Performance Evaluation of Machine Learning Models for Cyber Threat Detection and Prevention in Mobile Money Services.
Informatica (Slovenia)(2023)
摘要
In this paper, investigation was made to evaluate the effectiveness of the different classifiers suitable to predict the probability of cyber threat or fraudulent intent applicant during Mobile Money Service on-boarding or service activation process with the goal of determining the best machine learning model for the predictive model solution. Experimental work was carried out by formulating cyber threat predictive models, using six (6) supervised machine learning algorithms, Logistic regression, Naïve Bayes, Shallow Neural Network (SNN), Deep Neural Network (DNN), Classification and Regression Trees (CART) and Random Forest (RF) of different configurations. Each model was simulated with Synthetic Minority Operation Techniques (SMOTE) and without SMOTE (No-SMOTE) application on 25,000 dataset records of mobile money applicants. Twenty-four (24) different configurations of the formulated predictive models were simulated and evaluated using Python programming language. Simulation results of the predictive models proved that the Random Forest model multiclass configurations with SMOTE dataset outperformed all other configurations. The results also showed that the multiclass experiments with SMOTE has a better performance than the binary configuration without SMOTE of the predictive models. The study concluded that using the Random Forest based predictive machine learning model will increase the security level of Mobile Money solution by detecting and preventing anomalous customer registrations during mobile money for the unbanked on-boarding process.
更多查看译文
关键词
mobile money services,cyber threat detection,machine learning models,threat detection,machine learning
AI 理解论文
溯源树
样例
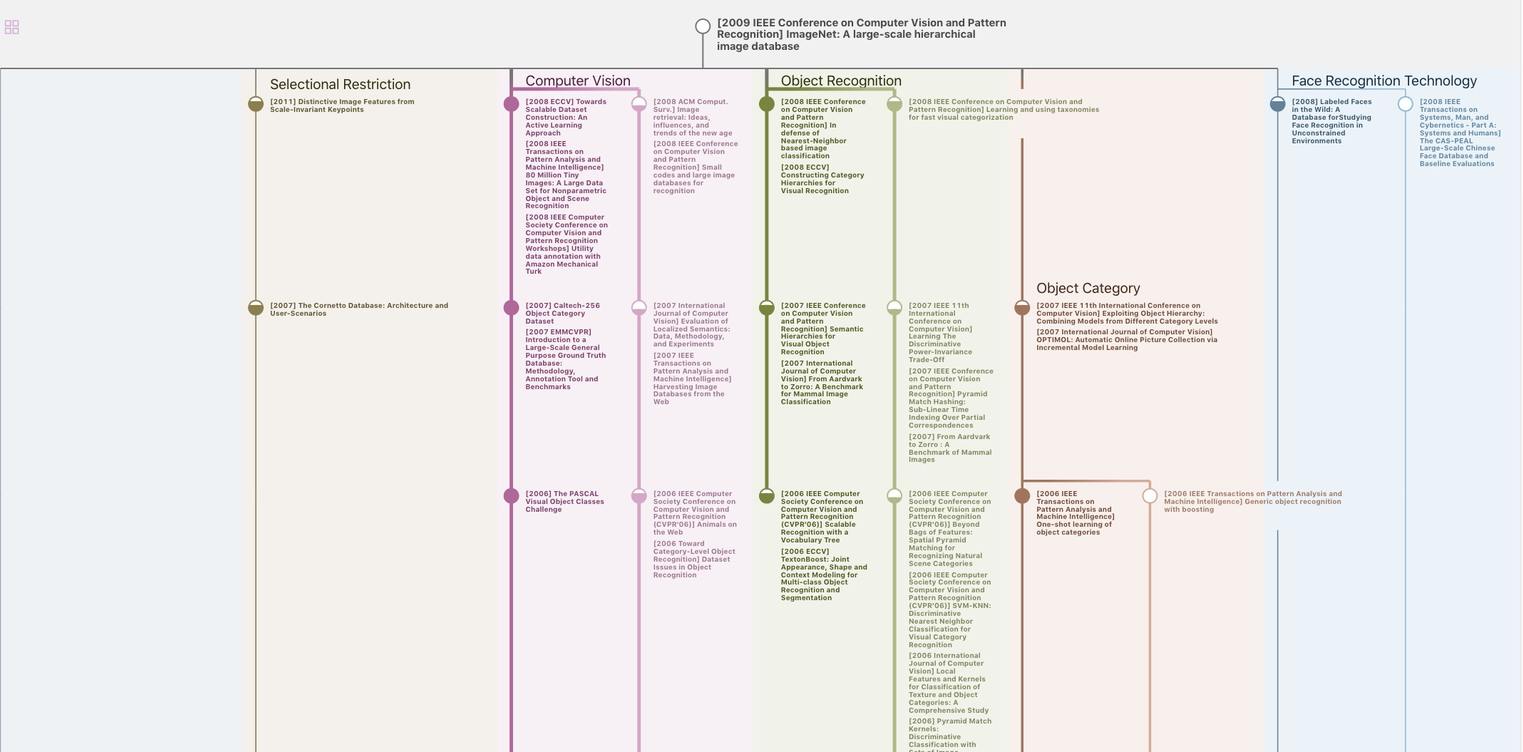
生成溯源树,研究论文发展脉络
Chat Paper
正在生成论文摘要