Monte Carlo PINNs: deep learning approach for forward and inverse problems involving high dimensional fractional partial differential equations
arXiv (Cornell University)(2022)
摘要
We introduce a sampling based machine learning approach, Monte Carlo physics informed neural networks (MC-PINNs), for solving forward and inverse fractional partial differential equations (FPDEs). As a generalization of physics informed neural networks (PINNs), our method relies on deep neural network surrogates in addition to a stochastic approximation strategy for computing the fractional derivatives of the DNN outputs. A key ingredient in our MC-PINNs is to construct an unbiased estimation of the physical soft constraints in the loss function. Our directly sampling approach can yield less overall computational cost compared to fPINNs proposed in \cite{pang2019fpinns} and thus provide an opportunity for solving high dimensional fractional PDEs. We validate the performance of MC-PINNs method via several examples that include high dimensional integral fractional Laplacian equations, parametric identification of time-space fractional PDEs, and fractional diffusion equation with random inputs. The results show that MC-PINNs is flexible and promising to tackle high-dimensional FPDEs.
更多查看译文
关键词
monte carlo pinns,deep learning,differential equations,inverse
AI 理解论文
溯源树
样例
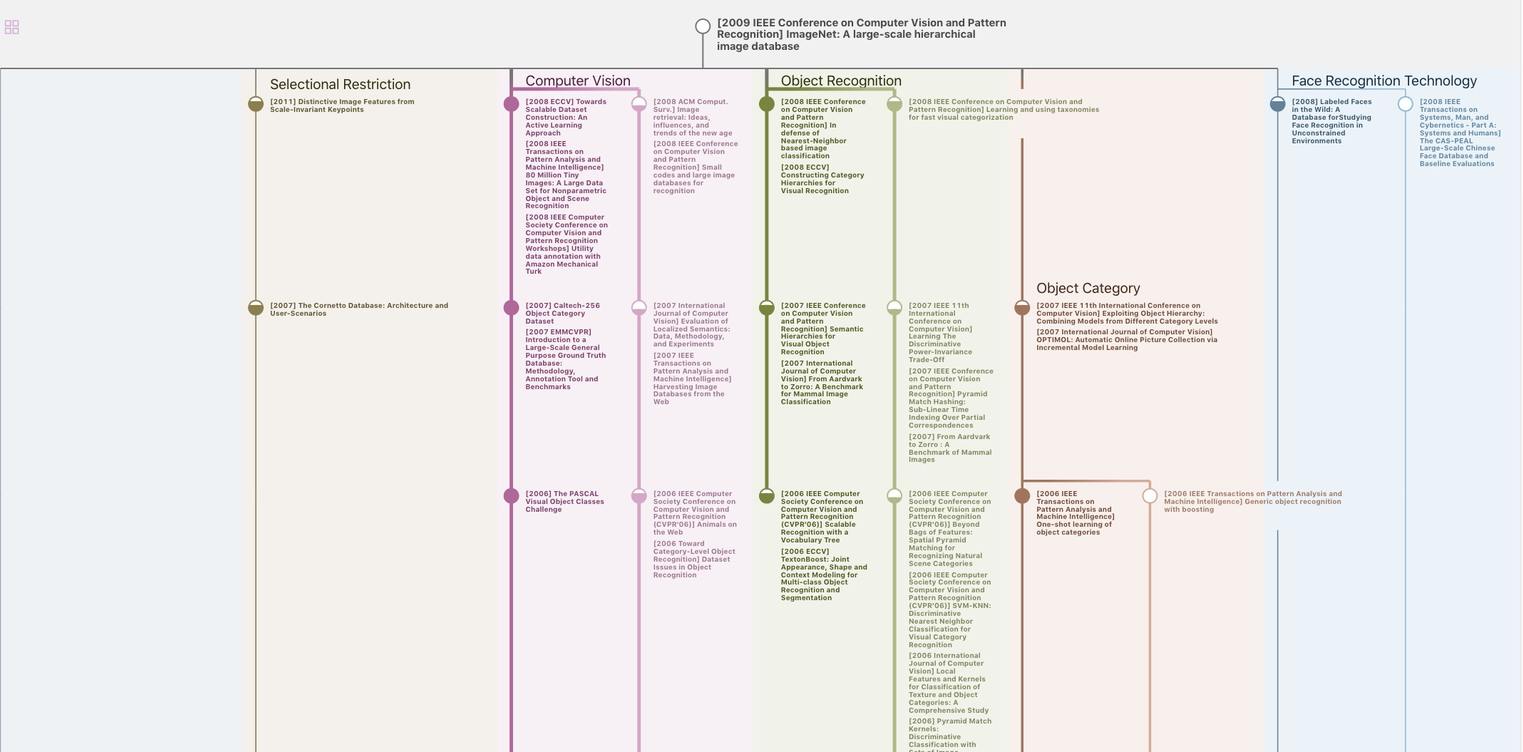
生成溯源树,研究论文发展脉络
Chat Paper
正在生成论文摘要