Stock Closing Price Prediction of Indian Tech Companies using Deep Neural Networks
Research Square (Research Square)(2023)
摘要
Abstract In today’s economic world forecast and study of stock market data have got a vital role but, stock price forecasting has been hampered by its nonlinearity and high volatility of financial time series, to maximize the profits and reduce the risk involved the investors are eager to find different ways to forecast upcoming trends. It’s a challenging task to crack stock portfolio allocation in such a suitable way to invest in selected stocks that efficiently minimize the risk involved while capitalizing on profit. The effects of rival stocks in the stock market and the impact of mutation points on the precision of target stock predictions are rarely considered as market sentiment by the current prediction models. To validate which deep learning algorithm is best suited for stock market prediction analysis, we have espoused four deep learning algorithms namely Long Short-Term Memory (LSTM), Autoregressive Integrated Memory (ARIMA), Bi-directional Long Short-Term Memory (Bi-LSTM), and Gate Recurrent Units (GRU). On the basis of these mentioned evaluation parameters i.e., Root Mean Square Error (RMSE), Mean Square Error (MSE), and Mean Absolute Percentage Error (MAPE) we have done the accuracy tests of the listed deep learning algorithms with evaluative stock prediction graph. Results have shown Bi-LSTM to perform exceptionally well for time series analysis. Regarding LSTM and GRU, the results have more or less stayed the same. While ARIMA gave an un-stable performance thus rendering its usefulness for our analysis.
更多查看译文
关键词
stock closing price prediction,deep neural networks,indian tech companies
AI 理解论文
溯源树
样例
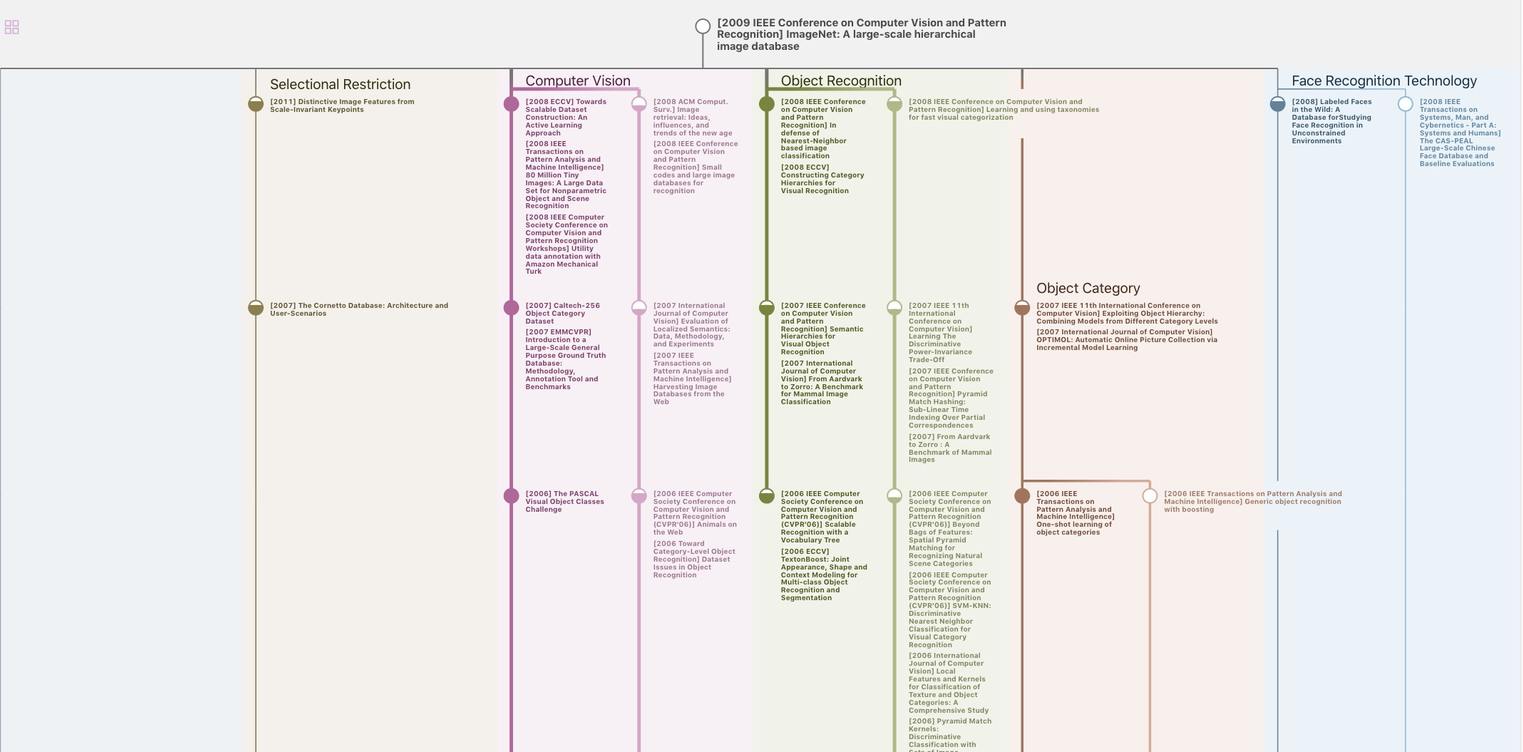
生成溯源树,研究论文发展脉络
Chat Paper
正在生成论文摘要