Materials cartography: A forward-looking perspective on materials representation and devising better maps
APL Machine Learning(2023)
摘要
Machine learning (ML) is gaining popularity as a tool for materials scientists to accelerate computation, automate data analysis, and predict materials properties. The representation of input material features is critical to the accuracy, interpretability, and generalizability of data-driven models for scientific research. In this Perspective, we discuss a few central challenges faced by ML practitioners in developing meaningful representations, including handling the complexity of real-world industry-relevant materials, combining theory and experimental data sources, and describing scientific phenomena across timescales and length scales. We present several promising directions for future research: devising representations of varied experimental conditions and observations, the need to find ways to integrate machine learning into laboratory practices, and making multi-scale informatics toolkits to bridge the gaps between atoms, materials, and devices.
更多查看译文
关键词
materials representation,better maps,forward-looking
AI 理解论文
溯源树
样例
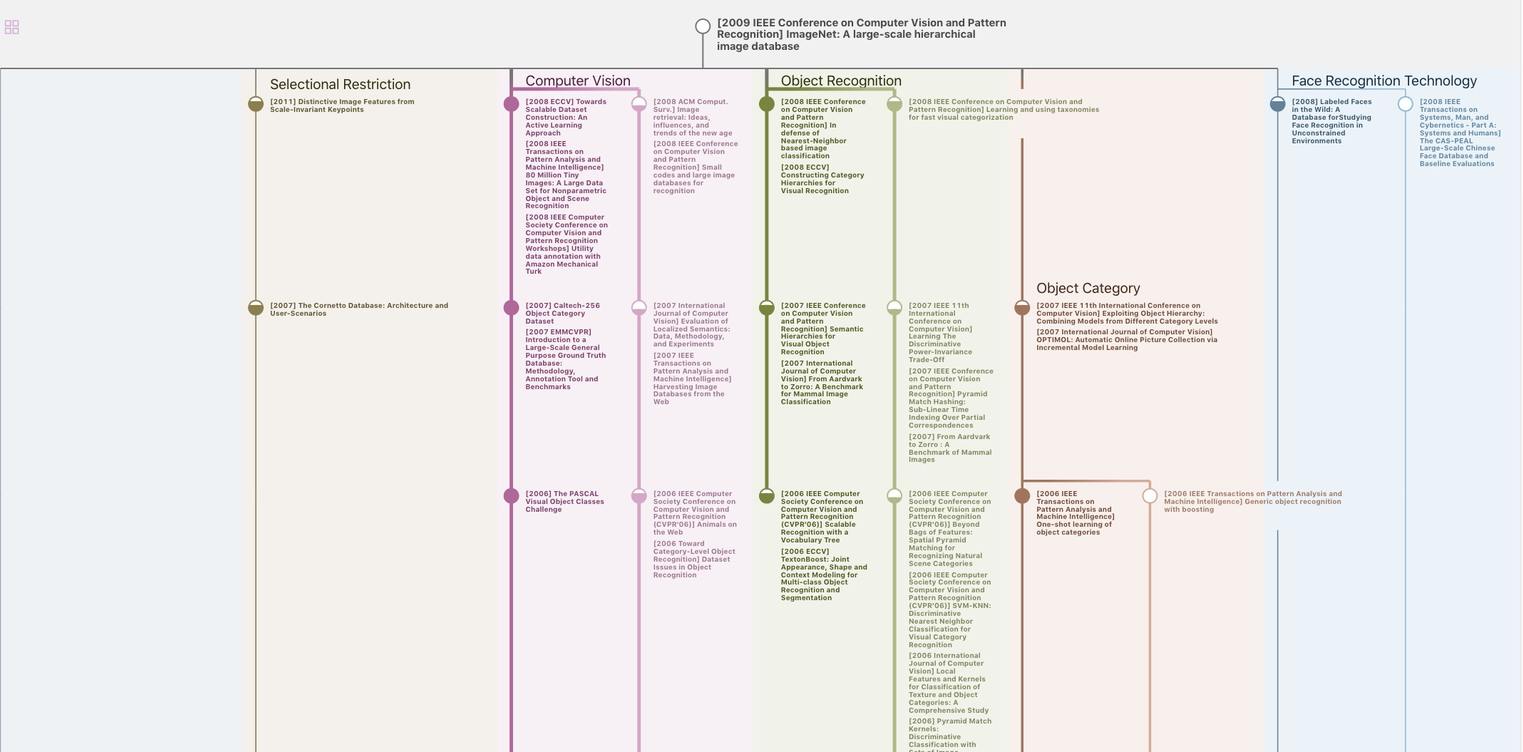
生成溯源树,研究论文发展脉络
Chat Paper
正在生成论文摘要