Efficient Hash Coding for Image Retrieval Based on Improved Center Generation and Contrastive Pre-training Knowledge Model.
Lecture Notes in Computer Science(2023)
摘要
In image retrieval tasks, effective hash coding methods can achieve better similarity preservation, so as to gain efficient retrieval results. Existing methods are usually divided into constructing global center constraints and utilizing local feature constraints. These methods mainly use the category labels of images as numbers, and do not fully exploit the value of classification labels as textual knowledge information. In this paper, we propose a novel and efficient hash coding framework. In the proposed method, the generation method of hash centers is improved, so that each image can correspond to multiple hash centers. In this way, the loss function for local similarity constraint is proposed, and the joint loss function for deep model is designed. Furthermore, based on the text-image pre-trained large model, we extract the text of the category label from the image as knowledge information, which is used to construct better hash codes for the image retrieval query, so as to improve the image retrieval evaluation effect. Extensive experiments on several large-scale datasets verify that the proposed method achieves better results than the state-of-the-art hash coding methods.
更多查看译文
关键词
image retrieval,hash,knowledge,pre-training
AI 理解论文
溯源树
样例
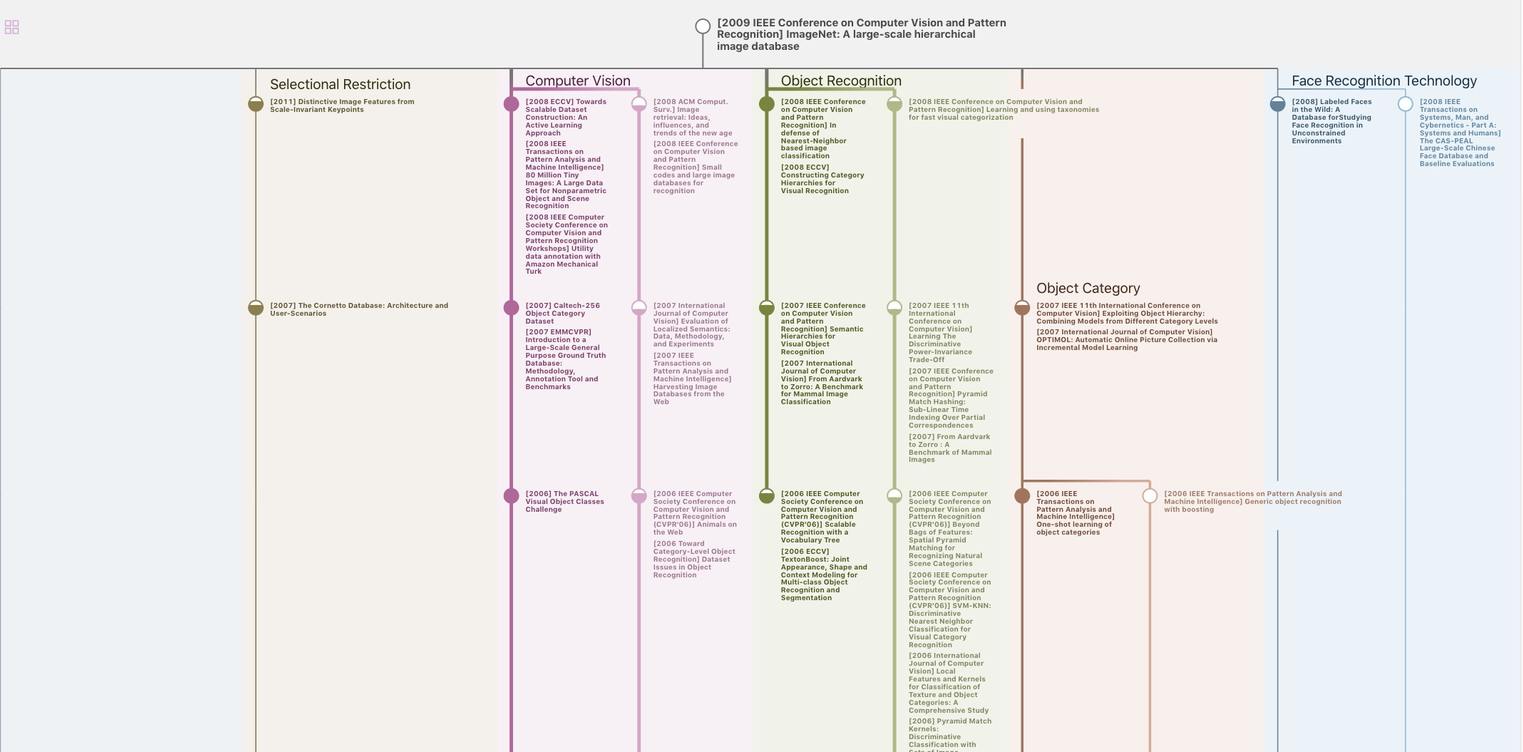
生成溯源树,研究论文发展脉络
Chat Paper
正在生成论文摘要