Boosting Semantic Segmentation from the Perspective of Explicit Class Embeddings
2023 IEEE/CVF International Conference on Computer Vision (ICCV)(2023)
摘要
Semantic segmentation is a computer vision task that associates a label with each pixel in an image. Modern approaches tend to introduce class embeddings into semantic segmentation for deeply utilizing category semantics, and regard supervised class masks as final predictions. In this paper, we explore the mechanism of class embeddings and have an insight that more explicit and meaningful class embeddings can be generated based on class masks purposely. Following this observation, we propose ECENet, a new segmentation paradigm, in which class embeddings are obtained and enhanced explicitly during interacting with multi-stage image features. Based on this, we revisit the traditional decoding process and explore inverted information flow between segmentation masks and class embeddings. Furthermore, to ensure the discriminability and informativity of features from backbone, we propose a Feature Reconstruction module, which combines intrinsic and diverse branches together to ensure the concurrence of diversity and redundancy in features. Experiments show that our ECENet outperforms its counterparts on the ADE20K dataset with much less computational cost and achieves new state-of-the-art results on PASCAL-Context dataset. The code will be released at https://gitee.com/mindspore/models and https://github.com/Carol-lyh/ECENet.
更多查看译文
关键词
semantic segmentation,explicit class
AI 理解论文
溯源树
样例
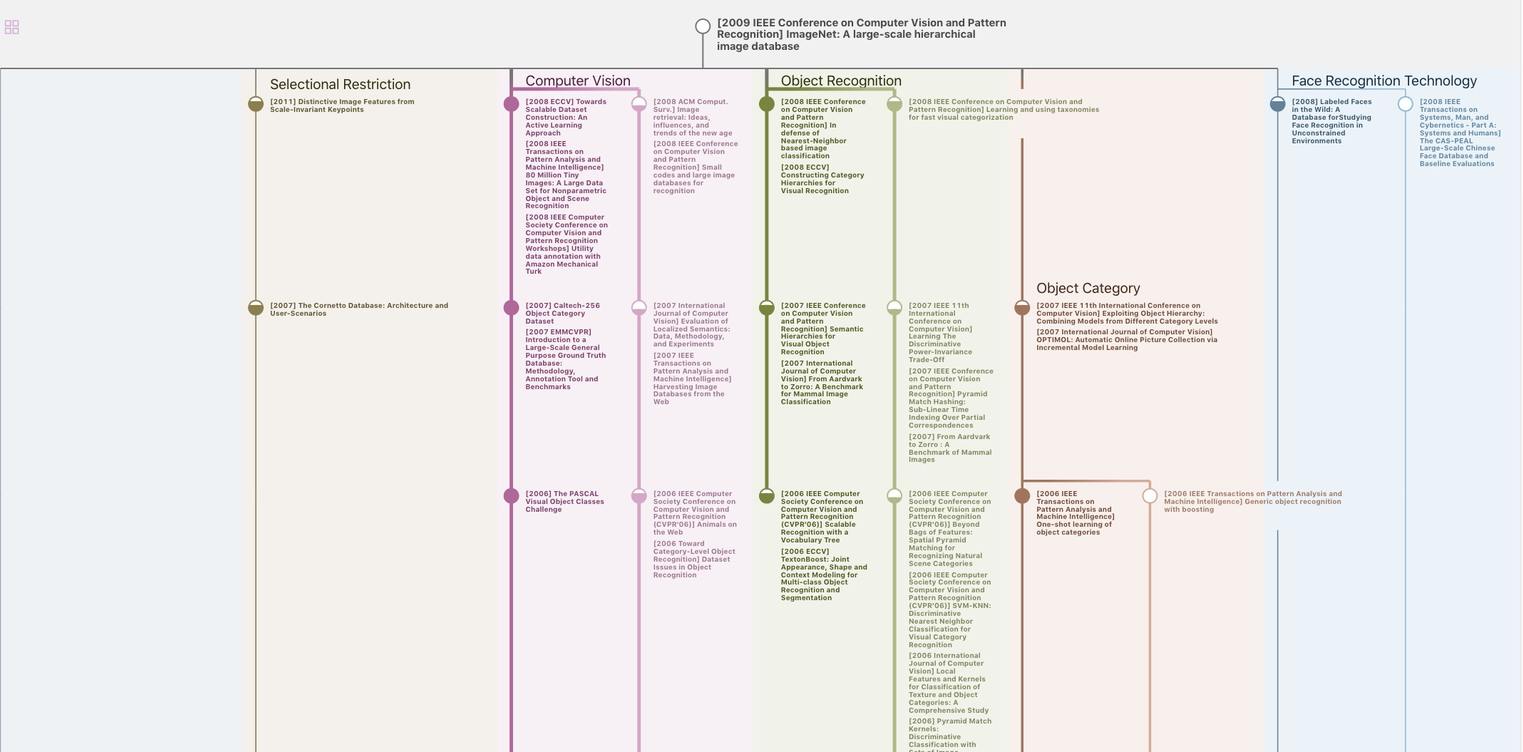
生成溯源树,研究论文发展脉络
Chat Paper
正在生成论文摘要