Auxiliary Functions as Koopman Observables: Data-Driven Analysis of Dynamical Systems via Polynomial Optimization
Journal of Nonlinear Science(2024)
摘要
We present a flexible data-driven method for dynamical system analysis that does not require explicit model discovery. The method is rooted in well-established techniques for approximating the Koopman operator from data and is implemented as a semidefinite program that can be solved numerically. Furthermore, the method is agnostic of whether data are generated through a deterministic or stochastic process, so its implementation requires no prior adjustments by the user to accommodate these different scenarios. Rigorous convergence results justify the applicability of the method, while also extending and uniting similar results from across the literature. Examples on discovering Lyapunov functions, performing ergodic optimization, and bounding extrema over attractors for both deterministic and stochastic dynamics exemplify these convergence results and demonstrate the performance of the method.
更多查看译文
关键词
Koopman operator,Auxiliary function,Dynamic mode decomposition,Semidefinite programming,Time average,Lyapunov function
AI 理解论文
溯源树
样例
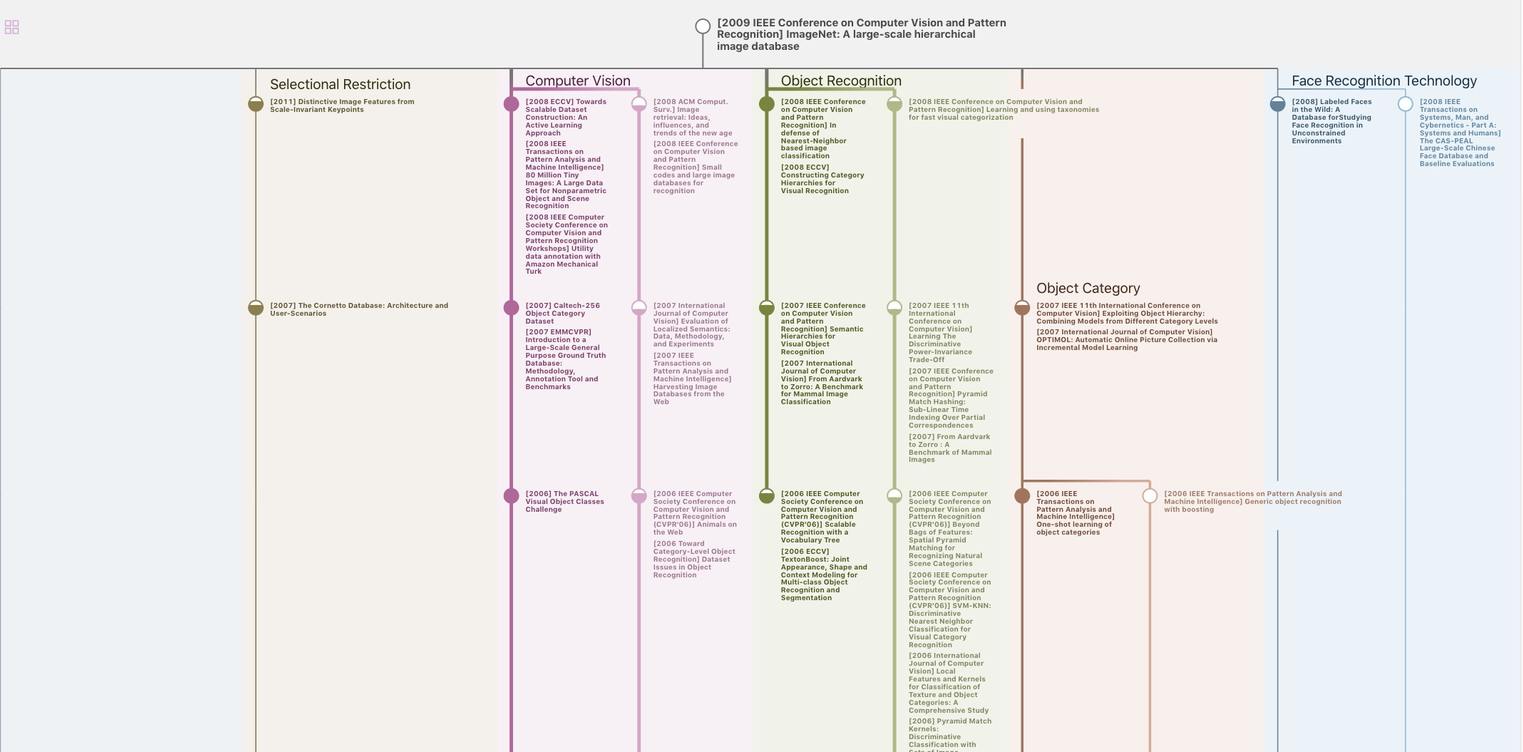
生成溯源树,研究论文发展脉络
Chat Paper
正在生成论文摘要