Open-Set Face Recognition with Neural Ensemble, Maximal Entropy Loss and Feature Augmentation
CoRR(2023)
摘要
Open-set face recognition is a scenario in which biometric systems have incomplete knowledge of all existing subjects. This arduous requirement must dismiss irrelevant faces and focus on subjects of interest only. For this reason, this work introduces a novel method that associates an ensemble of compact neural networks with data augmentation at the feature level and an entropy-based cost function. Deep neural networks pre-trained on large face datasets serve as the preliminary feature extraction module. The neural adapter ensemble consists of binary models trained on original feature representations along with negative synthetic mix-up embeddings, which are adequately handled by the designed open-set loss since they do not belong to any known identity. We carry out experiments on well-known LFW and IJB-C datasets where results show that the approach is capable of boosting closed and open-set identification accuracy.
更多查看译文
AI 理解论文
溯源树
样例
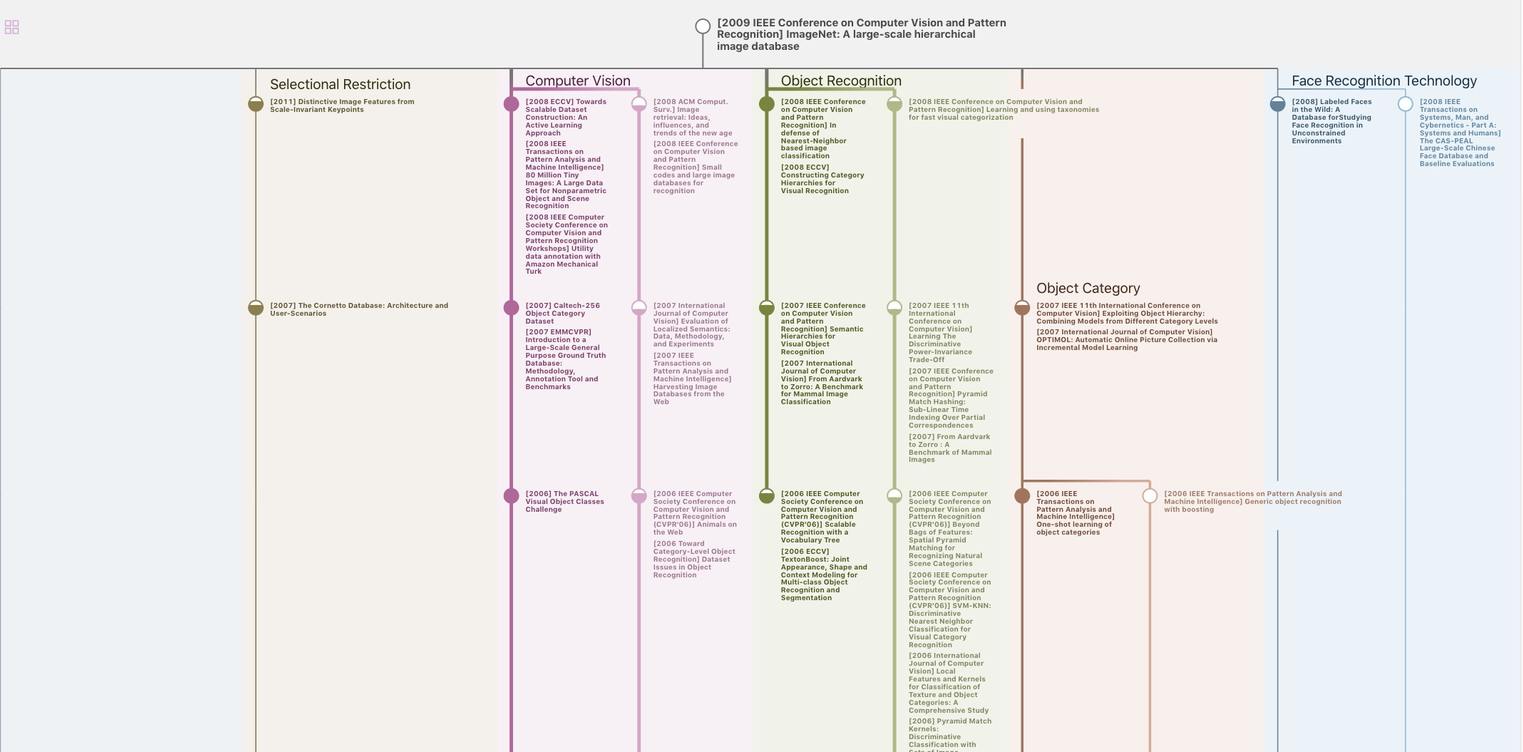
生成溯源树,研究论文发展脉络
Chat Paper
正在生成论文摘要