GraphMM: Graph-Based Vehicular Map Matching by Leveraging Trajectory and Road Correlations
IEEE TRANSACTIONS ON KNOWLEDGE AND DATA ENGINEERING(2024)
摘要
Map matching of sparse vehicle trajectories is a fundamental problem in location-based services, such as traffic flow analysis and vehicle routing. Existing literature mainly relies on sequence-to-sequence (Seq2Seq) models to capture the intra-trajectory correlation of an input trajectory and to sequentially predict the matched road segments. Due to the limited expressive capability of sequential models, these methods fall short of extracting inter-trajectory and trajectory-road correlations as well as correlation between road segments. We present GraphMM , a graph-based approach that explicitly utilizes all aforementioned correlations. Our model exploits the graph nature of map matching and incorporates graph neural networks and conditional models to leverage both road and trajectory graph topology, while manages to align road segments and trajectories in latent space. We formally analyze the expressive power of our model in capturing various correlations and propose efficient algorithms for model training and inference. In particular, our optimization techniques dramatically reduce the computational complexity, making our model feasible on datasets with thousands of road segments. Extensive experiments show that our model significantly enhances prediction accuracy, while improving training and inference efficiency by up to an order of magnitude over both the industrial implementation of the hidden Markov model and state-of-the-art Seq2Seq-based methods.
更多查看译文
关键词
Conditional model,graph neural network,inductive capability,map matching,trajectory and road correlations
AI 理解论文
溯源树
样例
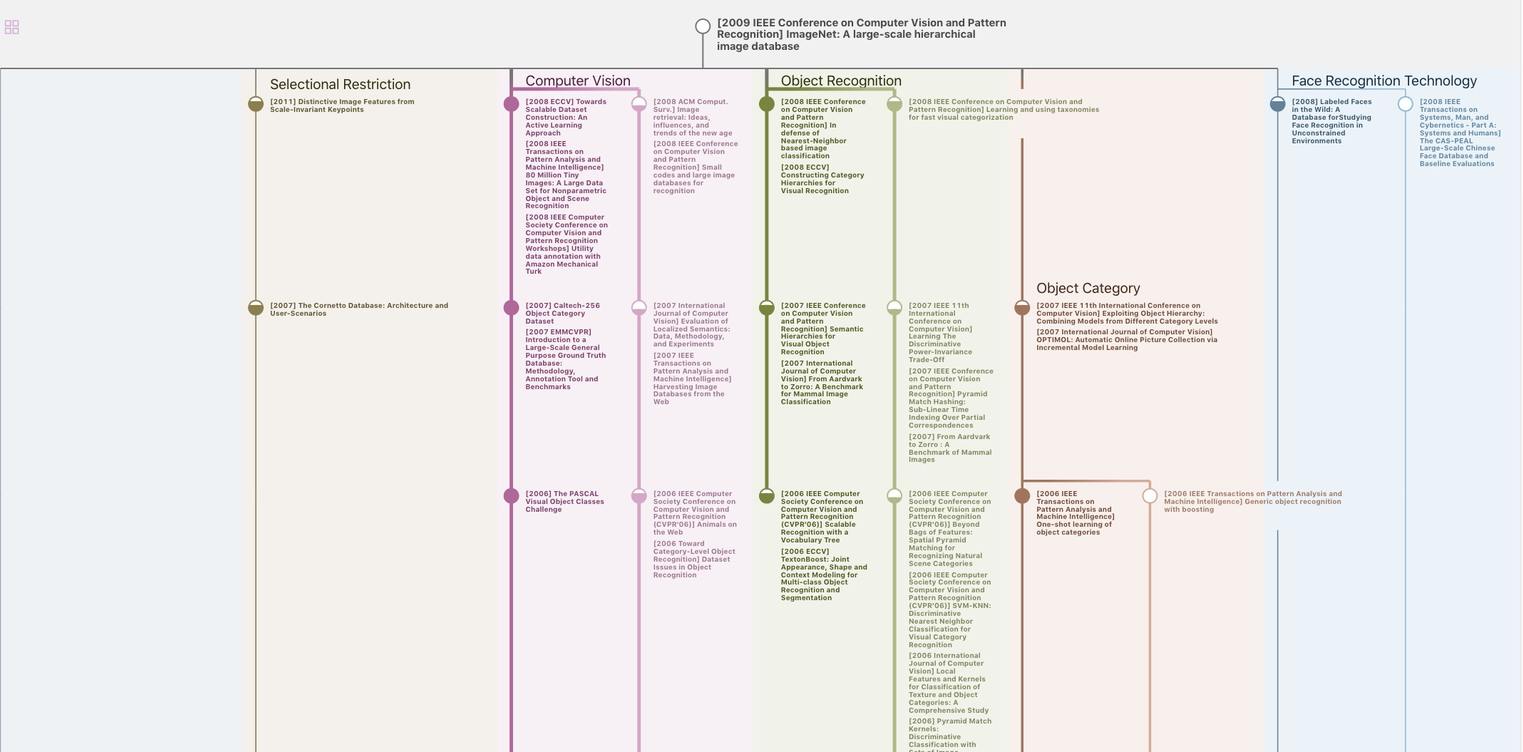
生成溯源树,研究论文发展脉络
Chat Paper
正在生成论文摘要