Mixture Proportion Estimation and PU Learning: A Modern Approach
arXiv (Cornell University)(2021)
摘要
Given only positive examples and unlabeled examples (from both positive and negative classes), we might hope nevertheless to estimate an accurate positive-versus-negative classifier. Formally, this task is broken down into two subtasks: (i) Mixture Proportion Estimation (MPE) -- determining the fraction of positive examples in the unlabeled data; and (ii) PU-learning -- given such an estimate, learning the desired positive-versus-negative classifier. Unfortunately, classical methods for both problems break down in high-dimensional settings. Meanwhile, recently proposed heuristics lack theoretical coherence and depend precariously on hyperparameter tuning. In this paper, we propose two simple techniques: Best Bin Estimation (BBE) (for MPE); and Conditional Value Ignoring Risk (CVIR), a simple objective for PU-learning. Both methods dominate previous approaches empirically, and for BBE, we establish formal guarantees that hold whenever we can train a model to cleanly separate out a small subset of positive examples. Our final algorithm (TED)$^n$, alternates between the two procedures, significantly improving both our mixture proportion estimator and classifier
更多查看译文
关键词
mixture proportion estimation,pu learning
AI 理解论文
溯源树
样例
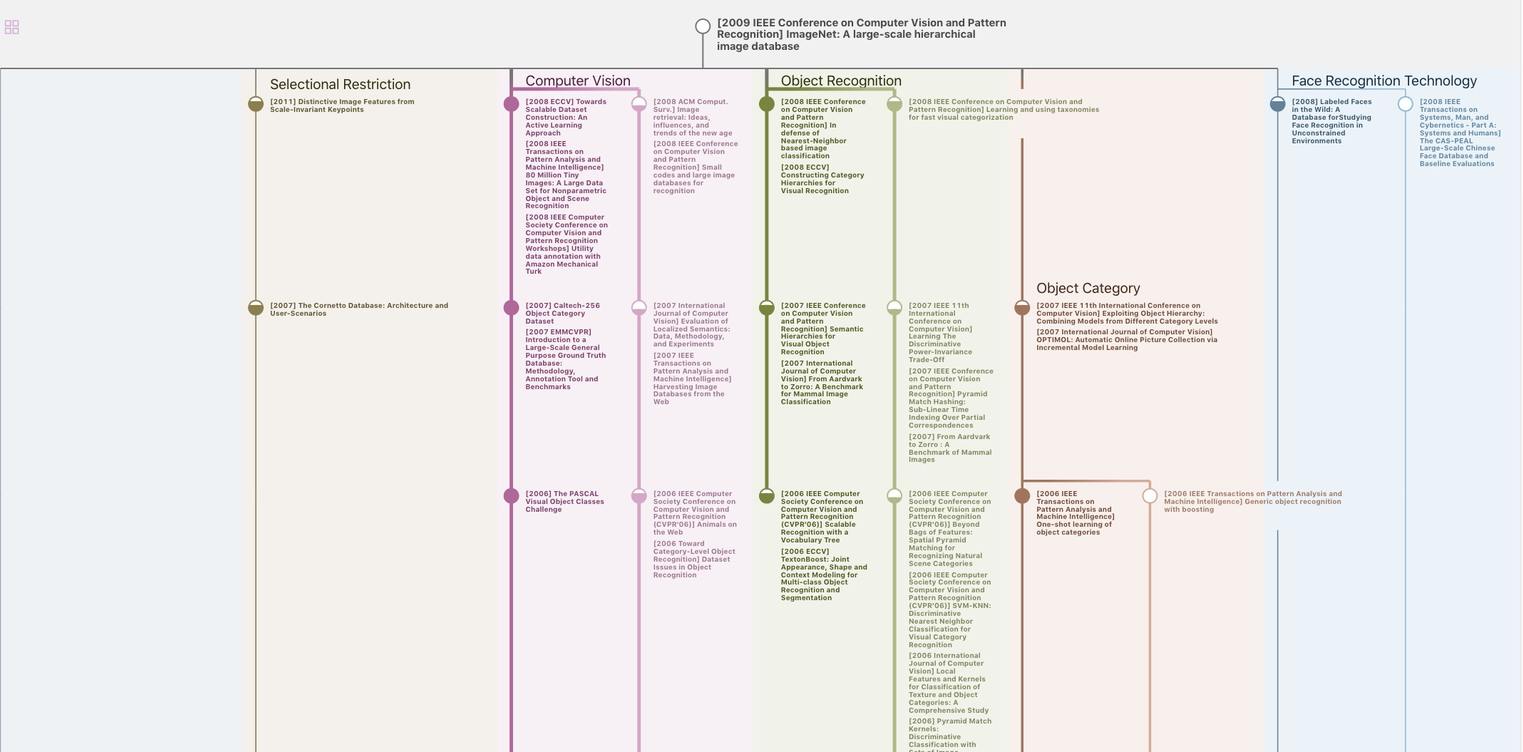
生成溯源树,研究论文发展脉络
Chat Paper
正在生成论文摘要