Pd34-06 machine learning prediction of symptomatic kidney stone recurrence using 24-hour urine data and electronic health record derived features
The Journal of Urology(2023)
摘要
You have accessJournal of UrologyCME1 Apr 2023PD34-06 MACHINE LEARNING PREDICTION OF SYMPTOMATIC KIDNEY STONE RECURRENCE USING 24-HOUR URINE DATA AND ELECTRONIC HEALTH RECORD DERIVED FEATURES Patrick Doyle, Wu Gong, Ryan Hsi, and Nicholas Kavoussi Patrick DoylePatrick Doyle More articles by this author , Wu GongWu Gong More articles by this author , Ryan HsiRyan Hsi More articles by this author , and Nicholas KavoussiNicholas Kavoussi More articles by this author View All Author Informationhttps://doi.org/10.1097/JU.0000000000003327.06AboutPDF ToolsAdd to favoritesDownload CitationsTrack CitationsPermissionsReprints ShareFacebookLinked InTwitterEmail Abstract INTRODUCTION AND OBJECTIVE: While 24-hour (24H) urine studies offer insight into the metabolic profile of recurrent stone formers, their use towards predicting stone recurrence events is limited. We sought to assess the prediction of symptomatic kidney stone recurrence episodes using machine learning models. METHODS: We trained three separate machine learning (ML) models (least absolute shrinkage and selection operator regression [LASSO], random forest [RF], and gradient boosted decision tree [XGBoost] to predict symptomatic kidney stone recurrences from electronic health-record (EHR) derived features and 24H urine data. ML models were compared to logistic regression [LR]. We evaluated all patients with an index stone treatment at our institution and a 24H urine test performed (N=1231). A manual, retrospective review was performed to evaluate for a symptomatic stone event, defined as pain, acute kidney injury or recurrent infections attributed to a kidney stone identified in the clinic or the emergency department, or for any stone requiring surgical treatment. We evaluated performance using area under the receiver operating curve (AUC-ROC) and identified predictors for each model. RESULTS: The 2- and 5- year symptomatic stone recurrence rates were 25% and 31%, respectively. The LASSO model (Figure 1) performed best for symptomatic stone recurrence prediction (2-yr AUC: 0.62, 5-yr AUC: 0.63). The other models demonstrated modest overall performance at 2- and 5-years: LR (0.585, 0.618), RF (0.570, 0.608), and XGBoost (0.580, 0.621). Top prioritized features among all models included age, diabetic status, stone composition and urine pH. Additionally, the LASSO model prioritized BMI and history of gout for prediction. CONCLUSIONS: Throughout our cohorts, ML models demonstrated comparable results to that of LR, with the LASSO model outperforming all other models. Further model optimization should incorporate 24H urine testing and EHR-derived features. Source of Funding: Vanderbilt CTSA Grant UL1TR002243 © 2023 by American Urological Association Education and Research, Inc.FiguresReferencesRelatedDetails Volume 209Issue Supplement 4April 2023Page: e922 Advertisement Copyright & Permissions© 2023 by American Urological Association Education and Research, Inc.MetricsAuthor Information Patrick Doyle More articles by this author Wu Gong More articles by this author Ryan Hsi More articles by this author Nicholas Kavoussi More articles by this author Expand All Advertisement PDF downloadLoading ...
更多查看译文
关键词
symptomatic kidney stone recurrence,machine learning prediction,machine learning,urine
AI 理解论文
溯源树
样例
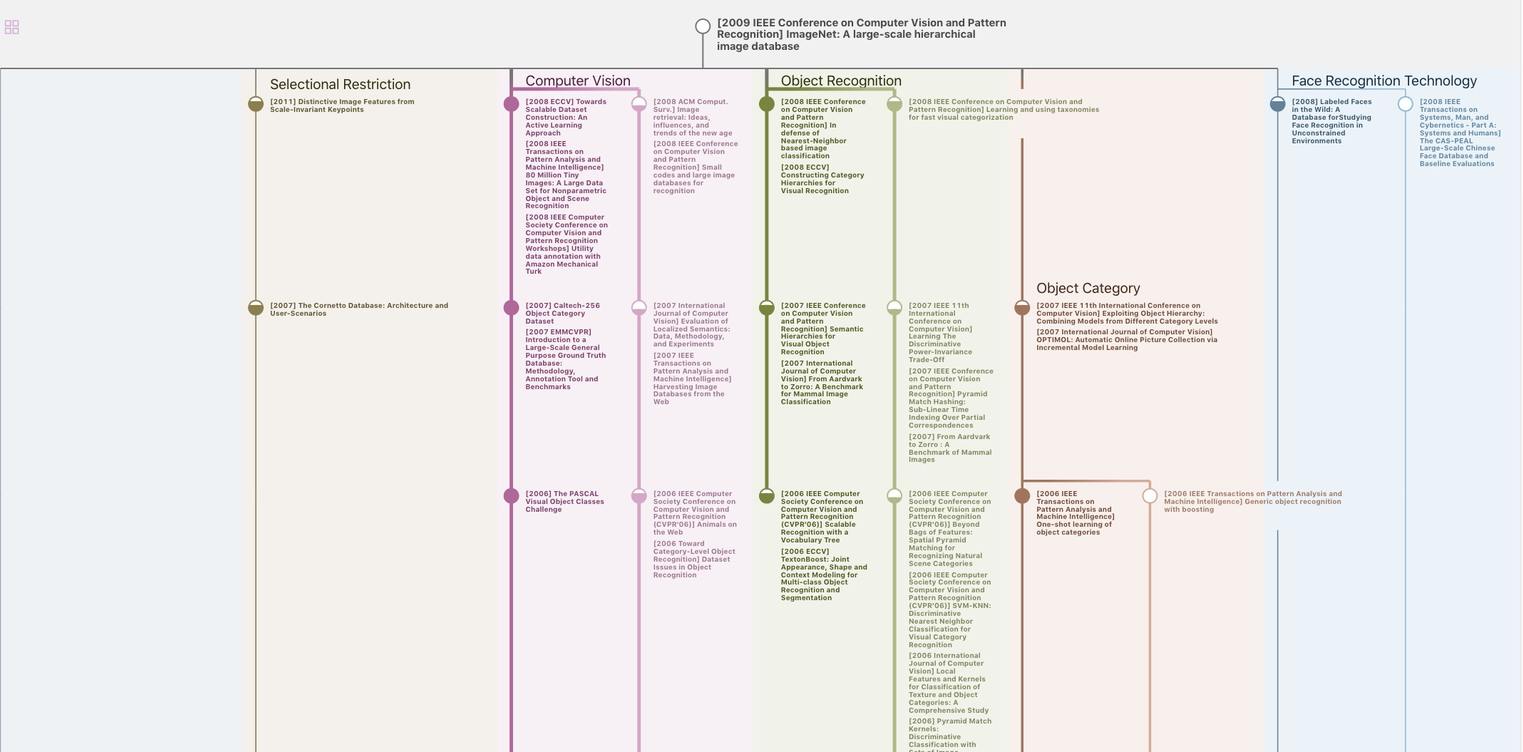
生成溯源树,研究论文发展脉络
Chat Paper
正在生成论文摘要