Visual transformer with stable prior and patch-level attention for single image dehazing.
Neurocomputing(2023)
摘要
Single-image dehazing aims to recover blurred image details and improve image quality, which is a challenging ill-posed problem due to severe information degradation. In the image dehazing task, extracting local features from adjacent regions is particularly important. However, Transformer-based methods lack relative awareness of patch-level features. Furthermore, due to the sensitivity of self-attention to textcolorreddata distribution, the model suffers severe performance degradation when migrating from synthetic domain to real domain. To alleviate the above problems, we propose visual transformer with stable prior and patch-level attention (VSPPA) for image dehazing. Firstly, we propose a region-aware patch-level attention module to obtain the positional correlation between local patches and contexts, which can enhance the concentration of local patch-related features. Next, due to the instability problem caused by distribution shifts, we introduce dataset-independent prior to guide the transformer model, thereby preventing feature drift thus to improve the robustness of the model. Finally, domain-drift leads to insufficient dehazing when the model trained on synthetic data while migrates to the real environment, we come up with a introduce a patch filling strategy (PFS) for fuzzy data to narrow the domain gap and realize the generalization in real scenes. Extensive experiments show that the model achieves State-of-the Art on the SOTS synthetic dataset and effective generalization to real-world scenarios.& COPY; 2023 Elsevier B.V. All rights reserved.
更多查看译文
关键词
visual transformer,attention,patch-level
AI 理解论文
溯源树
样例
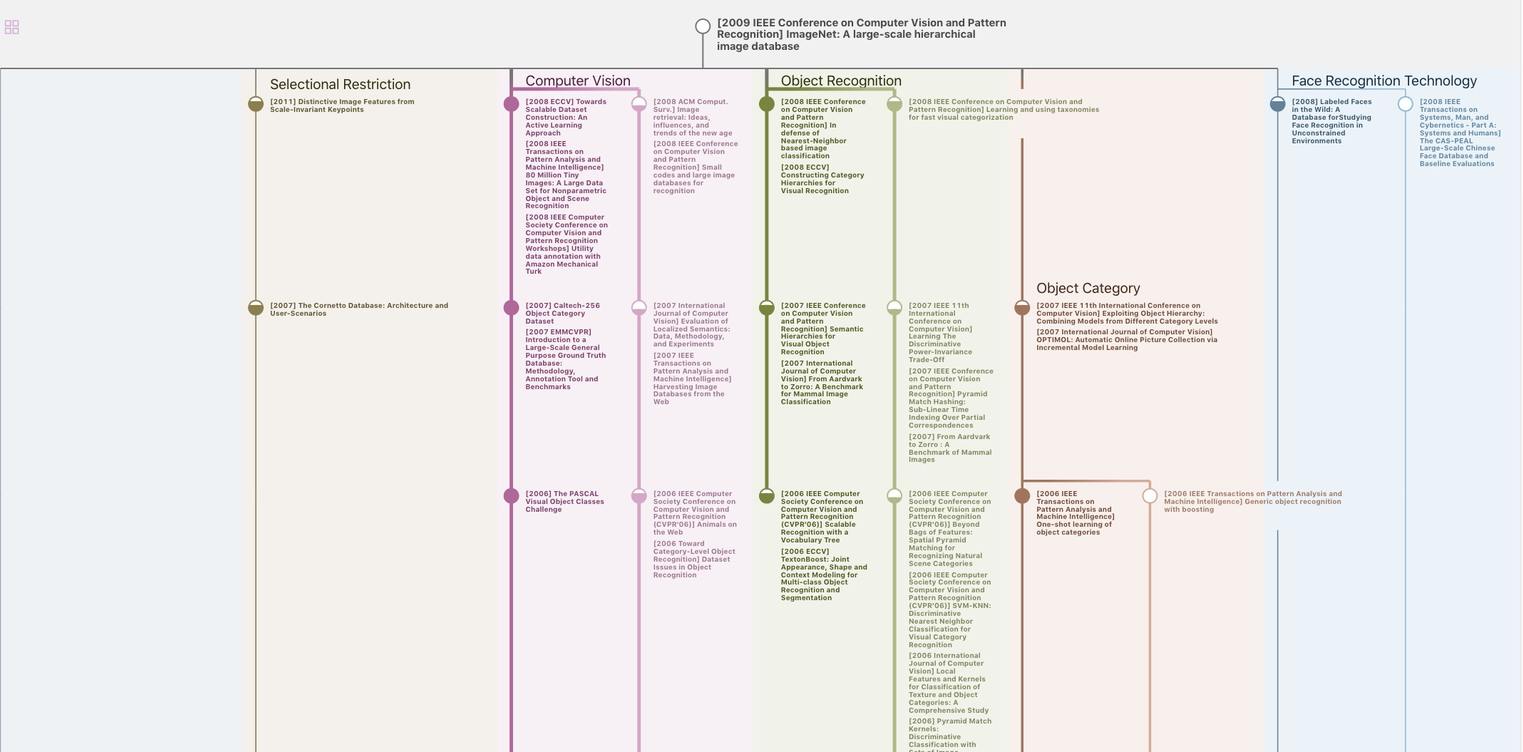
生成溯源树,研究论文发展脉络
Chat Paper
正在生成论文摘要