Evaluating the prediction performance of objective physical activity measures for incident Parkinson’s disease in the UK Biobank
Journal of Neurology(2023)
摘要
Background Parkinson’s disease (PD) is the fastest-growing neurological condition with over 10 million cases worldwide. While age and sex are known predictors of incident PD, there is a need to identify other predictors. This study compares the prediction performance of accelerometry-derived physical activity (PA) measures and traditional risk factors for incident PD in the UK Biobank. Methods The study population consisted of 92,352 UK Biobank participants without PD at baseline (43.8% male, median age 63 years with interquartile range 43–69). 245 participants were diagnosed with PD by April 1, 2021 (586,604 person-years of follow-up). The incident PD prediction performances of 10 traditional predictors and 8 objective PA measures were compared using single- and multi-variable Cox models. Prediction performance was assessed using a novel, stable statistic: the repeated cross-validated concordance (rcvC). Sensitivity analyses were conducted where PD cases diagnosed within the first six months, one year, and two years were deleted. Results Single-predictor Cox regression models indicated that all PA measures were statistically significant (p-values < 0.0001). The highest-performing individual predictors were total acceleration (TA) (rcvC = 0.813) among PA measures, and age (rcvC = 0.757) among traditional predictors. The two-step forward-selection process produced a model containing age, sex, and TA (rcvC = 0.851). Adding TA to the model increased the rcvC by 9.8% (p-value < 0.0001). Results were largely unchanged in sensitivity analyses. Conclusions Objective PA summaries have better single-predictor model performance than known risk factors and increase the prediction performance substantially when added to models with age and sex.
更多查看译文
关键词
Parkinson’s disease,Survival analysis,Accelerometry,Repeated cross-validation
AI 理解论文
溯源树
样例
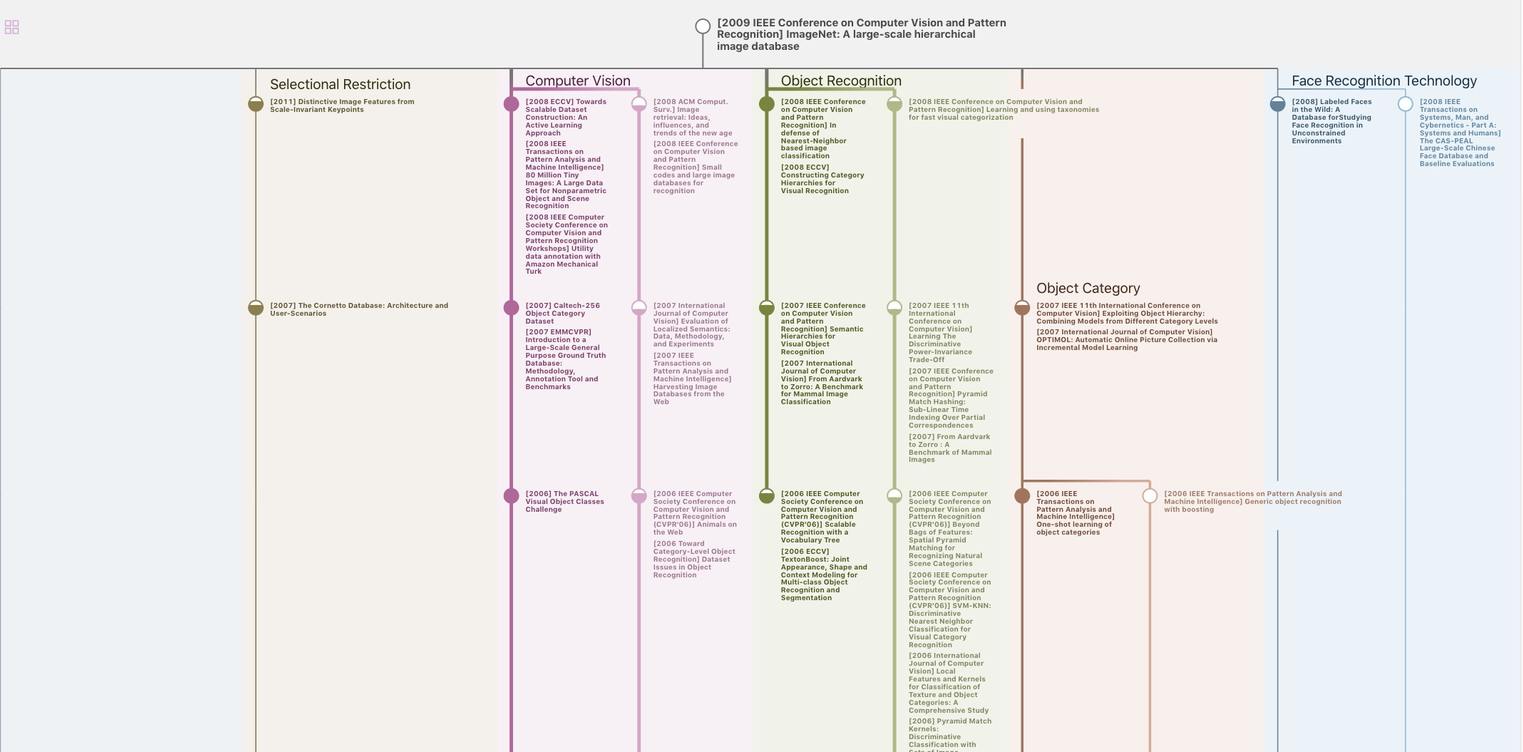
生成溯源树,研究论文发展脉络
Chat Paper
正在生成论文摘要