SCP: Spherical-Coordinate-Based Learned Point Cloud Compression
AAAI 2024(2024)
摘要
In recent years, the task of learned point cloud compression has gained prominence. An important type of point cloud, LiDAR point cloud, is generated by spinning LiDAR on vehicles. This process results in numerous circular shapes and azimuthal angle invariance features within the point clouds. However, these two features have been largely overlooked by previous methodologies. In this paper, we introduce a model-agnostic method called Spherical-Coordinate-based learned Point cloud compression (SCP), designed to fully leverage the features of circular shapes and azimuthal angle invariance. Additionally, we propose a multi-level Octree for SCP to mitigate the reconstruction error for distant areas within the Spherical-coordinate-based Octree. SCP exhibits excellent universality, making it applicable to various learned point cloud compression techniques. Experimental results demonstrate that SCP surpasses previous state-of-the-art methods by up to 29.14% in point-to-point PSNR BD-Rate.
更多查看译文
关键词
CV: 3D Computer Vision,CV: Applications,CV: Bias, Fairness & Privacy,CV: Other Foundations of Computer Vision
AI 理解论文
溯源树
样例
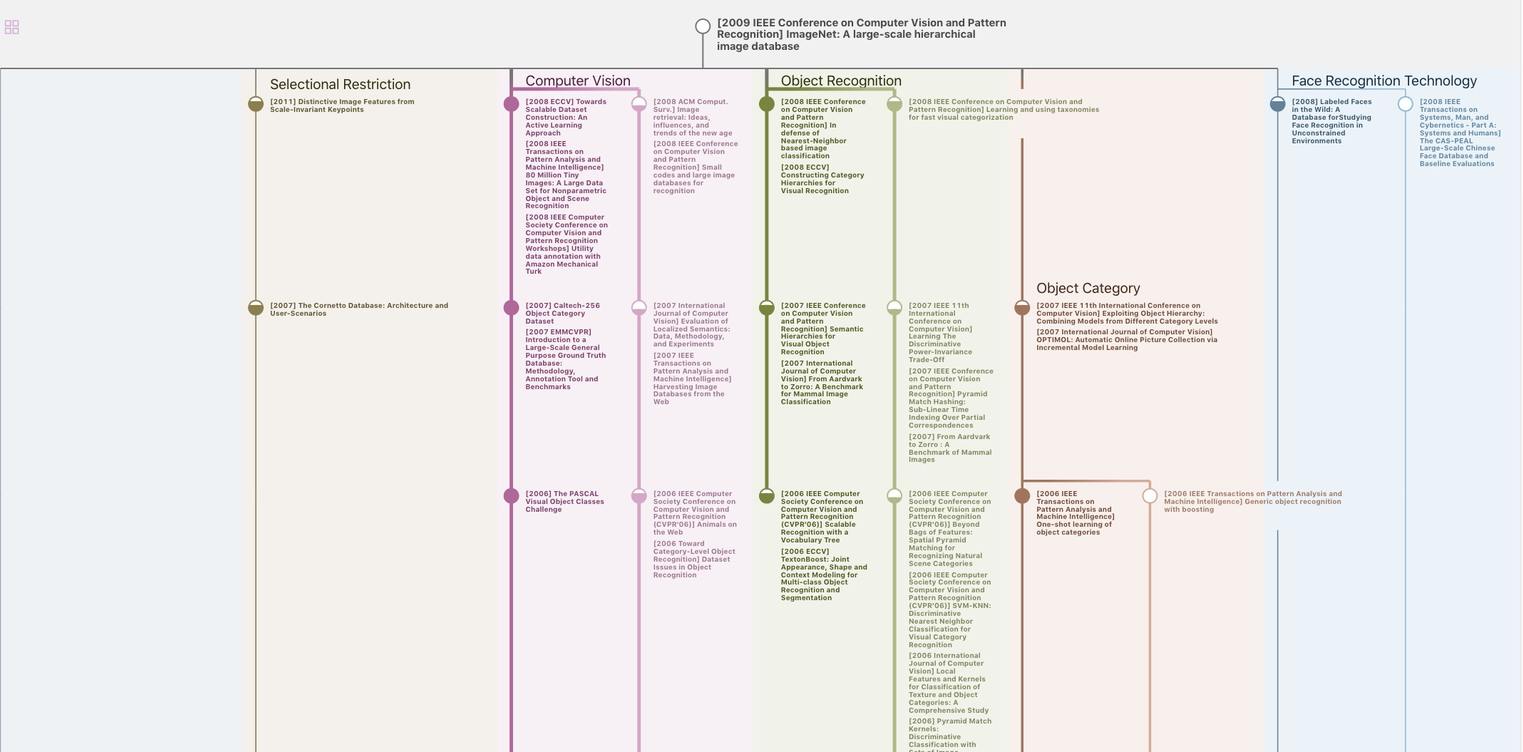
生成溯源树,研究论文发展脉络
Chat Paper
正在生成论文摘要