Statistical matching and subclassification with a continuous dose: characterization, algorithm, and application to a health outcomes study
arXiv (Cornell University)(2020)
摘要
Subclassification and matching are often used in empirical studies to adjust for observed covariates; however, they are largely restricted to relatively simple study designs with a binary treatment and less developed for designs with a continuous exposure. Matching with exposure doses is particularly useful in instrumental variable designs and in understanding the dose-response relationships. In this article, we propose two criteria for optimal subclassification based on subclass homogeneity in the context of having a continuous exposure dose, and propose an efficient polynomial-time algorithm that is guaranteed to find an optimal subclassification with respect to one criterion and serves as a 2-approximation algorithm for the other criterion. We discuss how to incorporate dose and use appropriate penalties to control the number of subclasses in the design. Via extensive simulations, we systematically compare our proposed design to optimal non-bipartite pair matching, and demonstrate that combining our proposed subclassification scheme with regression adjustment helps reduce model dependence for parametric causal inference with a continuous dose. We apply the new design and associated randomization-based inferential procedure to study the effect of transesophageal echocardiography (TEE) monitoring during coronary artery bypass graft (CABG) surgery on patients' post-surgery clinical outcomes using Medicare and Medicaid claims data, and find evidence that TEE monitoring lowers patients' all-cause $30$-day mortality rate.
更多查看译文
关键词
statistical matching,continuous dose
AI 理解论文
溯源树
样例
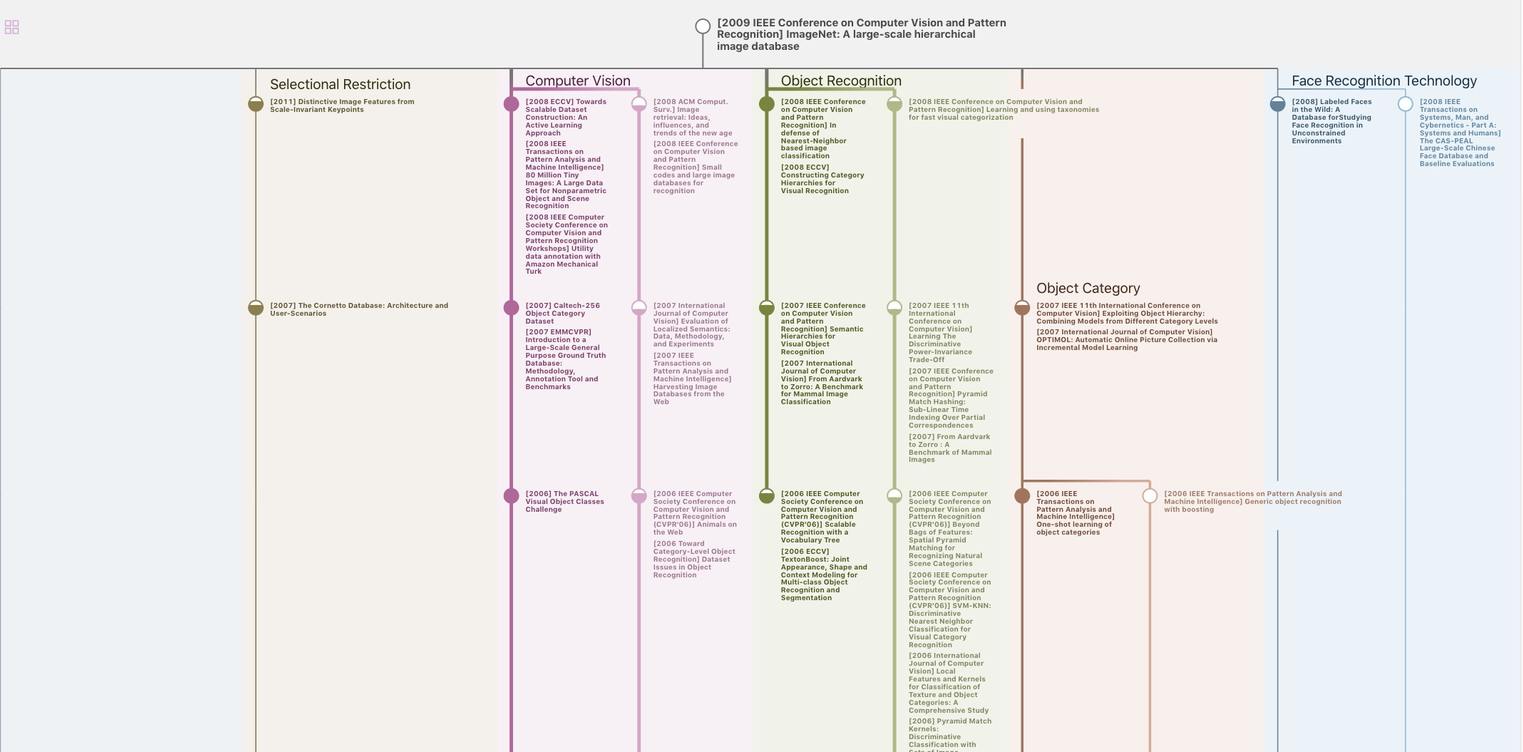
生成溯源树,研究论文发展脉络
Chat Paper
正在生成论文摘要