Digital Trial Solutions eScreening: Software to rank patients by their predicted clinical trial eligibility using real-world data and machine learning
Journal of Clinical Oncology(2023)
摘要
e13587 Background: The recruitment process for clinical trials is time-consuming and labor-intensive. Electronic screening tools aim to facilitate patient recruitment by focusing the recruiters’ efforts on patients most likely to be eligible. These tools are required to produce useful estimates of patient eligibility despite large amounts of missingness in Real World Data (RWD). Digital Trial Solutions (DTS) eScreening—unlike other proposed tools that use only top-level Inclusion/Exclusion (I/E) criteria—queries for all computable criteria and imputes missing data using Machine Learning (ML). Our ML models use the ConcertAI data from DTS clinical sites. Methods: DTS eScreening provides an eligibility score for each patient in a clinical trial cohort based on how many I/E criteria are fulfilled, weighted by criteria relevance as assessed by clinical experts. If information is missing in the patient record, DTS eScreening predicts the probability of passing the criterion using a binary classifier trained for each criterion. We evaluated classifier performance on a held-out test set. We evaluated the utility of DTS eScreening by collecting quantitative and qualitative data from users of DTS eScreening. Results: All evaluations were done on a multi-site clinical trial with 49 digitized criteria. The cohort was 10,156 patients with the appropriate cancer indication. Model performance: twenty-three criteria had complete data and so no ML models were built. To avoid biased model estimates, we avoided modeling ten criteria with greater than 99% missingness or 1000:1 imbalance. Seventeen LightGBM classifiers were built on the full cohort for criteria such as ECOG, stage, labs, and vitals. The model performance showed an average precision of >99% for most models, while the lowest precision was 83%. DTS eScreening utility: using our tool, clinical screeners reviewed 114 patients with the highest eligibility scores of the 10,156-patient cohort. Of the 114 patients, 38 were determined to meet all digitized eligibility criteria. The precision on reviewed patients was 33%, higher than other reports in the literature. Feedback from semi-structured interviews with 3 users indicated the tool was useful for reducing review time. A screener said: “It makes screening easier for me because the system already filters the most eligible patients at the top.”. Conclusions: DTS eScreening tool demonstrates the ability to conduct patient-trial matching using computable I/E criteria while addressing missingness in RWD. Positive initial feedback from users highlights improvements in the efficiency of patient recruitment in clinical trials. We believe our solution is scalable and can be applied beyond oncology. Future improvements include relaxing restrictions for modeling at low counts to include additional I/E criteria and incorporating unstructured data.
更多查看译文
关键词
clinical trial eligibility,software,machine learning,real-world
AI 理解论文
溯源树
样例
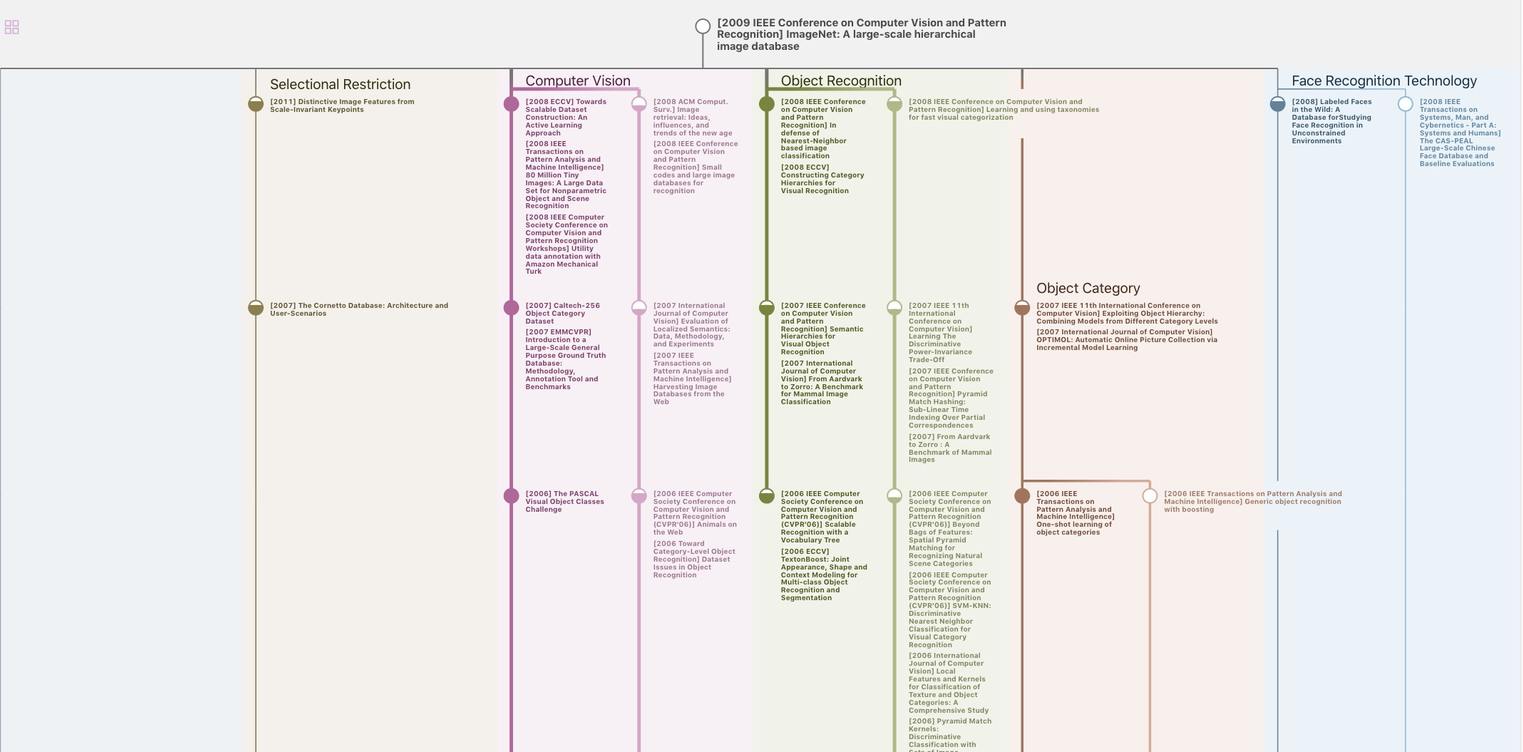
生成溯源树,研究论文发展脉络
Chat Paper
正在生成论文摘要