A new multi-source remote sensing image sample dataset with high resolution for flood area extraction: GF-FloodNet
International Journal of Digital Earth(2023)
摘要
Deep learning algorithms show good prospects for remote sensing flood monitoring. They mostly rely on huge amounts of labeled data. However, there is a lack of available labeled data in actual needs. In this paper, we propose a high-resolution multi-source remote sensing dataset for flood area extraction: GF-FloodNet. GF-FloodNet contains 13388 samples from Gaofen-3 (GF-3) and Gaofen-2 (GF-2) images. We use a multi-level sample selection and interactive annotation strategy based on active learning to construct it. Compare with other flood-related datasets, GF-FloodNet not only has a spatial resolution of up to 1.5 m and provides pixel-level labels, but also consists of multi-source remote sensing data. We thoroughly validate and evaluate the dataset using several deep learning models, including quantitative analysis, qualitative analysis, and validation on large-scale remote sensing data in real scenes. Experimental results reveal that GF-FloodNet has significant advantages by multi-source data. It can support different deep learning models for training to extract flood areas. There should be a potential optimal boundary for model training in any deep learning dataset. The boundary seems close to 4824 samples in GF-FloodNet. We provide GF-FloodNet at https://www.kaggle.com/datasets/pengliuair/gf-floodnet and https://pan.baidu.com/s/1vdUCGNAfFwG5UjZ9RLLFMQ?pwd=8v6o.
更多查看译文
关键词
flood area extraction,remote sensing,image sample,multi-source,gf-floodnet
AI 理解论文
溯源树
样例
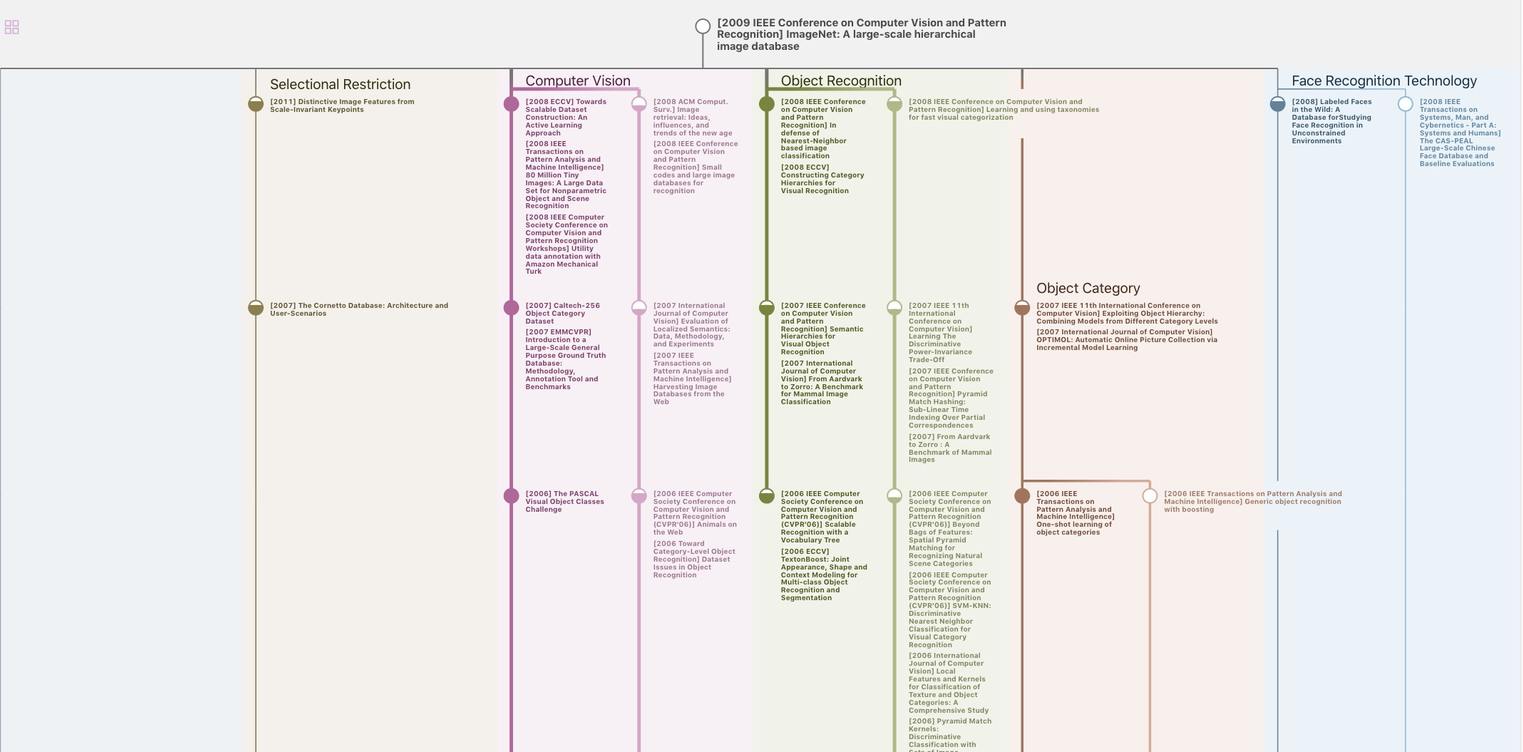
生成溯源树,研究论文发展脉络
Chat Paper
正在生成论文摘要