A novel framework for single-cell Hi-C clustering based on graph-convolution-based imputation and two-phase-based feature extraction
bioRxiv (Cold Spring Harbor Laboratory)(2021)
摘要
ABSTRACT The three-dimensional genome structure plays a key role in cellular function and gene regulation. Singlecell Hi-C technology can capture genome structure information at the cell level, which provides the opportunity to study how genome structure varies among different cell types. However, few methods are well designed for single-cell Hi-C clustering, because of high sparsity, noise and heterogeneity of single-cell Hi-C data. In this manuscript, we propose a novel framework, named ScHiC-Rep, for singlecell Hi-C data representation and clustering. ScHiC-Rep mainly contains two parts: data imputation and feature extraction. In the imputation part, a novel imputation workflow is proposed, including graph convolution-based, random walk with restart-based and genomic neighbor-based imputation. In the feature extraction part, a two-phase feature extraction method is proposed, including linear phase for chromosome level and non-linear phase for cell level feature extraction. The evaluation results show that the proposed framework outperforms existing state-of-the-art approaches on both human and mouse datasets.
更多查看译文
关键词
single-cell single-cell,feature extraction,imputation,graph-convolution-based,two-phase-based
AI 理解论文
溯源树
样例
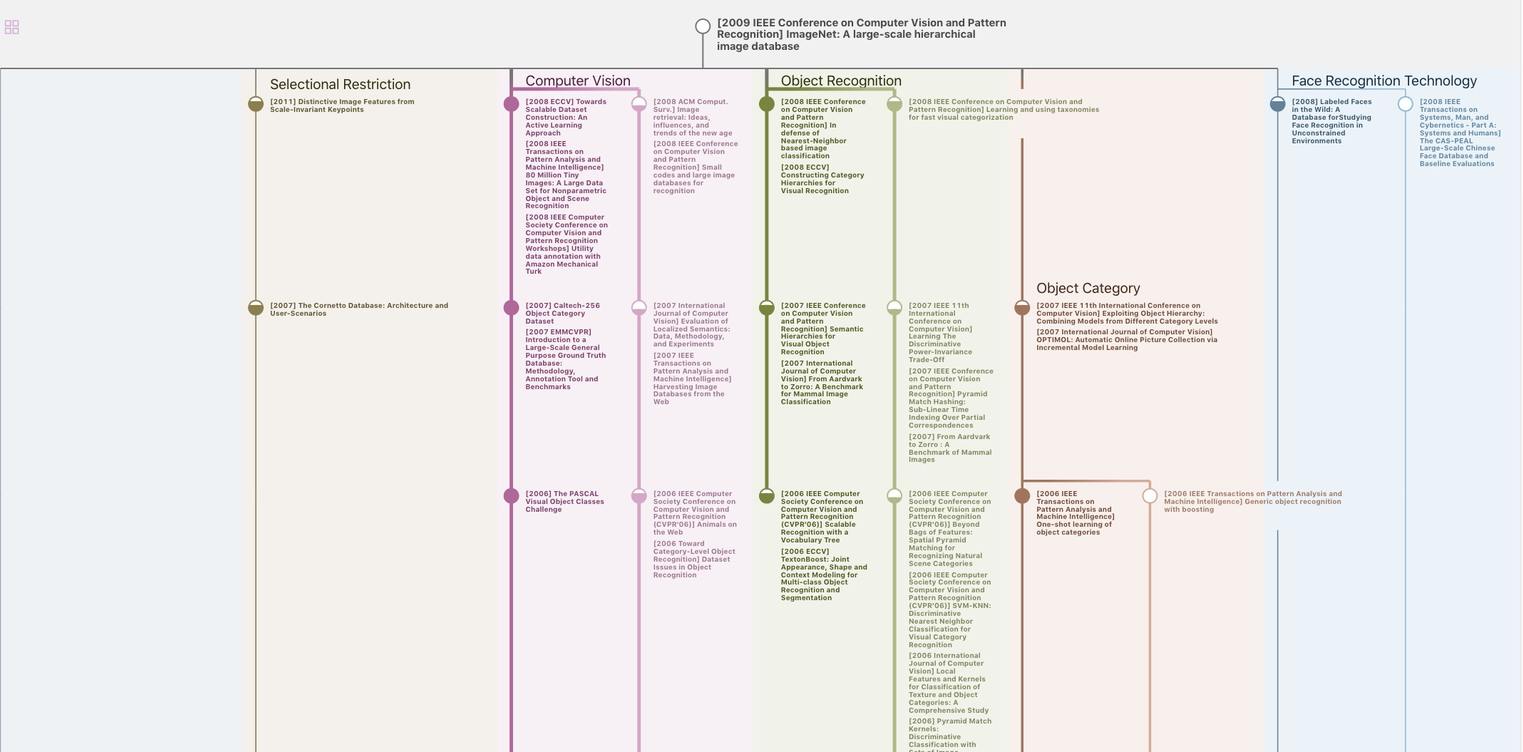
生成溯源树,研究论文发展脉络
Chat Paper
正在生成论文摘要