Optimal Transport-Based Volumetric Shape Clustering
Research Square (Research Square)(2023)
摘要
Abstract Clustering of data points in $n$-dimensional Euclidean space, i.~e. assigning each data point to exactly one group (cluster) in order to detect previously unseen relations within the data set, has become a standard task for (unsupervised) machine learning. In this paper, this concept is generalized to shape data consisting of three-dimensional volumetric objects. As the underlying distance on the space of such objects, the optimal transport-based Wasserstein distance is considered and different variants of such a clustering approach are presented and compared. To counteract an over-smoothing of the cluster center volumes a variational autoencoder representing these cluster centers is incorporated. Numerical experiments for three distinct, volumetric data sets are presented to validate the performance of the proposed methods.
更多查看译文
关键词
volumetric shape clustering,transport-based
AI 理解论文
溯源树
样例
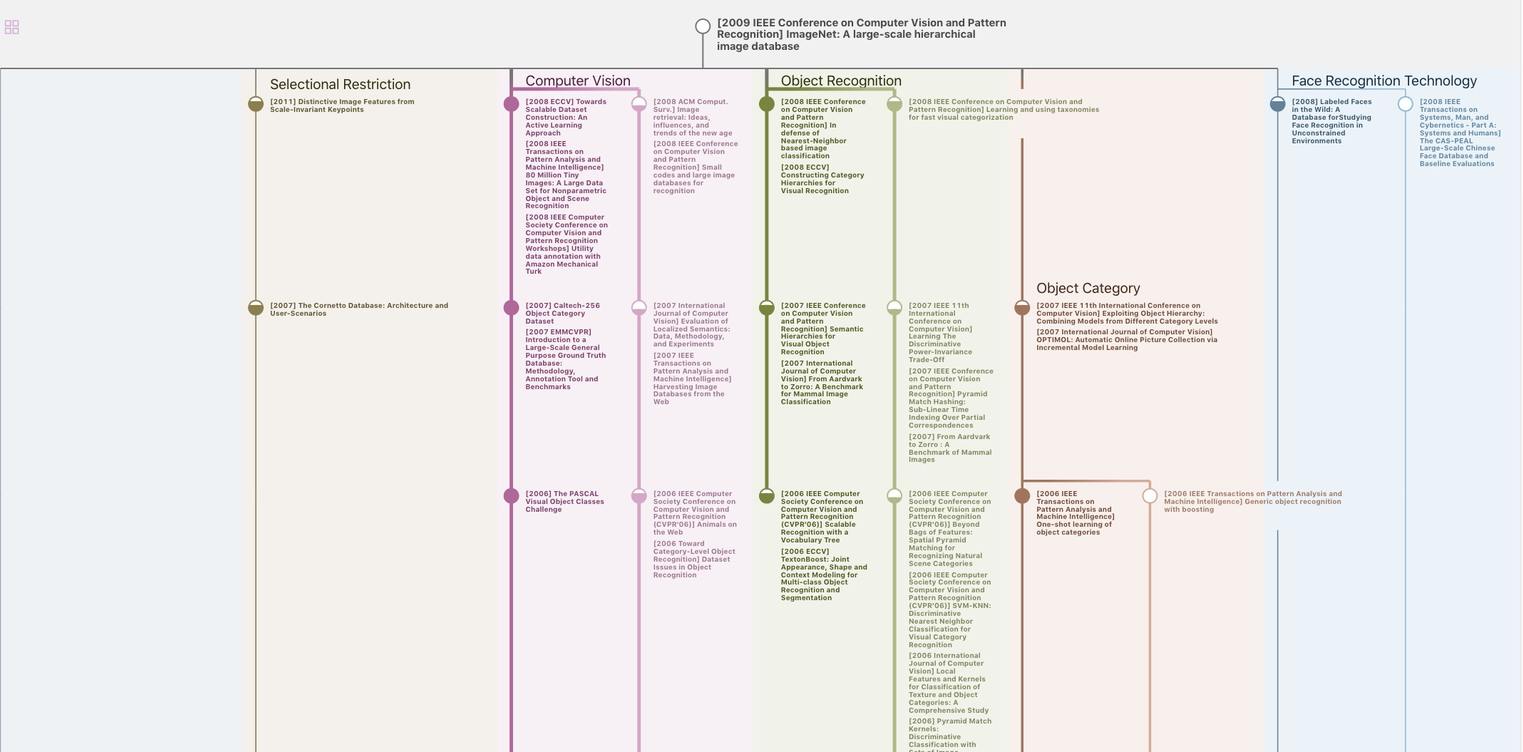
生成溯源树,研究论文发展脉络
Chat Paper
正在生成论文摘要