Utilization of deep learning to evaluate the measurement results of forced oscillation technique
Research Square (Research Square)(2023)
摘要
Abstract No reference values have been established for MostGraph, which measures total respiratory resistance and reactance using the forced oscillation technique, making it difficult to interpret the results. This study aimed to establish a reference range for MostGraph measurements and examine its usefulness in discriminating participants with asthma from controls (participants without any respiratory diseases). The study also aimed to investigate the effectiveness of deep learning in discriminating between the two aforementioned groups. To establish reference ranges, the MostGraph measurements of healthy controls (n = 215) were power-transformed to distribute the data more normally. After inverse transformation, the mean ± standard deviation ⋅ 2 of the transformed values were used to establish the reference ranges. The number of measured items from the reference ranges was evaluated to discriminate patients with asthma (n = 941) from controls. Additionally, MostGraph measurements were evaluated using deep learning. Although reference ranges were established, patients with asthma could not be discriminated from controls. However, with deep learning, we could discriminate between the two groups with 78% accuracy. Therefore, deep learning, which considers multiple measurements as a whole, was found to be more effective in interpreting MostGraph measurement results compared to the reference range, which considers each result individually.
更多查看译文
关键词
deep learning,measurement results
AI 理解论文
溯源树
样例
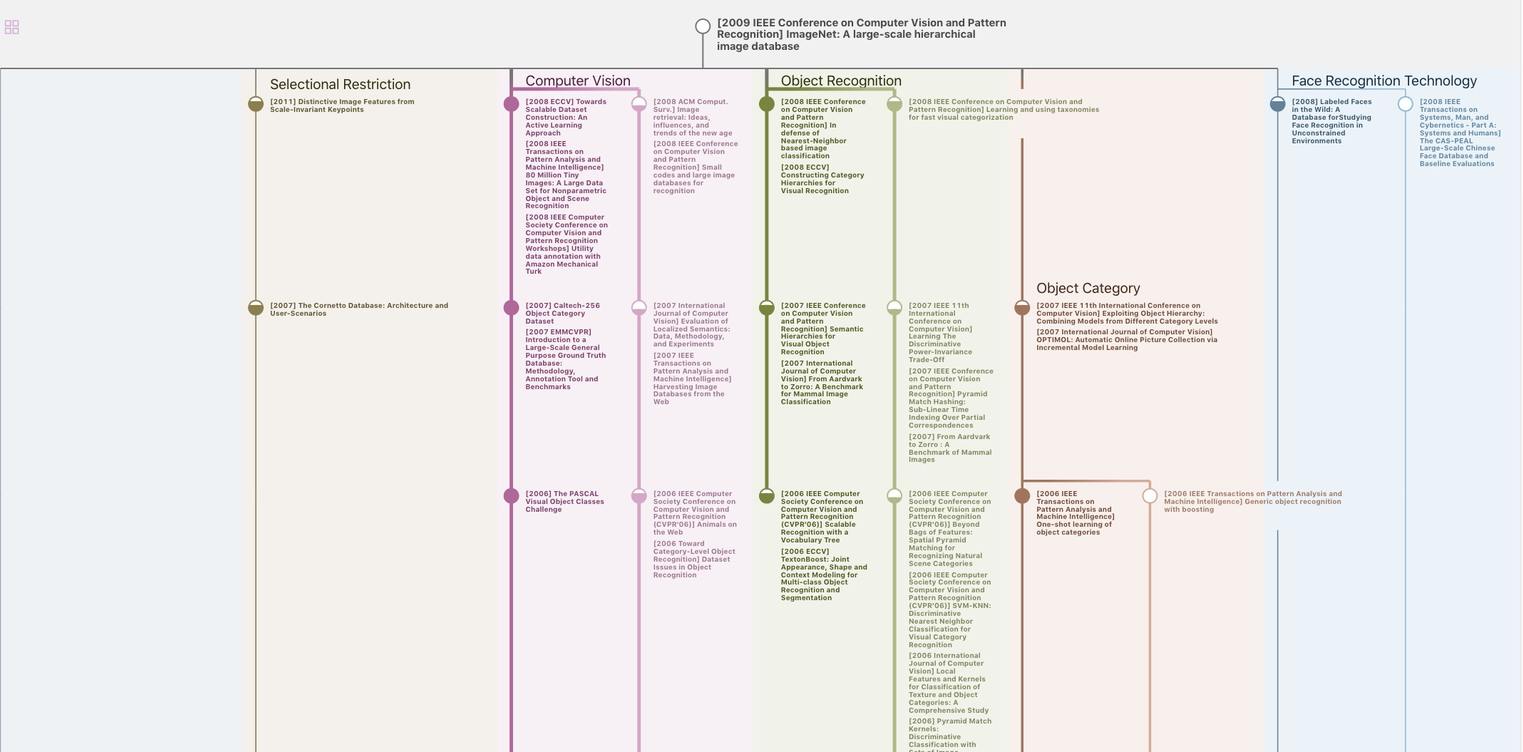
生成溯源树,研究论文发展脉络
Chat Paper
正在生成论文摘要