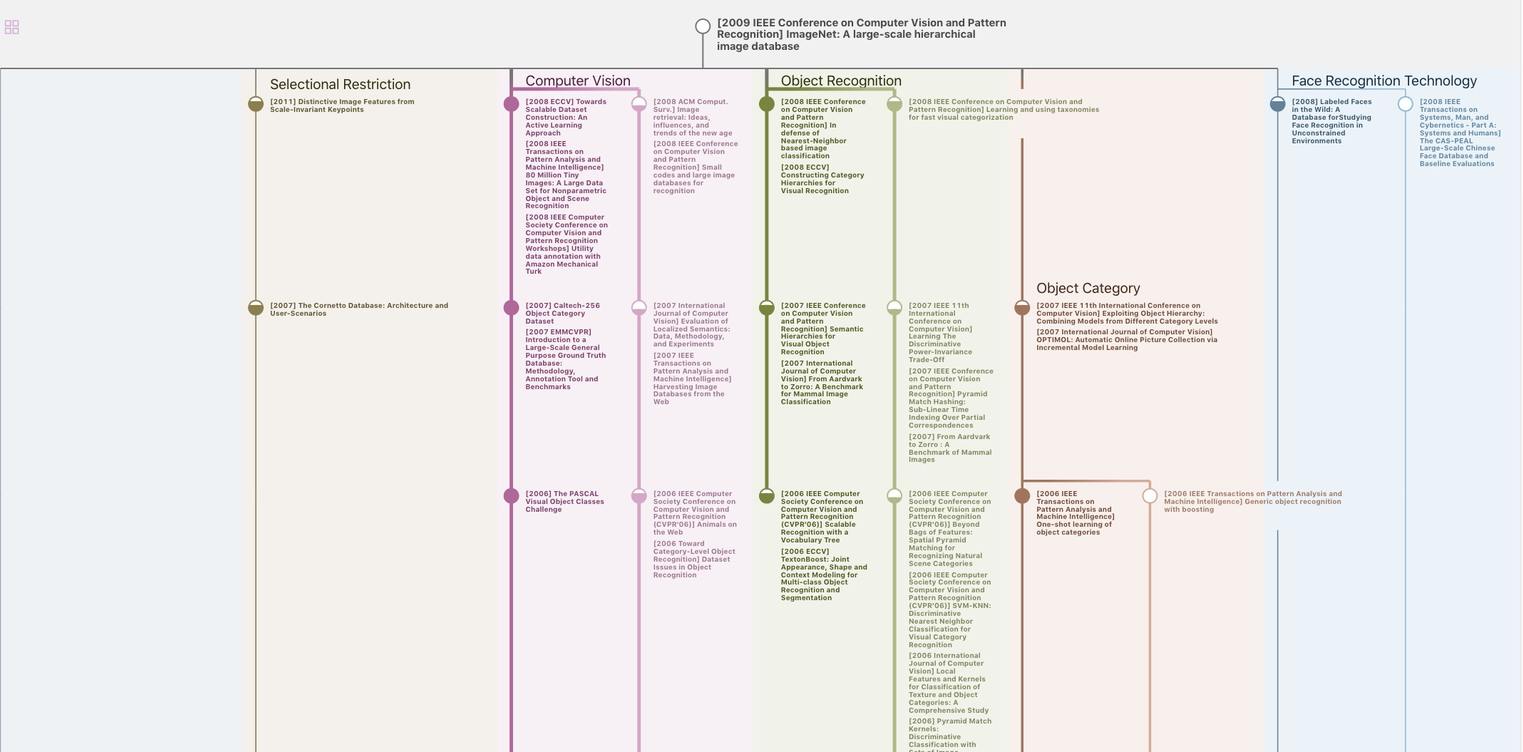
生成溯源树,研究论文发展脉络
Chat Paper
正在生成论文摘要