Simulating daily PM2.5 concentrations using wavelet analysis and artificial neural network with remote sensing and surface observation data.
Chemosphere(2023)
Abstract
Accurate PM2.5 concentrations predicting is critical for public health and wellness as well as pollution control. However, traditional methods are difficult to accurately predict PM2.5. An adaptive model coupled with artificial neural network (ANN) and wavelet analysis (WANN) is utilized to predict daily PM2.5 concentrations with remote sensing and surface observation data. The four evaluation metrics, namely Pearson correlation coefficient (R), mean absolute percentage error (MAPE), root mean square error (RMSE), and mean absolute error (MAE), are utilized to evaluate the performances of the artificial neural network (ANN) and WANN methods. From the predicting results, The WANN model has a higher R (R = 0.9990) during the testing period compared with R (R = 0.6844) based on the ANN model. Similarly, the WANN model has a lower MAPE (3.6988%), RMSE (1.0145 μg/m3), MAE (1.3864 μg/m3), compared with MAPE (80.0086%), RMSE (16.5838 μg/m3), MAE (12.2420 μg/m3) of the ANN. In addition, comparing the outcomes of the proposed WANN method with the ANN method, it was observed that the error during the training and verification period has decreased significantly. Furthermore, the statistical methods are used to analyze WANN and ANN, showing that WANN has higher training accuracy and better stability. Therefore, it is feasible to establish WANN to predict PM2.5 concentrations (1 day in advance) by using remote sensing and surface observation data.
MoreTranslated text
AI Read Science
Must-Reading Tree
Example
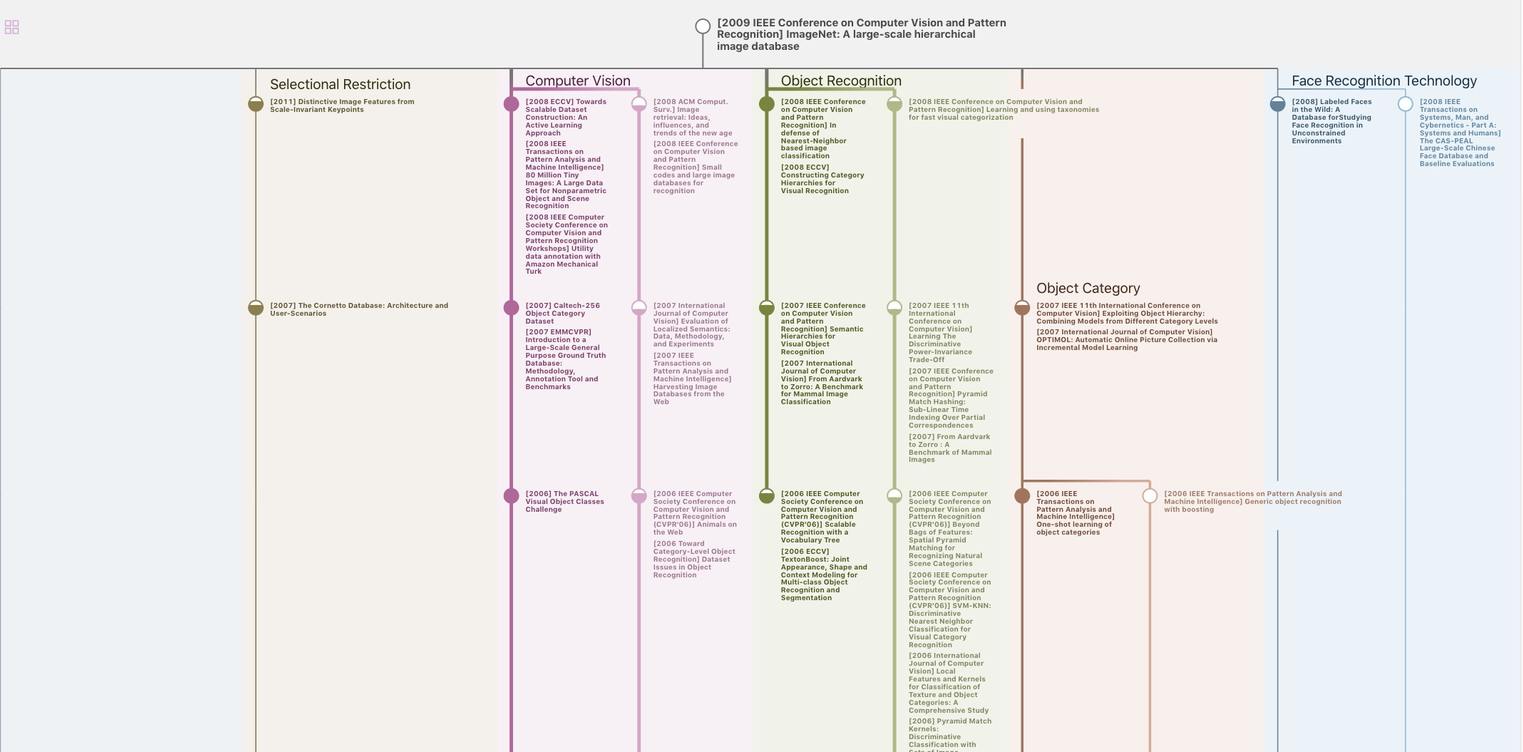
Generate MRT to find the research sequence of this paper
Chat Paper
Summary is being generated by the instructions you defined