Voice Biomarkers for Parkinson's Disease Prediction Using Machine Learning Models with Improved Feature Reduction Techniques
Journal of Data Science and Intelligent Systems(2023)
摘要
As a chronic and life-threatening disease, Parkinson’s disease (PD) causes people to become rigid and inactive and have shaky voices. There is an argument that current PD detection techniques are ineffective due to their high latency and low accuracy. To enhance the accuracy of PD identification, voice recordings were used as biomarkers in conjunction with the synthetic minority oversampling technique (SMOTE). Three machine learning (ML) models namely support vector machine (SVM), K-nearest neighbors (KNN), and random forest (RF) were adopted to calculate the prediction accuracy. By applying an unsupervised dimensional reduction method, the generated model eliminates redundant data and speeds up training and testing. Model performance is estimated with three parameters, including accuracy, F1 score, and area under the curve (AUC) values. Experimental outcomes suggested that the RF model outperforms other models with 97.4% of classification accuracy. This type of research aims to analyze patient voice recordings to determine the disease severity.
更多查看译文
关键词
parkinson,disease prediction,machine learning models,machine learning,voice
AI 理解论文
溯源树
样例
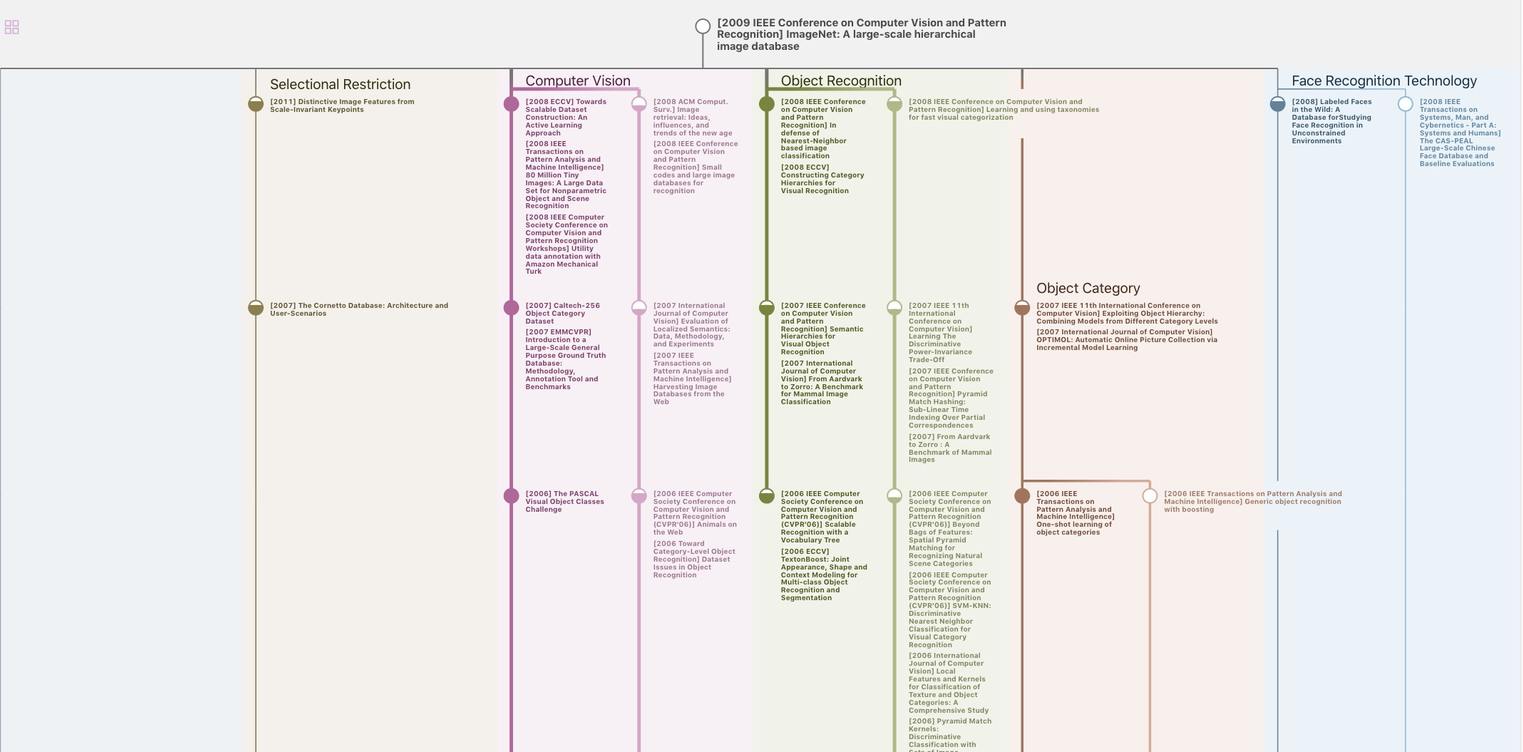
生成溯源树,研究论文发展脉络
Chat Paper
正在生成论文摘要