Low-Rank Tensor Train and Self-Similarity Based Spectral CT Reconstruction
IEEE ACCESS(2024)
Abstract
Spectral computed tomography (CT) has a wide range of applications in material discrimination, clinical diagnosis and tissue representation. However, the photon counting detector measurements are subject to serious quantum noise caused by photon starvation, photon accumulation, charge sharing, and other factors, which will lead to a decrease in the quality of the reconstructed image and make clinical diagnosis more difficult. To tackle with this problem, this paper proposes a spectral CT reconstruction technique that exploits the spatial sparsity of inter-channel images and the high correlation of images between different energy channels. Specifically, similar patches from spatial and spectral domains are extracted to form the low-rank tensors. Then the tensor-train rank, which is derived from a well-balanced matricization technique, is adopted to depict the high correlation among different energy channels. To capture the self-similarity of the low-rank tensors, the L0-norm of the image gradient is employed for image smoothing. An efficient algorithm is devised to solve the reconstruction model utilizing the Alternating Direction Method of Multipliers. For the sake of testing and verifying the effectiveness of the proposed algorithm, numerical simulations and real data experiments are conducted. Qualitatively, the designed method demonstrates a clear advantage in image quality over the existing state-of-the-art algorithms. For instance, when taking the full energy bin image as an example, the proposed method reduces the Root Mean Square Error (RMSE) by 52.07%, 38.69%, 35.13%, 12.67%, respectively, compared to the competing methods. Quantitative and qualitative assessment indices have revealed that the suggested method has excellent noise suppression, artifact elimination, and image detail preservation properties.
MoreTranslated text
Key words
Spectral computed tomography,image reconstruction,non-local similarity,tensor train decomposition,alternating direction method of multipliers
AI Read Science
Must-Reading Tree
Example
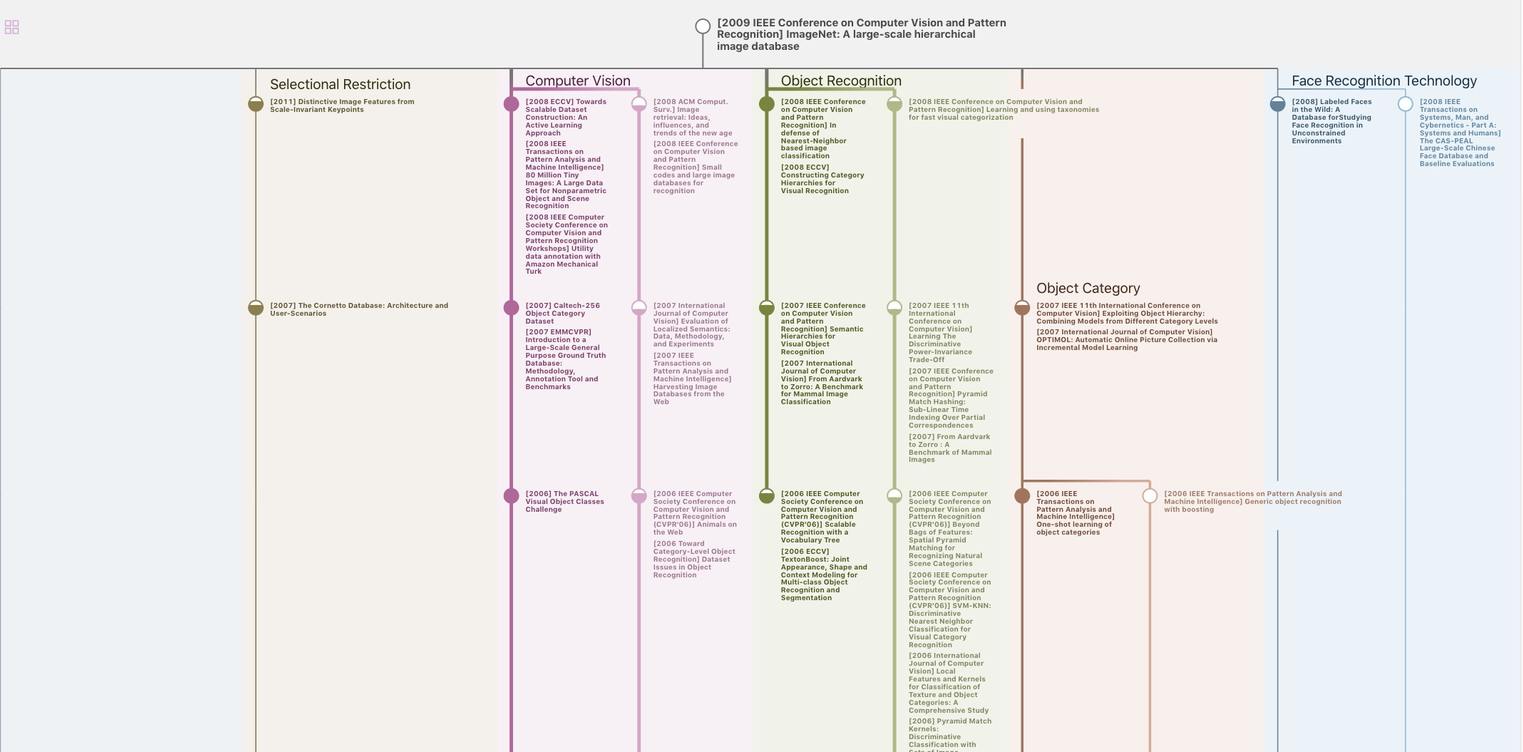
Generate MRT to find the research sequence of this paper
Chat Paper
Summary is being generated by the instructions you defined